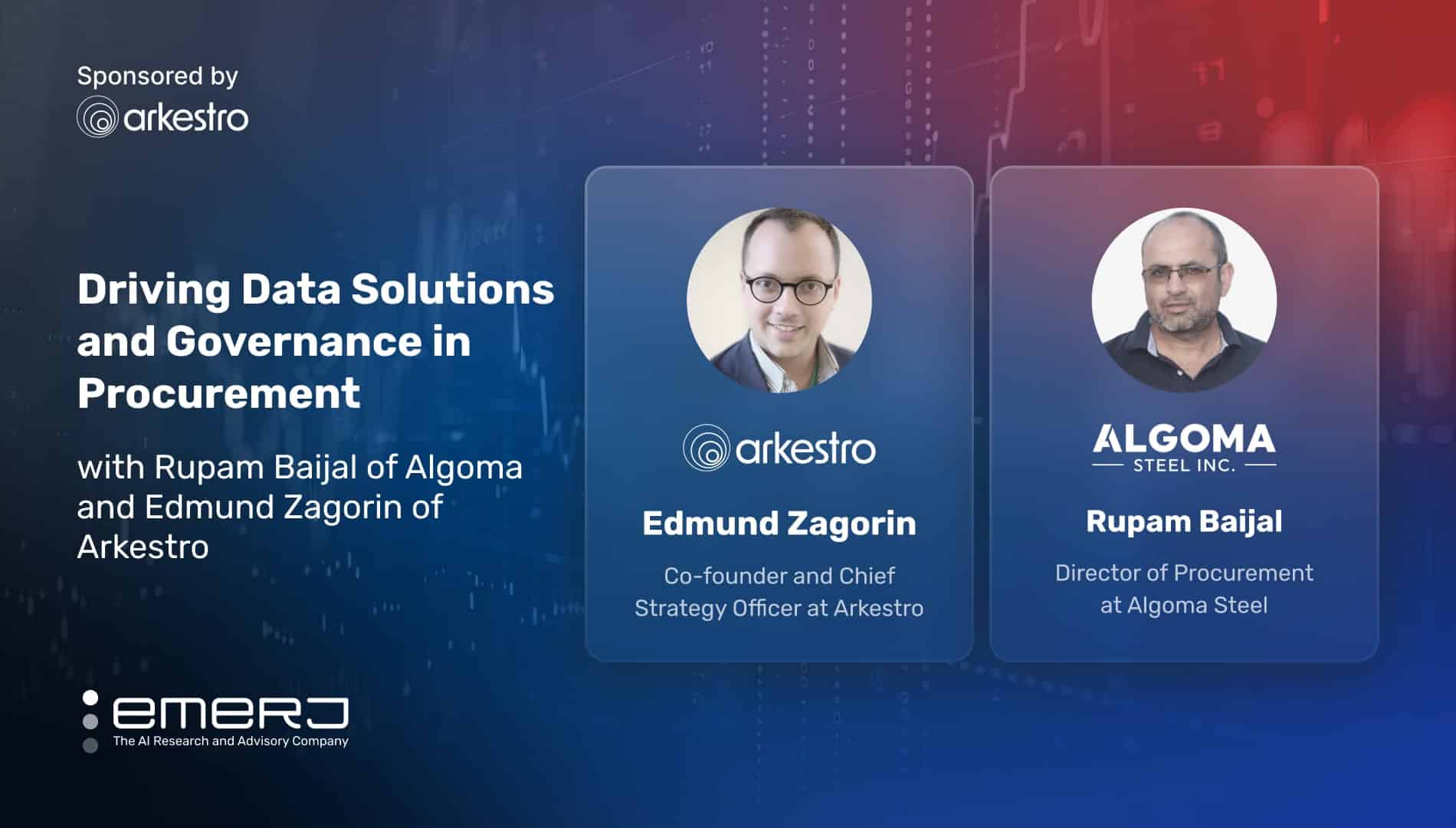
This interview analysis is sponsored by Arkestro and was written, edited, and published in alignment with our Emerj sponsored content guidelines. Learn more about our thought leadership and content creation services on our Emerj Media Services page.
Supply chain leaders are finding data tools that – even when equipped with basic approaches to collection, governance, and analysis – yield tremendous value, particularly in commodities and raw materials spaces. Further market research is quick to mention that some centralization of these data and processes is essential, no matter the size or shape of the organization. Raw materials players, in particular, must be cautious about having an approach tethered to the experiences of true subject matter experts.
