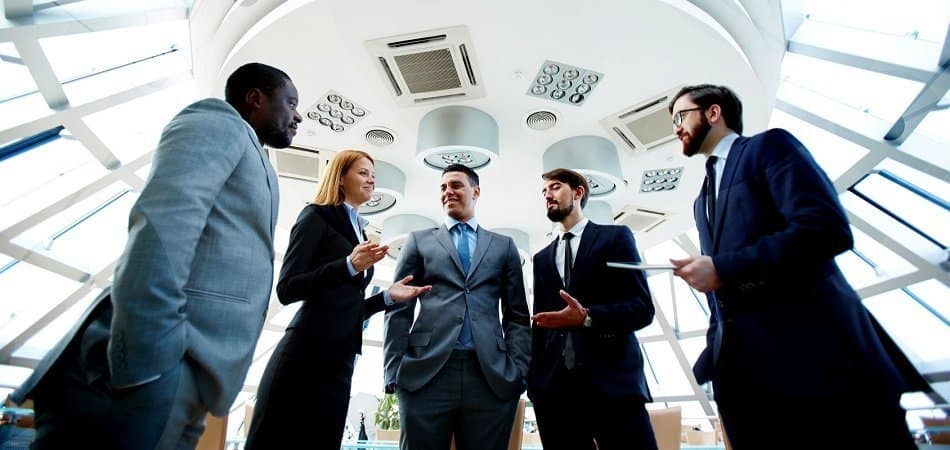
Episode Summary: Whether we’re talking about customer service, marketing, or building developer teams, what we try to do on our AI in Industry podcast is bring to bear lessons that are transferable. There are few more transferrable ideas than what makes a company ready to adopt AI. When it comes to the willingness and the ability to integrate AI into a company strategy and to fruitfully adopt the technology to really see an ROI, what do the companies that do so successfully have in common? What do the companies that are not ready or too fearful to do it have in common?
There are probably few companies in the AI vendor space that are aiming to sell AI more ardently into the enterprise than Salesforce, and there are few people that know more about how that process is going than Allison Witherspoon, Senior Director of Product Marketing for Salesforce Einstein, which is their artificial intelligence layer on top of the Salesforce product.
We speak to Witherspoon about the telltale signs of a company that understands the use cases of AI in their industry and that have a good chance of driving value with AI. We also talk about the common qualities of companies that might not ready for I adoption.
We hope this interview allows executives to get a better sense of whether or not their own companies are ready for AI.
Subscribe to our AI in Industry Podcast with your favorite podcast service:
Guest: Allison Witherspoon, Senior Director of Product Marketing, Salesforce Einstein at Salesforce – Salesforce Einstein
Expertise: marketing, AI adoption in the enterprise
Brief Recognition: Prior to Salesforce, she was the Marketing Manager for the North American division of Enecsys and a corporate communications specialist at TIBCO Software.
Interview Highlights
(03:30) Where do you see fear coming from the C-suite [with regards to AI adoption]?
