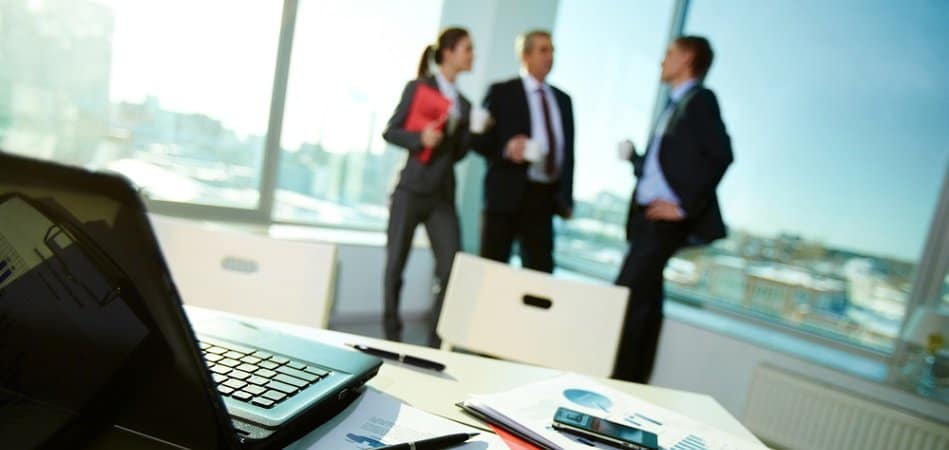
Artificial Intelligence has many applications in marketing and advertising. However, it may prove useful in the near-term future for businesses to start looking into AI solutions for after they generate a lead. AI already has applications for converting leads into paying customers and making progress on various steps in the sales process overall.
Sales enablement includes a myriad of processes from improving the operational efficiency of a sales team to closing a sale. Implementing AI for enterprise sales teams involves aligning the approaches of sales managers and the data scientists, who may not be used to working with data that in many ways can be difficult to quantify.
Businesses might face more challenges when implementing sales enablement platforms with AI capabilities than they would if they implemented consumer-facing AI applications, such as chatbots. To better understand how AI can help businesses improve their sales processes, we spoke with Amir Fishslevich, CEO of Incentives Solutions (makers of Joopy), and Vitaly Gordon, VP Data Science and Engineering at Salesforce.
According to Gordon, applying AI to sales enablement involves not just solving challenges that sales teams face, but also identifying customer issues. Data sharing from both these segments might be inherently complex due to privacy concerns. Gordon explains with an example:
One example is access to data. As a data scientist, I used to get familiar with the dataset I was working on, which is something that as a vendor giving service to customers, they’re not as comfortable with that as consumer data.
There is a whole array of privacy of how can we do machine learning without access to the data of the business transaction. Some of it is the most sensitive data of our customers and they are correct in their opinion that they don’t want people outside their organization to access it.
Privacy might only be one issue that large businesses face when implementing AI projects for sales enablement. The sheer scale of sales enablement for Fortune 500 companies might prove a big challenge in terms of managing all the incoming data.
That being said, an increasing number of organizations seem to have managed to implement AI capabilities into their sales processes. Many of the AI capabilities built into sales enablement platforms today serve the following functions:
- Lead Scoring
- Targeting Sales
- Lead generation
- Managing Sales Representatives
In this article, we’ll discuss the current state of AI for these aspects of the sales. We’ll also discuss how business leaders can prepare for:
- Implementing AI for Sales Enablement
- The Future of AI in Sales Enablement
We’ll begin our discussion with an overview of AI for automating the sales process, and then we’ll one-by-one present the current and future applications of AI for sales enablement with quotes from Fishslevich and Gordon:
Sales Process Automation – An Overview
Sales representatives’ jobs involve many repetitive tasks that need to be performed daily, such as finding the right products to pitch for each customer or prioritizing their leads. This might especially be true for sales teams that make higher ticket sales.
In industries such as electronic or tech, where there is a relatively high volume of sales, managing and organizing sales tasks might become challenging for sales teams. Additionally, businesses often need to guide customers through their purchase process even after a customer decides to buy.
In such cases, the volume of customers can make the sales process ripe for automation. Sales automation platforms can include AI capabilities that streamline some parts of the sales processes in businesses.
AI can likely help most in managing scale issues and personalizing the sales experiences for each lead. Many of the current applications involve augmenting the capabilities of existing human sales representatives through process automation.
Gordon states:
I would say we’ve had a lot of success in optimizing the job of sales individuals. If you think about sales, a lot of their work is process automation and following a playbook: not actual sales. They spend time on a lot of things that machines are very good at.
Lead Scoring
He explains further with an example of a sales representative who needs to rank leads in the order of who might be most likely to make a purchase. In such cases, there usually exists a lot of customer data that sales representatives need to scour through in order to generate ranking lists. This is a repetitive process that might be done every day by human sales reps for long periods of time.
Applying machine learning techniques to historical sales data from CRMs and training algorithms with sales datasets clearly indicating which leads converted into sales and which didn’t might help businesses automatically organize lead ranking lists and reduce the time spent by the sales representative in this process.
Similarly, in a use case where sales representatives need to track several leads at a time and decide the “Next Best Action” (NBA) lead, a lot of time is spent in studying the current sales process status for each lead.
Amir Fishslevich, CEO of Incentives Solutions, also adds:
The ‘Next Best Action’ recommendation is achieved by activating prescriptive analytics techniques. Prescriptive analytics is an area in artificial intelligence which relate to both descriptive and predictive analytics and focuses on the best direction that should be taken for a given, known scenario. The NBA recommendation can be given to sales reps on a daily basis and is recognized as a very effective tool to enroll sales reps to the organization’s business targets and help them achieve these targets as expected.