In the rapidly evolving landscape of AI, the role of the Chief Procurement Officer (CPO) is undergoing a significant transformation along the way. As industry conferences shift their focus from theoretical discussions to practical applications, with examples making significant noise in the market, like Toyota’s “AI transformation” of their IT service center. These initiatives include specific AI investment clauses in vendor contracts, underscoring a crucial shift from human-dependent services to AI-delivered solutions. As an industrial trend, the transition is resulting in enhanced operational efficiency and cost reductions.
Cutting-edge technologies such as document automation for statements of work, negotiation chatbots, and bringing predictive capabilities to procurement orchestration are already demonstrating substantial impacts. The new AI-driven era for procurement not only promises operational improvements but also significant financial benefits, providing CPOs with powerful tools to enhance their already significant contributions to profitability.
Emerj Senior Editor Matthew DeMello facilitated a conversation between Algoma Steel Director of Procurement Scrap & Iron Units Rupam Baijal and Arkestro Co-founder and Chief Strategy Officer Edmund Zagorin about how heavy industry leaders can best translate what they do into language that helps data scientists better understand where to drive efficiencies. Together, their discussion yielded new perspectives on driving value in procurement systems in two distinct areas we will examine:
- Overcoming data challenges: Standardizing data between enterprise divisions and partner organizations to prevent breakdowns in the procurement process, particularly in scenarios involving mergers or acquisitions.
- AI-driven vendor performance monitoring: Leveraging AI to monitor vendor performance metrics, emphasizing the significance of tracking delivery times and addressing delays promptly to ensure on-time delivery and maintain trust in the supply chain.
- Measuring productivity: Measuring productivity metrics such as spend, number of suppliers, and cycles per headcount, emphasizing the need for standardized yet adaptable measurement processes to evaluate the effectiveness of AI investments in procurement.
Guest: Rupam Baijal, Director of Procurement Scrap & Iron Units, Algoma Steel
Expertise: Vendor negotiation, Supplier relationship management
Brief Recognition: Rupam is a 30-year veteran of the commodities space who has been with Algoma since 2006. He has a Bachelors in Engineering from Motilal Nehru National Institute of Technology.
Guest: Edmund Zagorin, Founder & Chief Strategy Officer, Arkestro
Expertise: Entrepreneurship, Artificial Intelligence, Operations Management
Brief Recognition: Edmund Zagorin is the Founder & CEO of Arkestro Inc. Before founding Arkestro, he worked as a strategic sourcing advisor specializing in digital process transformation for large health systems and Fortune 1000s, with a focus on predictive pricing, process design, and data operations. He graduated from the University of Michigan with a degree in International Affairs and Public Policy.
Overcoming Data Challenges in Procurement
Edmund begins the conversation by summarizing on the broader challenges of adopting AI in the manufacturing sector, particularly in procurement. Despite a surge in interest in large language models and horizontal AI, the manufacturing industry has been notably resistant to digitization over the past decades. While there have been advances, the industry is now at a crossroads where companies are considering whether it’s possible to leapfrog from traditional, often outdated systems like EDI or email to workflows that incorporate AI agents.
In response, Rupam points to another business problem in the cleanliness and consistency of data in procurement processes, especially when dealing with a large number of line items. Rupam breaks down how, when data is clean and standardized, it becomes easier to clearly define what is needed, leading to breakdowns in the procurement process.
The issue becomes even more challenging when companies undergo mergers or acquisitions, resulting in disparate data sources. Rupam acknowledges efforts by external agencies to clean and standardize data but also acknowledges that the effectiveness of this cleansing process depends heavily on the quality of the data provided to them initially.
In turn, Edmund echoes Rupam’s point about encountering issues when dealing with a large number of line items and dirty data. He adds another layer to the problem by highlighting the challenge of “supplier fatigue,“ referring to suppliers who have been working with a company for years and are accustomed to its particular procurement process but may become worn down by its length.
Edmund also delves into the traditional approach to procurement in manufacturing, where different items are often awarded to other suppliers, unlike indirect procurement, where one supplier may be selected for multiple items. He explains how demand plans are usually split across various suppliers according to percentages, a task traditionally performed manually in Excel using pivot tables or macros:
“One of the things that, I think, has become really interesting is when the supplier makes a mistake in the quote. Maybe the original part number is wrong, or maybe the supplier just mistakenly keys something wrong or copied and pasted some data that’s not right. Now, you have the beginning of what will become a data challenge of the future. All of these inputs graduate through the different systems that they move through, and then the data where you’ve done what Rupam has described – you’ve hired someone to clean it – all of a sudden is getting dirty again, and it’s getting challenged for that. So, we tried to take a different approach. We said, ‘What if there’s a way to make data anti-fragile, in some sense, where every process that you include cleans the data and makes it cleaner and cleaner as you go?'”
