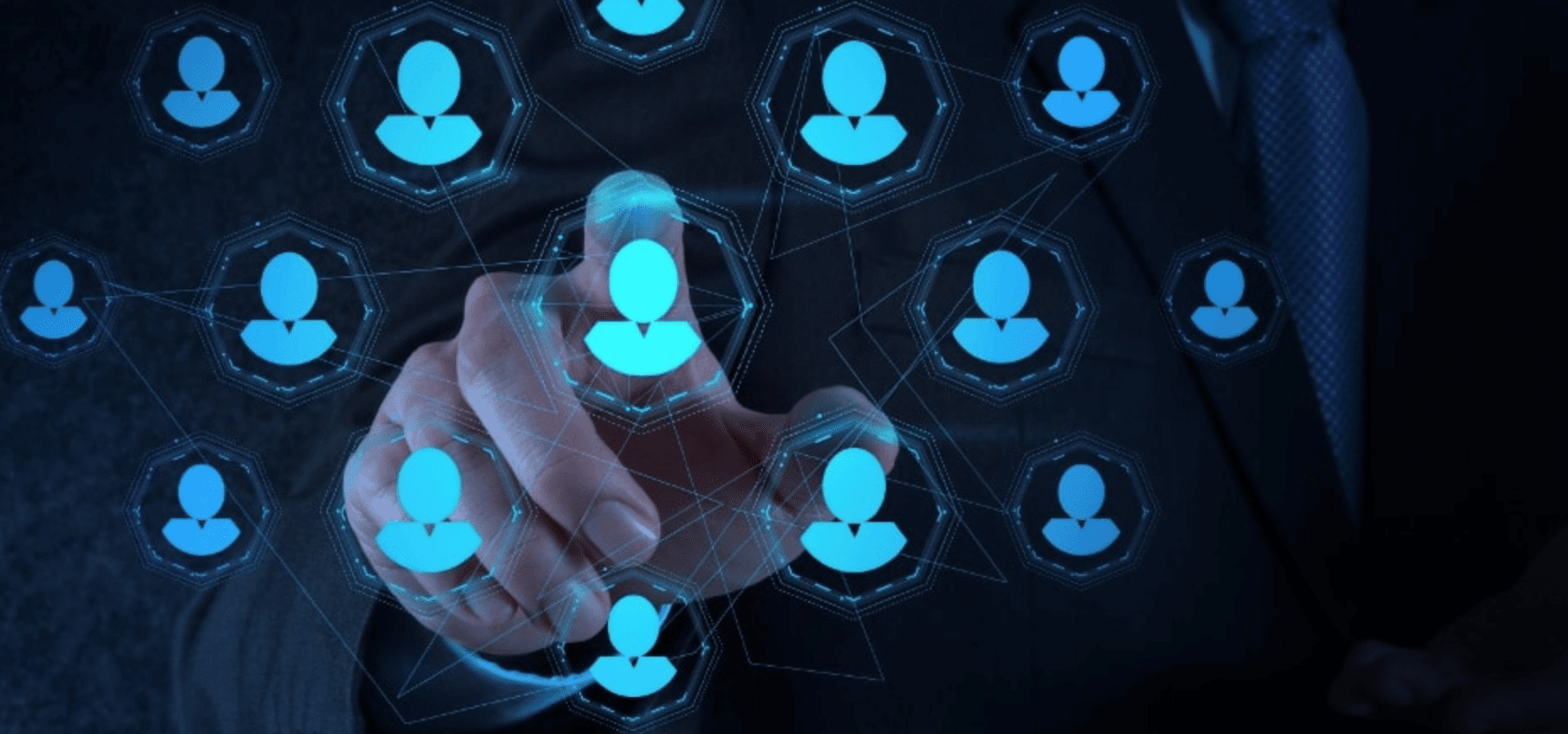
When we search on Google or Amazon, we’re reminded of the improved capabilities of artificial intelligence over the last half decade.
What we often don’t realize is the role that human beings have in tagging and manually working through near-infinite reams of data to develop genuinely relevant search.
Sure, data scientists and ML specialists must construct a search system – but for the time being – much of the “human-like” results that we get in the world of social media, search engines, and Commerce – comes from – well, humans.
This week on AI in Industry we interview Vito Vishnepolsky of ClickWorker. Clickworker is a large and well-rated microtasking marketplace. Clickworker cloud technology platform caters data management and web research services as well as AI algorithms training. The firm claims to have over one million workers on its global platform as of September 2017.
Vito’s perspective is valuable because he has a finger on the pulse of crowdsourced demand, handing business development for various crowdsourced AI support services – both for tech giants and startups.