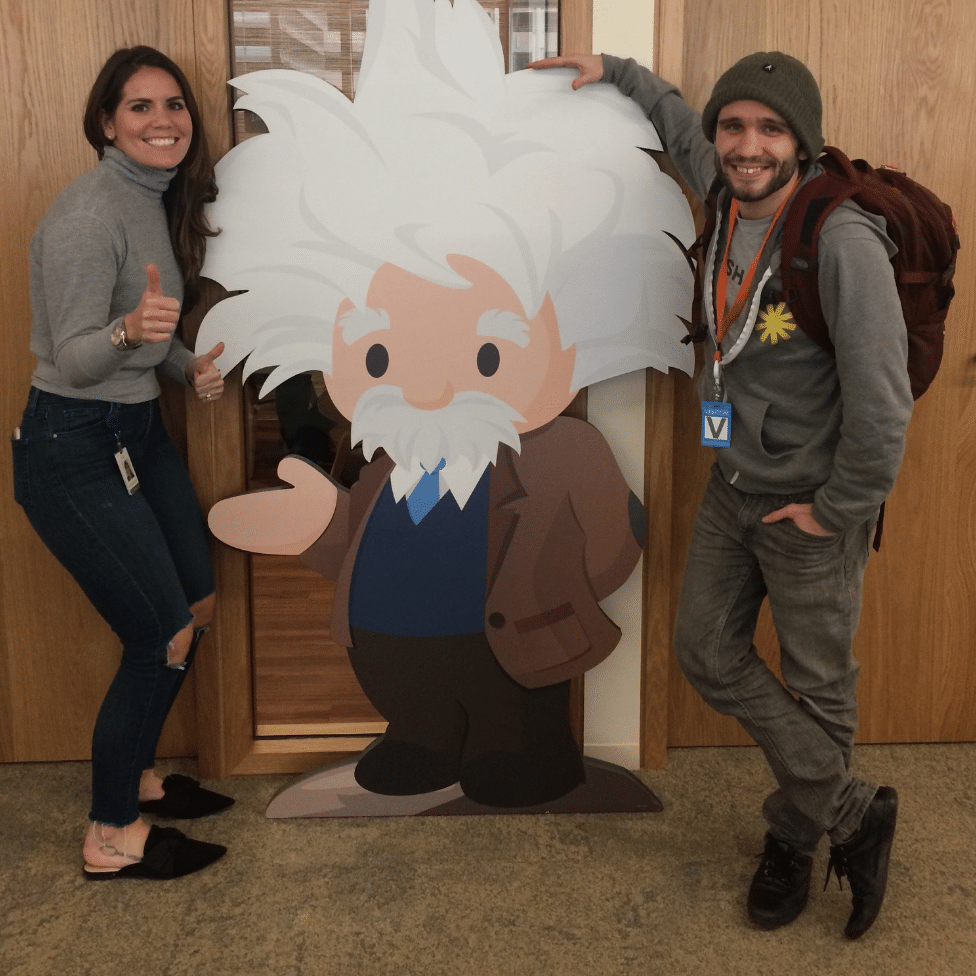
So the good news is, I think we’ve seen a bit of a pendulum swing in the past three to five years where–I call it the “AI fear hurdle”–I think we’ve seen that start to lower a little bit, and I think as I talk to more and more customers and executives, people are warming up to AI. It’s taken a bit longer, obviously, definitely a bit longer for the enterprise and the consumer world, but we are starting to see more and more, especially astute companies, leading companies, understand the fact that they need to have an AI strategy in place.
That being said, I kind of bucket them into four main categories of the fear hurdles that we see in artificial intelligence. The first I think is just defining the problem, or what we consider to be the use case for AI. I think a lot of companies just don’t even know where to get started, and so they just don’t get started because they don’t know the right problems for machine learning to solve.
I always like to say start with one and then prove out the value and move on. So we talk to a lot of companies who maybe have a list 20 items long of use cases that they see for AI. Everything from predicting churn to predicting deal conversion, all of these different things that they want to do, all these different kinds of models they want to build, and I just say, “start with the low hanging fruit. Start with one, prove out the value to your business users who are actually going to be interacting with whatever the output of that model is, the prediction that this model makes, and then move on from there.” So I think helping folks to prioritize that list of 20, and then helping them kind of figure out what the low hanging fruit is that might be good for an automated tool, versus where they need their data scientists to focus.
We call it solution mapping here, and as a vendor, we’re more than that. We’re a strategic partner, and so that means that we have to help our customers look through that long list of 20 use cases, help them solution map, help them figure out where our technology can fit in, and help them quite honestly figure out which use cases are better left for data scientists. So that’s an exercise that we do with our customers all the time, and I think it’s really necessary.
