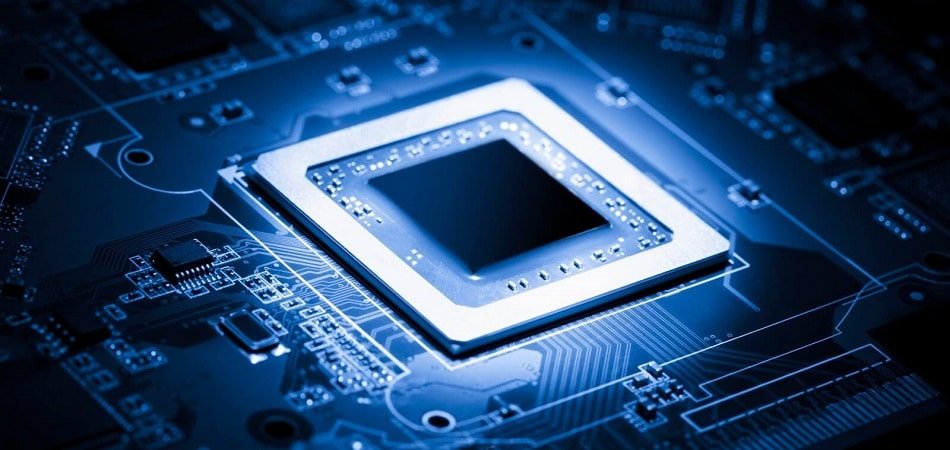
In this episode of the AI in Industry podcast, we speak with Marshall Choy, VP of Product at SambaNova, an AI hardware firm based in the Bay Area. SambaNova was founded by a number of Oracle and Sun Micro Systems alumni. We speak with Choy on two fundamental questions:
- How will business models fundamentally change with respect to new AI hardware capabilities?
- How can business leaders think about their AI hardware needs?
SambaNova is one of many firms that’s going to be advertising at the Kisaco Research AI Hardware Summit in Beijing June 4th and 5th.
Subscribe to our AI in Industry Podcast with your favorite podcast service:
Guest: Marshall Choy, VP of Product – SambaNova
Expertise: Product management, AI hardware
Brief Recognition: Prior to SambaNova, Choy was Vice President, Systems Product Management and Solutions Development at Oracle for over 8 years. He also spent over 11 years at Sun Microsystems in various leadership roles.
Interview Highlights
(03:30) What are the real shifts in business that occur with hardware?
MC: Speed is certainly one of the benefits that you’re always going to hear about, but it goes much, much broader and much deeper than that when it comes to AI and how a business is going to adopt that. And so, if you look at the way that things in the past have been done with different things like predictive analytics and big data, a lot of the focus has been around how we look at patterns and things that are going on.
Like in banking, for example, with automated threat detection systems. How do we review historical patterns and how do we then project those forward and forecast what’s going to happen and make best guesses about that?
But I think, in this example with threat detection, it’s going to be more around generating automated threat prevention systems. Whether that’s for fraud or counter-terrorism funding, anti-money laundering. It’s really about not just gleaning from past patterns of what’s going to happen, but really understanding what is really going to happen based on a number of other factors.
And so if you look again at retail, for example, you have automated customer service agents who right now you may have a big problem just getting that agent to understand what it is you are trying to say. In the future, it’s going to be much more about that agent, that automated customer service agent knowing what you meant to say versus just doing literal translations.
Understanding intent and context and being able to pry a much richer service to end users. We think that’s a big part of where this is going.
I think one of the imperatives here is to get started sooner than later because everybody is looking at this and the problems are just getting bigger and bigger. But the reality is with machine learning as the larger subset of AI that we’re talking about here is the more data, the more you train the system, the more accurate and precise and quick to answer the system gets.
In a sense, the faster it learns and the more intelligent it becomes to actually be able to provide those answers and those insights that we’re lacking today.
It really comes down to the ability to automate out a number of things that may take just a few seconds for a human to decide on mundane and repetitive tasks. Let’s take another example. If you look at healthcare and diagnostic systems for medical imaging where you’re looking at some sort of an X-ray for some anomaly, these are oftentimes very, very human-intensive things.