Subscribe to our AI in Industry Podcast with your favorite podcast service:
Below, we’ll break down the AI techniques that leverage crowdsourced labor to improve search, and we also explore what Vito believes to be the future direction of online search.
Crowdsourced Techniques Behind Improving Search
Search improvement isn’t done with a single strategy, and there are a number of strategies used to continually ensure that search applications deliver “human-like” results. Below we’ll explore four of these algorithm techniques, and how they might apply in a business use case.
Relationship Ranking
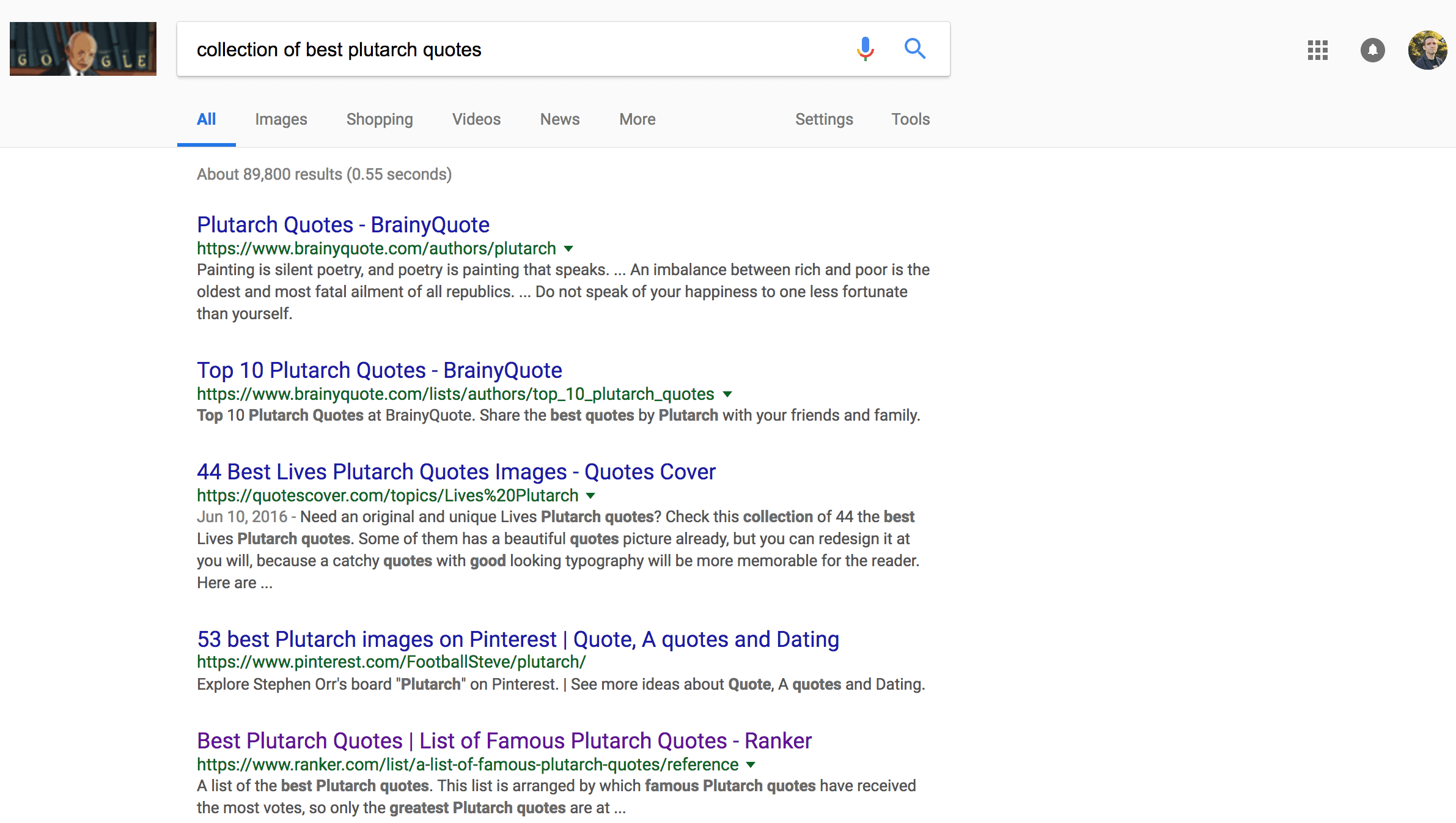
Determining related keywords is an important task for search applications, but it’s not always an easy task. In a sentence with 15 words, which 3 or 4 words are most likely to refer to the full (15-word) query?