Targeted Sales
A key part of everyday business for sales teams across industries is using historical product and customer data to filter and segment prospects and leads. Sales teams might have to make crucial decisions and take calculated risks, taking into account both industry trends and business goals.
In the short term, sales automation software enhanced with AI will likely augment the capabilities of salespeople and improve the chances of forming relationships with potential customers and leads. For example, a salesperson at an automotive spare parts company might use AI to access information about their products, the manufacturers, and customers to personalize sales pitches.
If the spare parts firm received several orders for a failing spark plug in a particular car model, the AI module software can prompt the sales team with an alert to offer the manufacturer a higher volume of spark plugs at a profitable price.
Peter Schwartz, SVP Strategic Planning at Salesforce states:
I’d be more worried about being replaced by another salesperson who is empowered by intelligence than by a machine.
In the next two years, AI capabilities within Sales Enablement Platforms software will likely be applied to augment human sales reps with insights about how to better segment and filter lists of potential customers. A key area where AI might do better than humans in such applications might be its ability to identify trends and correlations in data that might have gone unnoticed by humans due to the sheer scale of the datasets.
Lead Generation
Personalizing sales and marketing initiatives to customers seems to be a key focus for businesses using AI today. C-level sales and marketing executives at top firms might find that AI has been demonstrated to yield reasonable results in helping to find insights in their sales data, which can lead to benefits such as the identification of new markets or customer segments.
For example, a pharmaceuticals sales team might use a lead generation platform with AI capabilities to identify trends in historical sales and customer data and use them to predict which customers in a locality might prefer a particular brand of their products.
AI today might be capable of detecting new leads by scouring through data and automatically extracting or categorizing information that might be useful. Machine learning can discover patterns in customer data and find cause-and-effects relationships between products and customers.
For example, AI software assisting an investment manager at a bank might prompt them to pitch a new mutual fund comprised entirely of eco-friendly companies to a particular customer whose personal data was tagged with the labels “green,” or “eco-friendly,” or “environmentally conscious.” AI software helps unearth such new lead opportunities by finding connections in sales data.
Managing Sales Representatives
Management by Objectives (MBO) is a practice which aims to increase organizational performance by aligning goals throughout an organization.
Ensuring that these goals are aligned requires the organization understand what sales reps’ motivations and personal targets might be.
Amir Fishslevich, Incentives Solutions CEO adds:
The main challenge regarding the MBO is how well the organization know[s] its employees and how much employees are aware to their way being motivated and achieving personal targets. We see that in many cases objectives which are set by the organization, with a full agreement and cooperation of the employees, appear to be inefficient in driving employees and sales reps.
For example, quota-based KPIs are set to employees who are functioning poorly under such conditions. On the other hand, there are employees who best function under competitive environments while their assigned KPIs and deployed business logic, for incentives and commission payments, hardly express competition-based incentive.
The employees’ behavior analysis capability is targeted to strengthen the linkage between the organization‘s objectives and employees’ personal behavior analysis, in order to improve the organization’s MBO definitions and business results.
Implementing AI for Sales Enablement – Challenges
The core component of any AI implementation is high-quality data from a variety of data sources. In comparison with consumer or customer applications for AI, the access and collection of data about employee behavioral patterns or sales records might be challenging for Tier1 organizations as well as for small and medium-sized companies.
Moreover, as many organizations and companies in different industry verticals are in a transition phase of all their systems from on-premise to the cloud, they need to ask themselves what data items can be loaded to the cloud to enable artificial intelligence capabilities.
Gordon suggests that automated machine learning, where businesses can implement machine learning models without a human in the loop, might be key to resolving some of the data privacy-related issues. On the other hand, emphasizes Fishslevich, there are use cases which do not necessarily deal with customers’ personal data and hence organizations need to carefully pay attention to each use case, regarding data privacy.
The Future of AI in Sales Enablement
Privacy and ethical considerations about using the personal data of employees might be another likely issue that businesses need to flesh out before getting into AI implementations in the enterprise. Gordon suggests businesses might think about the privacy concerns in terms of the trade-offs they might gain.
For example, this might be analogous to autonomous driving features in cars like Teslas might share data amongst themselves, in essence collectively “learning” from the unique behaviors of each car to adapt to new situations. But this kind of AI application might not be possible without sharing personal data from each car.
The experts we spoke to seemed to suggest that one likely possibility for the future might be that more businesses could find access to growing volumes of employee and customer data from emails, calendars, locations, meetings, and data from sensors in the workplace. In the next two to five years sales representatives could expect more levels of automation in repetitive tasks.
In the long term, with the amount and sources of data in businesses growing, AI capabilities, which are embedded in any sales enablement platform, might augment humans proactively by understanding the context in that data, thus automatically making suggestions for information sales reps might need next.
For instance, sales representatives in the future might “converse” with Siri-, Alexa-, or Google Home-types of platforms that proactively make suggestions and manage employee work schedules.
AI software might monitor sales contract risk in real-time by scouring through news and media to notify sales reps in case of events like a drop in competitor prices or prompting them to reply to an email with highly negative sentiment from a current lead.
Fishslevich shared his thoughts on the future of AI in sales enablement:
In light of the expected impact of artificial intelligence on sales processes and sales enablement platforms, companies and organizations are expected to invest more at the design process. In sales performance management (SPM) platforms, for example, that means investing in the assignment of commission models to sales reps, in order to create an optimized match between the organization targets, the commission model and the sales reps. The artificial intelligence is expected to provide a personal view regarding what type of incentives models and accelerators are most effective for each sales rep to maximize business results and payments
This article was sponsored by Incentives Solutions and was written, edited, and published in alignment with our transparent Emerj sponsored content guidelines. Learn more about reaching our AI-focused executive audience on our Emerj advertising page.
Header Image Credit: Introhive.com