And I think we’ve all unfortunately heard the stories of a friend or a relative who has been in for a scan and they march to the person who was in charge of looking at the scan and applaud their valiant and heroic efforts because they found this tumor that was overlooked by countless other doctors.
While well certainly that effort is successful, we can increase the success rates so that we can turn that multistage, multiperson process of passing around the film and examining and analyzing it to just a couple of seconds or even less than a second. And so, therefore, the healthcare provider can focus less on the analyzing and diagnosis part and more on the treatment side of things, which is a higher value-add to the patient and the end user customer then than sitting there staring at a medical chart.
We talk to a lot of customers, and a lot of the problems they have are just insurmountable in terms of the amount of time and effort and people resources to solve them. One of the byproducts of the speed is if I can take something that was going to take you 30 days and bring that down to three minutes, suddenly something that you gave up on trying to solve for that was going to be a six-month problem is now concatenated to a significantly more doable timeframe. And so the realm of possibilities quickly opens up in terms of what’s solvable tomorrow versus what’s currently unsolvable today.
(12:00) How should business leaders think about their AI hardware needs?
MC: I think for most business leaders, their interaction point with the technology stack is going to be starting with the business application. And rightfully so. Hardware is something several layers below that and is oftentimes quite abstracted from the overall business application. But my belief is the full stack actually matters.
And if you look at what’s happened over the last decade or so, software’s kind of eaten the world and changed things quite a bit. And machine learning, in turn, is really changing the way that software is developed and run. So we’re in much more of a software-led, software-defined world than ever, which we think is the right approach to this.
Hardware leading software just doesn’t make a lot of sense. And so engaging with hardware from a perspective of using infrastructure that is thoughtfully designed from a “software first” mentality and therefore designed to accommodate the requirements and needs for data flow and data processing from the upper level software application, we think is the right way to think about this and legacy implementations just don’t provide it because they weren’t built with that current mindset.
If you look at the traditional world of transactional processing software systems, whether that be an ERP system or a core banking system or a taxation system in the public sector, the underlying software capabilities are pretty similar in terms of how they’re developed and how they’re implemented.
The developer writes a very deterministic set of instructions that are literally interpreted by the computer and we are obsessively focused on “n”th degree of accuracy. For example, if you’re a government collecting taxes, you want to collect the taxes down to the penny. If you want to check your bank account statement, you don’t want that to be estimated. You want that to be very deterministic and accurate.
With AI and machine learning though, it’s a little bit different. Let’s look at a different example.
Maybe it’s a service recommendation system that could be in banking, that can be in retail, that could be a citizen services site for government and public sector. The key there is that the system is going to be written very differently. It’s going to be written much more probabilistically. And so using machine learning techniques, the developer’s role in this is now to provide training data and the application is actually going to be written by the machine itself.
We’re not actually after a hundred percent accuracy. To get to the right recommendation, you probably need to get to 70, 80% accuracy. And as a result, we can actually get to that answer faster and with a greater level of accuracy. And so that’s kind of the big difference in in the software model. Then that clearly has implications on the hardware underneath.
What I encourage people to do is don’t look at the low-hanging fruit as areas where they can achieve cost reductions or cost savings or just eliminating labor cost and effort. I mean those are very tactical solutions that can provide some short term fixes, but at the end of the day, what you want to focus on are areas that are going to help you to achieve new revenue streams, create new products and new service offerings.
That’s a much more strategic view. And where that comes in is again, it’s a reversal of the old world of predictive analytics where again it’s more about trying to forecast based on the historical patterns of what’s going on, but more using AI to be able to foresee the future and different trends based on other attributes. And so that’s kind of where we see things being applied much more intelligently.
Subscribe to our AI in Industry Podcast with your favorite podcast service:
This article was sponsored by Kisaco Research, and was written, edited and published in alignment with our transparent Emerj sponsored content guidelines. Learn more about reaching our AI-focused executive audience on our Emerj advertising page.
Header Image Credit: Techspot