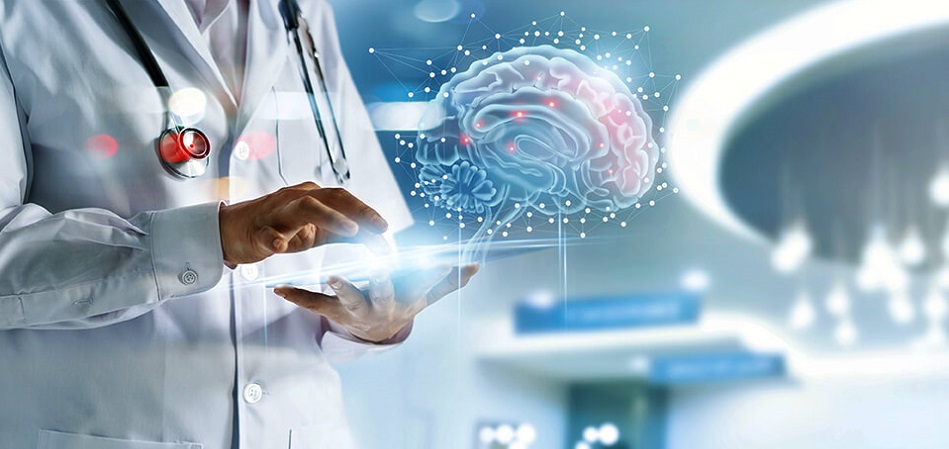
This article was written by Sergii Gorpynich, co-Founder and CTO at Star, co-written by Perry Simpson, Managing Director of Star, and was written, edited and published in alignment with our transparent Emerj sponsored content guidelines. Learn more about reaching our AI-focused executive audience on our Emerj advertising page.
While it’s easy to find healthcare AI use-cases online, it isn’t clear which applications are viable, and which are hype. Healthcare leadership needs to consider the specific data assets, resources, and needs of their organizations before deciding on an AI initiative.
In this article, we go into more detail about how executives need to adapt to the changes that AI might bring in businesses.
For more on adopting AI in the healthcare industry, download the Emerj-Star white paper, Executive Guide to AI Adoption in Healthcare.
How Healthcare Executives Adapt To AI
Without a basic grounding in what AI can do and how it works, it can be impossible for executives to find and assess AI opportunity areas.
What Executives Have to Know
What AI Can Do
Healthcare leaders should understand the basics of how technologies behind AI work, key concepts of machine learning and data science in general, the critical role of data, the importance of data infrastructure, and the kinds of problems that AI can and cannot solve.
Basic Use-Cases
Some familiarity with basic AI use-cases in healthcare can be a very helpful start. Knowing the precedents of computer vision for diagnostics, NLP for workflow management, chatbots for customer care automation, and other use-cases can help leaders look at their enterprises through a lens of AI opportunity, finding low-hanging fruit that has already proven valuable at other healthcare organizations or at businesses within different domains.
Setting Expectations for AI Projects
AI is not IT. Executives who expect to “plug in” a machine learning algorithm to improve their processes are in for a rude awakening. AI initiatives involve determining hypotheses, organizing data, training algorithms, and testing the viability of ideas iteratively and rapidly.
Many leaders already have some understanding of this difference, and many healthcare leaders have a sense of the importance of doing something with the data they’re already sitting on. Most of them acknowledge the importance of the data. They know that there is value there. They already feel urgency to exploit that value.
Some degree of urgency can be a good start, but it won’t prepare leaders for the iteration, testing, and experimentation required to bring an AI project to life. Leaders should also understand the critical importance of combining data science and subject-matter expert teams together not just when brainstorming an AI initiative, but also during the initial pilot and proof of concept.
With an understanding of team dynamics, data requirements, and necessary iteration/experimentation time, executives can more confidently choose AI projects, and data science teams are more likely to keep that executive buy-in throughout the project.
Overcoming Initial Data Hurdles
Most AI projects require historical datasets that can be used to train algorithms in a machine-readable format. The challenge in acquiring this data is that although many businesses might have existing historical data, a lot of that data might not be in a format that is accessible or useful for machine learning projects.
For instance, in the healthcare sector, Electronic Medical Records (EMR) and Electronic Health Records (EHR) are now being used to train AI algorithms, and AI vendors offer solutions to hospitals and clinics that use this type of patient data in various use-cases (from diagnostics, to billing, and beyond). Although hospitals were mandated to store these records, they were usually not stored with the intention of leveraging them to train algorithms.
What this means for management teams at large organizations, including hospitals and healthcare providers, is that data competency needs to be a grassroots drive where a business’ whole data infrastructure is tailored towards feeding self-learning algorithms. This is a change in mindset among executives in an organization, a change to a more data-centric approach. This kind of data overhaul typically doesn’t need to take place until an AI application has proven its value. We discussed the 3-phase approach to getting started with AI in healthcare in a previous article.
Understanding Time-to-Value
It is often unreasonable for executive teams to expect an immediate financial ROI on an initial AI project. Iteration time, altering data infrastructure, and adjusting team dynamics mean that AI is a true investment in learning and technology and not quick bolt-on improvement.
By viewing AI as a critical capability, as a new and important skill set, executives can frame the “ROI” of an AI project in broader and more accurate terms than by measuring short-term financial returns as well. While a company should enter a project with a clear end in mind and a way to measure the success of an AI application, they should see AI as a learning experience that will yield a competitive advantage in the years ahead.