Humans don’t always type fully structured sentences into search applications, and when a short query is entered, a machine still needs as much context as possible around that event to determine what to serve to the user – and Vito tells us that this is a challenge suited to crowdsourcing.
An example of a search query might be:
“April the giraffe finally gives birth to a healthy male calf at Animal Adventure Park”
We then need to read the sentence and choose different sets of keywords according to the relevancy. In that way we’re doing some reverse engineering in order to help the ML algorithms to help find this term. A crowdsourced worker might take a cluster of keywords, and ranking them all on a 1-4 scale of how relevant they are for the search query.
Suggested terms might include:
“Animal Adventure Park giraffe birth”
“April giraffe baby”
“Baby giraffe at the zoo”
“Birth of a baby giraffe”
Permutations like this come up on screen under the original term, and crowdsourced workers will identify which of these related most to the original sentence by scoring them individually.
Without this added layering of human interpretation, a search engine might not realize immediate that they news event “April the giraffe finally gives birth to a healthy male calf at Animal Adventure Park” was more closely related to “Animal Adventure Park giraffe birth” than “Baby giraffe at the zoo” (which doesn’t mention April – the mother – or Animal Adventure Park – the location).
In Vito’s words:
In that way we are doing some reverse engineering of the search engine system. We’re training the system to know the mostly closely related keywords to this specific enquiry.
Manually updating a search engine with this added input of human perception helps to make sure that events or entities can be found quickly, by taking out some of the guesswork around which related terms most closely tie to a specific desired item.
Vito tells us that the determination phrases and related terms is something determined by the client company’s data scientists, not by Clickworker themselves. Data science teams determine their own unique needs and structure the tasks, and Clickworker takes over by distributing that work and delivering the resulting data to the client.
Search Precision
Query precision is a task that involves scoring search results based on their relevance across a unique set of pre-determined criterion.
An example of a search query entered by a user might be:
“Summer rock and roll concerts in Chicago”
This query might have the following attribute parameters:
Attribute 1: Location, Value: Chicago, IL
Attribute 2: Music, Value: Rock and Roll
Attribute 3: Time range, Value: May 10th 2018 to September 15th 2018
In order to do quest precision and “distribute” the relevance task via crowdsourcing, these initial structures have to have been thought up and determined ahead of time by data scientists. Crowdsourced workers will simply select work to score searches along these defined criterion ranges, on a scale of 1-4 for relevance.
In the case of Clickworker, it just distributes tasks to the right workers – it is the client companies that have to do the data science and parameter determination work themselves. Clients integrate with an API, or give a spreadsheet input with the task, and Clickworker’s workers delivers the output through the same spreadsheet.
Another example of a search query entered by a user might be:
“How to use a table saw”
This query might have the following attribute parameters:
Attribute 1: Hobby / Topic, Value: Vintage
Attribute 2: Content Type, Value: Instruction
With enough human scoring, algorithms can be trained to optimize for the right “weight” of different terms or attributes in order to consistently show results that satisfy a searcher’s intent. Combined with data from real users (i.e. the click-through data from search listings, and the time-on-page on selected search result pages), this level of human curation helps keep algorithms sharp.
Vito mentions that this crowdsourced training technique can be particularly important in situations of sparse data, such as:
- New terminology (in science or technology, for example). New terms without a previous precedent may not be immediately understood by machines, and may benefit from human clarification.
- Issues related to current events. The example we used above about the new Google phone release is one such example, and we can imagine many others in industry, politics and other sectors. Distinguishing between many similar news events may involve nuances in search terms and language that aren’t intuited by machines, but come naturally to people.
- Foreign languages. A search engine operating in Thailand will need to have training and phrases in the Thai language. With much less search volume and historical training data that English or Mandarin, a search engine might benefit from additional human training.