–Edmund Zagorin, Founder & Chief Strategy Officer at Arkestro
Of this partial self-cleaning approach, Edmund shares an example of working with Koch Industries, where they used AI to propose item IDs and components during the quoting process, allowing suppliers to validate this information and effectively using them as subject matter experts to clean the data. This approach ensures that data quality is maintained or even improved over time, eliminating the need for periodic large-scale data-cleaning projects.
AI-driven Vendor Performance Monitoring
Rupam discusses the importance of effectively managing different types of items in procurement processes. He categorizes items into three segments:
- Low-value, low cost
- Low-value, high cost
- High-value, high price
Throughout his explanation, Rupam emphasizes that, while the need for a part may arise regardless of its value, the consequences of not receiving a crucial part can lead to significant losses, especially for high-value items.
He proposes this area in particular is ripe for AI application to prioritize procurement decisions. Rupam illustrates this with an example where spending extra time finding the best deal for a low-value item may save a small amount of money upfront, where the delay could result in substantial losses in production if the part is unavailable when needed. The next step would be develop a program to analyze metrics related to the speed of requisition fulfillment to ensure the timely procurement of critical items.
Rupam also stresses the importance of on-time delivery from suppliers and the trust established in the supply chain. He suggests that AI can play a crucial role in monitoring and analyzing vendor performance metrics, including tracking delivery times and following up on delayed parts or purchase orders.
In response, Edmund provides more insights into different procurement strategies and organizational structures, drawing on the framework of the Kraljic Matrix to illustrate various approaches. He explains that each quadrant of the matrix corresponds to a different priority or “North Star“ for procurement activities:
- Bottleneck Quadrant: The focus is on ensuring continuity of supply to prevent production delays or interruptions. The goal is not necessarily to maximize value creation but to maintain operations smoothly.
- Strategic Quadrant: The emphasis is on building solid relationships with suppliers, mainly when there are limited options available. Companies in this category may rely on suppliers for innovation and technological advancements.
- Leverage Quadrant: This quadrant involves seeking cost reductions and cost avoidance opportunities. Procurement efforts here are geared towards negotiating favorable terms and driving down expenses.
Measuring Productivity in Procurement
Edmund also discusses the importance of the organizational structure of the procurement function within a company. He notes that the reporting lines of procurement — whether to finance, operations, or supply chain — can significantly impact decision-making processes.
He highlights a trend, accelerated by the COVID-19 pandemic, where procurement is increasingly being integrated into the operations or supply chain organization to ensure alignment with production needs and priorities.
“I just go back to this question of productivity and, as we’re thinking through the new era of procurement, especially manufacturing, I think there are some interesting metrics around productivity, which could be measured simply as amount of spend, number of suppliers, number of cycles – however it is quantified, and every company will quantify it differently. But just looking to see activities per headcount increase year over year, as there are more investments in technology and in AI specifically.”
– Edmund Zagorin, Founder & Chief Strategy Officer at Arkestro
Edmund emphasizes the interconnected nature of AI solutions, drawing parallels with user-friendly tools like DocuSign. He stresses the importance of AI solutions being seamlessly integrated into existing workflows, such as email, to ensure widespread usability and adoption.
Additionally, Edmund underscores the significance of these solutions being connected and regularly updated using both internal and external data sources. Such connectivity enables procurement and supply chain teams to leverage diverse datasets, including market and weather data to enhance decision-making and operational efficiency.
In return, Rupam expresses excitement about the potential of AI in procurement, but raises an important question regarding the role of human judgment and risk-taking abilities in the procurement process. He acknowledges that AI can handle many procurement decisions efficiently. Still, he talks about envisioning how AI will be able to replicate the nuanced decision-making and risk-assessment abilities of human procurement professionals is a hurdle for many leaders today:
“It’s about the risk-taking ability also, where you walk into a negotiation, or you’re buying a commodity, or you’re doing all the fancy stuff that really hits the company’s bottom line. How will AI be able to refer and to mimic that? To me, or the person sitting beside me who is the same caliber, has the same education, and the same experience: While we might make different decisions on different instances, that’s where I would like to investigate more and understand how AI can react to that.”
–Rupam Baijal, Director of Procurement Scrap & Iron Units at Algoma Steel
Rupam suggests that while AI may excel in handling transactional tasks and following preset parameters, it may need help in areas where human judgment and adaptability are crucial, such as navigating complex negotiations and making other vital strategic decisions.