Phases Involved in AI Upskilling
We believe that managers in businesses might be more interested in use-cases related to their specific function, as opposed to the business at large. Most managerial level employees today seem to view AI as a useful additional technology to know about, rather than looking at it as an upskilling of knowledge. This is what we have termed as the Emergence phase of AI.
In the last couple of years at Emerj, we have seen a slew of AI conferences and events that are being sought after by business executives to gain contextual insights into the fundamentals of AI. This might be a necessary transition period where business executives familiarize themselves with what AI is and what it can do with the eventual aim of knowing what the correct AI strategies might be or which AI vendors to work with.
It is likely that in the future we will find a large number of business executives who have a strong general understanding of AI use-cases and what capabilities can be gained through the technology. We call this the Adoption phase.
In the next two to three years we believe business leaders should gain the following level of understanding about AI:
- What AI applications are hyped and which types of adoption metrics and returns from AI products claimed by vendors seem reasonable. Collaboration with the right consultants and AI vendors can help speed up this process.
- The organizations existing data topography and identifying which use-cases are best suited to the company’s business goals.
- How to develop and maintain a robust data infrastructure that is scalable, and suitable for the business’s needs.
- Being able to communicate about conceptual level data science ideas across the organization, with technical and non-technical staff being familiar with fundamental terminology and use-cases.
In the third phase, what we call the Dispersion phase, we expect business leaders to have a firm grasp on general AI concepts with a more nuanced understanding of how to apply the capabilities gained through AI to solve real business problems, along with some positive returns usually in the form of cost, time or human effort reduction.
In order to better understand this phase we use the analogy of the internet. Similar to how “understanding” of what capabilities are offered by the internet is almost unanimously commonplace today, future business sectors. Most healthcare businesses are in the Emergence phase today, and those that move quickly on upskilling their teams technically (data scientists) and in terms of conceptual grasp of AI and the importance of data (business leadership) will be able to move more quickly into AI adoption.
Two Ways to Find an AI Opportunity
Sometimes, business leaders might be unsure of where to start with handling data and identifying where AI can be effectively applied to solve a business outcome.
In other cases, businesses might be confident in their areas of focus, but uncertain as to where or how artificial intelligence might add value to their process. There are two potential points of origin for integrating AI and data science into a business initiative.
Begin with a Business Outcome
In some cases, businesses might already be engaged in projects where collecting consumer data might be a part of the workflow.
Business executives who understand what capabilities are gained through AI can spot these potential data ‘mines’ and try to identify how that data can be leveraged using AI.
Lets say a company is deploying smart injection devices, which are connected to a mobile app allowing for more efficient and reliable in-home injections for patients. Such as project will likely involve collection of patient data through injection device and connected mobile app into the cloud data storage. If a healthcare firm already has this project running before even considering AI, they are collecting valuable data that could potentially be used in AI projects such as predicting patient outcomes.
We might imagine another example involving medical billing. A hospital chain may implement a new software to track payments, follow up with patients about billing issues. The hospital may decide that the data tracked in this system could be used to better forecast collections or that the data gleaned from patient messaging might be used to customize billing-related messaging (and timing of that messaging) in order to improve overall collection rates.
These are representative use-cases, but hundreds of other examples might be brainstormed, relating to almost any existing healthcare tech or data initiative. In this way, existing business objectives might become inroads for building AI capability within the firm by finding the areas where AI is the best tool for the job.
Begin with Data Assets
While existing business initiatives might be a good place to start for finding AI opportunity, it’s also possible to begin with the data itself. Healthcare leaders are often aware of the value of the data they’ve collected but are unable to determine exactly how it might be leveraged to bring about value in their organization.
As with the process of starting with business initiatives, it’s important for business leaders to have a conceptual understanding of AI’s capabilities, and to be able to foster an interdisciplinary conversation with data scientists (internal or external) and subject-matter experts in order to develop the most fruitful ideas to extract value from existing data.
For instance, a chain of clinics might have a historical record of medical images such as orthopedic X-ray images or images of lesions and tumors. Healthcare firms can potentially leverage this data to train computer vision algorithms. For example:
- An algorithm may be able to train on orthopedic X-ray images of the knee joint (from patients of a variety of ages and activity levels) in order to better determine the future severity of a particular joint injury, or the kind of pain medication most likely to help the patient.
- An algorithm trained on various staff infection lesions may be able to help doctors determine the severity of the infection, or make more informed decisions about medication or treatment.
Opportunity might also present itself in the form of operational data. It might be possible to use data about healthcare appointment volumes to better predict demand, or to more accurately forecast staffing costs.
In Conclusion
With a clear line of sight on the company’s goals and data assets, leadership teams (and their data science/subject-matter expert task force) can develop an almost unlimited number of potentially valuable AI use-cases in an effort to determine a first application area.
Ultimately, whether beginning with a business outcome or with data assets, having a culture of innovation in the organization and especially in the C-suite and management teams is critical to success in AI projects. VPs, managers, and other heads of departments inside businesses will need to understand and communicate to the rest of the organization an assessment of the right AI strategies and technology solutions to move their company’s goals forward.
Most important of all is to get started.
Header Image Credit: Datameer