Yeah. It’s funny because of, you know, in the startup world … You guys are a huge company and have been around for quite a long time, and in the startup world, I think people also have to end up doing this because whether you’re selling into … For example, a lot of our audience is banking in pharma. You’re not walking into a room of folks that are already going to have, click, all the concepts and use cases, so they have to do the same mapping but they don’t have the same team and budget. So for them, it’s kind of annoying. It’s like damn, we want the market share, we want the lock-in. It’d be great to just have a product that could sell, but we know we got to do this dance first-
I think back to the biggest lessons we’ve learned over the past two years since Einstein’s been in the market. You will never win a conversation with a customer if you go in and you pitch AI or you pitch machine learning or you pitch natural language processing or you pitch deep learning, especially to C-suite, especially to executives. They don’t care. They have acute business problems that they want their vendors to help them solve. Things like churn, things like deal scoring, all of those kinds of things. If you start with a business problem, if you start with the pain, and you speak to them in human language, and you don’t lead with the tech, you will win them over.
So that’s kind of where I see everyone’s journey with AI starting, really defining those use cases. Then once you have your use cases in place, it’s all about the data, and this is the number one most common challenge that we hear from our customers is, “okay, we think we know, we think we have this kind of AI project that we’re ready to set out on, but we don’t know the data that we need. It might live in multiple sources. We don’t know how to bring it all together. We don’t know how to clean it and scrub it and make sure it’s not biased. We don’t know if we have enough of it to even make machine learning valuable, so all of these questions around data.”
(08:45) Is it going to be years until we get [to where AI is as understood as the Internet], or do we never get there with the C-suite?
Yeah. I don’t know. I think it’s going to require a bit of handholding in the short term. I definitely think you have to … And this was kind of my hurdle three and four, which I tend to lump together, is really around trust and change management, because I think they kind of are part and parcel.
So with change management, we think about the business users whose routines will be affected by the AI. So if, let’s just take an example like lead scoring, for example. If we turn on machine learning and we’re scoring all of the leads for a company and telling them which ones are most likely and least likely to convert, that sales rep has to start trusting a machine now, versus in the past maybe they were scoring by intuition or maybe they had a different way that they were calling down on their leads every day.
So they have a historic way of doing things. We’re asking them to change it. We’re asking them to trust a number that’s all of a sudden being served up to them. That requires some change management and some trust. And so it’s about building things into the tooling, into the process, into the modeling where, one, we’re exposing why things are scored the way they scored so that that sales rep starts to trust that number.
So we like to say like AI is not just a black box. We’re showing you the underlying factors that contribute to that score. So that’s one piece, and then the second piece is giving them the autonomy to kind of act on that information and also influence it. So one, nothing’s ever being done automatically without their oversight, but then two, how they interact with that score automatically then goes back and feeds into the model. So the model is constantly learning from how a sales rep interacts with that lead score.
(11:30) Do you find that transparency is the critical kind of mode for overcoming that in some sense? Is it kind of bringing the subject matter experts who maybe aren’t the techies into the conversation about how we build it? What gets people to get that buy in cross-functionally?
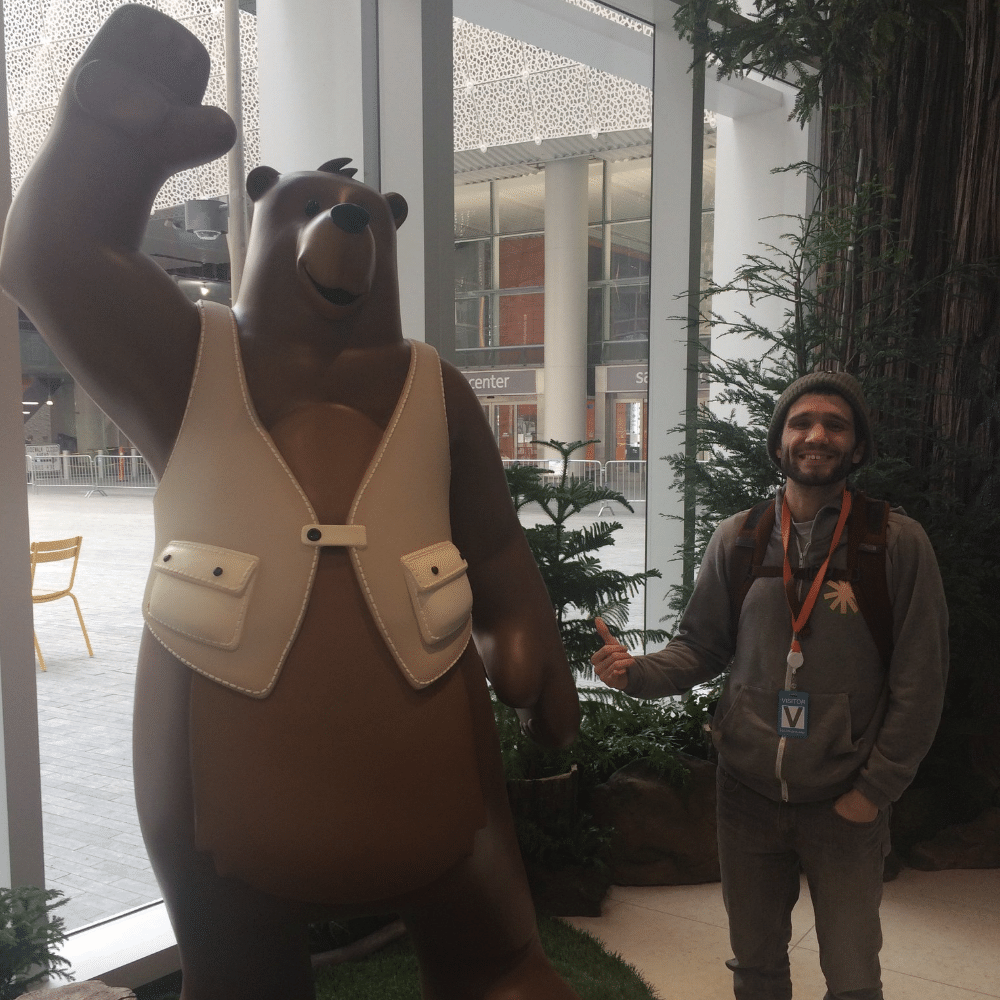
I think there’s a couple things. One is I think you have to settle on a KPI and prove out the ROI before you turn the AI on. So let’s take lead scoring for example. The way that you measure if a lead is converting and whether your leads are converting effectively is lead lift. Are you converting more leads? And so if we can prove out to a set of sales reps, hey, if we turn on AI, you’re going to see a 3X increase in your lead conversion, then they kind of sit up on the edge of their seat. And so I think if you can start doing that ROI modeling and prove that out before you even turn on the features, before you even invest in the projects, whatever it might be, you start getting their buy in because they see how their life will improve. Whether it’s a productivity gain, time saved, deal conversion, win rates, whatever that metric is that you settle on, prove it out ahead of time.
But then back to this idea too of kind of black box and transparency, with every single prediction that Einstein makes for Salesforce, for example, we ship what’s called predictive factors. So for lead scoring, for example, you wouldn’t just see a 95 or a 72. Next to that number, you would see why it’s being scored the way it is, the top five negative and the top five positive correlating factors. So a positive contributing factor could be ZIP code or the lead source. And so those kinds of triggers and that kind of information also helps with the transparency.
(13:45) How do we minimize sort of the frustration factor there? Because you could see if the beta version went out to all the sales folks, they might be like, “I’m never using this.” Is there a small cohort that needs to get that training down pat? How does this work?
So we have actually some customers who will turn this feature on and do an AB test. So they’ll turn it on for maybe half their reps and then let the other half continue with whatever method they’re doing, maybe intuition based, and they do kind of an internal bake-off, once again, back to this idea of proving out the value ahead of time. If we can show in this kind of AB tests that the model works better than intuition, we can get the buy in from that second cohort. So that’s one example. I think, yeah, it’s just tricky.
It requires trust, back to the whole change management piece too. I think the more that we can do to make it easy for the C-suite to consume this information without ever having to expose a … Like, you get into rooms and they’re like, well … Maybe you have a data scientist in the room who wants to see the model, but nine times out of 10 you’re going to be talking to people who don’t care.
They don’t want to know how the prediction’s being made or what data is being fed in. They just want back to this idea of some business pain solved for them.
In addition to the kind of the bake-off idea, the AB testing, is models should constantly be refining and getting better, and that’s one thing that we at Salesforce believe in, is that our models are constantly being rerun, reshipped to customers, based on all of that indirect and direct feedback. So direct feedback could be as simple as a thumbs up, thumbs down arrow in a chatbot conversation.
Was this conversation helpful? It was helpful. Great. The model will learn from that. It was a good conversation. Versus kind of indirect feedback, back to the lead scoring example, maybe Salesforce or Einstein scores a lead at 95. It doesn’t end up converting. Okay. That was a pretty crummy lead score. The model will learn from that. So this idea that it’s not just you ship it once and it’s done, but that it’s constantly tuning, it’s constantly learning as you go.
(16:00) I imagine a lot of the time selling into the enterprise, it’s going to be the vendor that’s kind of staying on top of the model and kind of, I don’t want to say consultative or coaching in some sense, but that is there in a technical way to kind of see that iteration continue to mature. Is that sort of how it works generally on the Einstein side?
Yeah, so a lot of the Einstein features at Salesforce in particular are what we call like admin enabled, so a Salesforce admin is going to go into setup. They’re going to turn them on, they’re going to be the ones configuring them, they’re going to be the ones monitoring them. So we also ship model metrics with our models, so you can actually see the strength of the prediction, the strength of the model, and you can see that trend over time, and so-
So we don’t have to monitor that for our customers. We give them the tools, we give the admins, the super users, the tools to monitor it themselves, and hopefully they see that trend line going up into the right as that model is constantly learning and tuning and getting better.
We get a lot of customers who come in and they say, “Well, it’s great that Einstein can do a lot of this kind, these CRM specific use cases, but I also have a team of data scientists internally who have already built a churn model, who have already built a lead scoring model,” and I say, “That’s fine.” Like Einstein, any AI system can coexist with an internal team of data scientists. It’s back to that use case list. What are your 20 use cases? What can machine learning from Einstein just chop off, versus what do you need your experts who know your business, who know your data, who can focus on more complex use cases that are specific to your company? Save that for your data scientists.
(18:30) What feels like low hanging fruit? What do those teams have in common where we feel like maybe they’re ready to make this stuff work? Is it having the data ready, is it the talent?
It kind of goes back to those four things and the inverse of them. They know the use cases. They have their data in one place, in a structured format, because in order to do machine learning. Your data has to be structured, and that’s the beautiful thing about Salesforce. All of our data sits on fields, on records, and objects. So it’s that beautifully structured data we can already start learning from. So they come in with a very clear idea of their use cases, like I did a customer meeting a few weeks ago, and the customer came in and said, “We know we need chatbots for service. We know we need some next best action workflow engine.” Like they came in and they already knew exactly the problems that they needed to solve, so it was a very easy conversation to have with them.
So they’ve already brought in the departments that are going to be affected by AI. For chatbots, maybe it’s customer service. For email scoring, it’s your marketers, whatever it might be. They have that business group in the room who is experiencing the pain because it’s not IT that’s experiencing the pain. It’s that marketer, it’s that sales rep, it’s that service agent, so that person is in the room, the person who’s going to be using the features, consuming the AI, and the IT decision maker, and you have alignment between those two constituent groups.
(20:15) Those execs, they understand the use cases. They have their data in order. Where did they learn this stuff, or are they just born and bred in San Francisco and those are the guys that can do it? Do they go to a lot of events? Are they googling a bunch?
I don’t know if there’s kind of one answer, one silver bullet. I think it’s probably the same folks who invested in mobile apps and invested in social back in the day and invested in the cloud first.
I think the important thing is it doesn’t just have to be the big enterprises, and that’s really kind of our mission at Salesforce is democratizing access to this type of technology because I think in the past there’s this misconception that, oh, well, in order to do AI, like I’m never going to be able to do AI. I can’t hire data scientists. They’re the most expensive people to hire these days, and they’re too hard to come by. Like I guess I’ll just never scratch the surface of that. And so it’s about, how do you ship features and functionality that any size company, whether you’re a six-person nonprofit or multi-hundreds of thousands big corporation, you can leverage the technology and leverage the insights.
Subscribe to our AI in Industry Podcast with your favorite podcast service:
Header Image Credit: Gravity Payments