Listing Precision
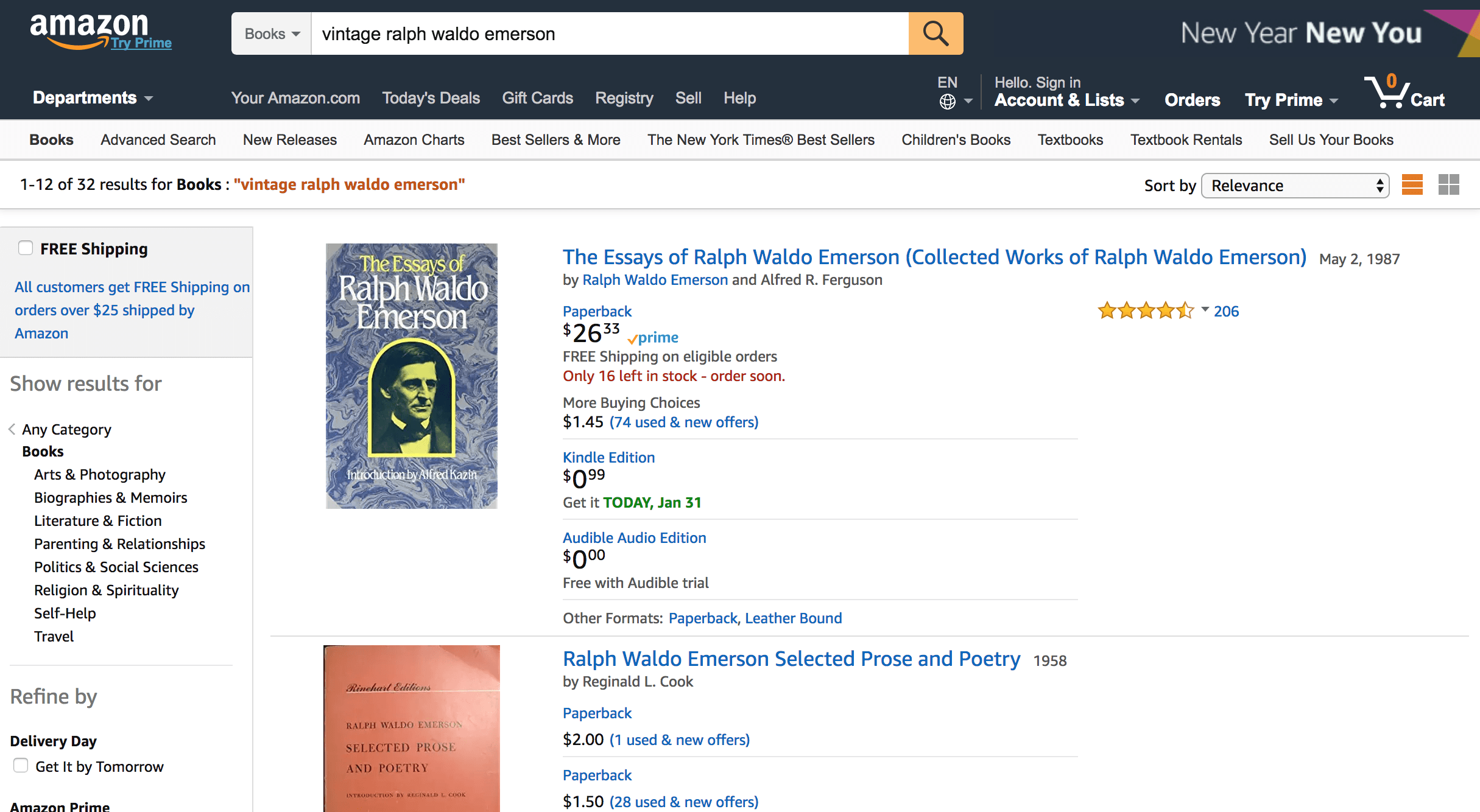
While CareerBuilder.com might respond to queries for jobs or applicants, and EventBrite.com might respond to queries for events or concerts, eCommerce stores must reply to queries with products, and this task is somewhat similar to how it would be in other search applications.
An example of an eCommerce query entered by a user might be:
“Green plastic coat hanger”
This query might have the following attribute parameters:
Attribute 1: Material, Value: Plastic
Attribute 2: Color, Value: Green
Attribute 3: Home Goods, Value: Coat Hanger
As with the previous example, the parameters needed and the crowdsourced task required would need to be defined by human data scientists before sending the project along to be micro-tasked.
The same idea could be applied to more or less any eCommerce item. An example of a search query entered by a user might be:
“Vintage equestrian shoes”
This query might have the following attribute parameters:
Attribute 1: Style A, Value: Vintage
Attribute 2: Style B, Value: Equestrian
Attribute 3: Apparel Item, Value: Shoes
In addition, “listing” could apply to a variety of search results other than online products. The same kind of human scoring and training might be used for:
- Listings for homes or rental properties (or sites like AirBnb.com)
- Listings for company profiles of service providers (say, on a platform like Thumbtack.com or Houzz.com)
- Listings for jobs (Monster.com, among others)
- Personal profiles on a blogging or social network site (like Tumblr or Facebook)
- Etc…
For businesses that rely on their ability to meet a searcher’s intent, any of these listing types may require robust human effort to refine the search results to be as intuitive as possible.
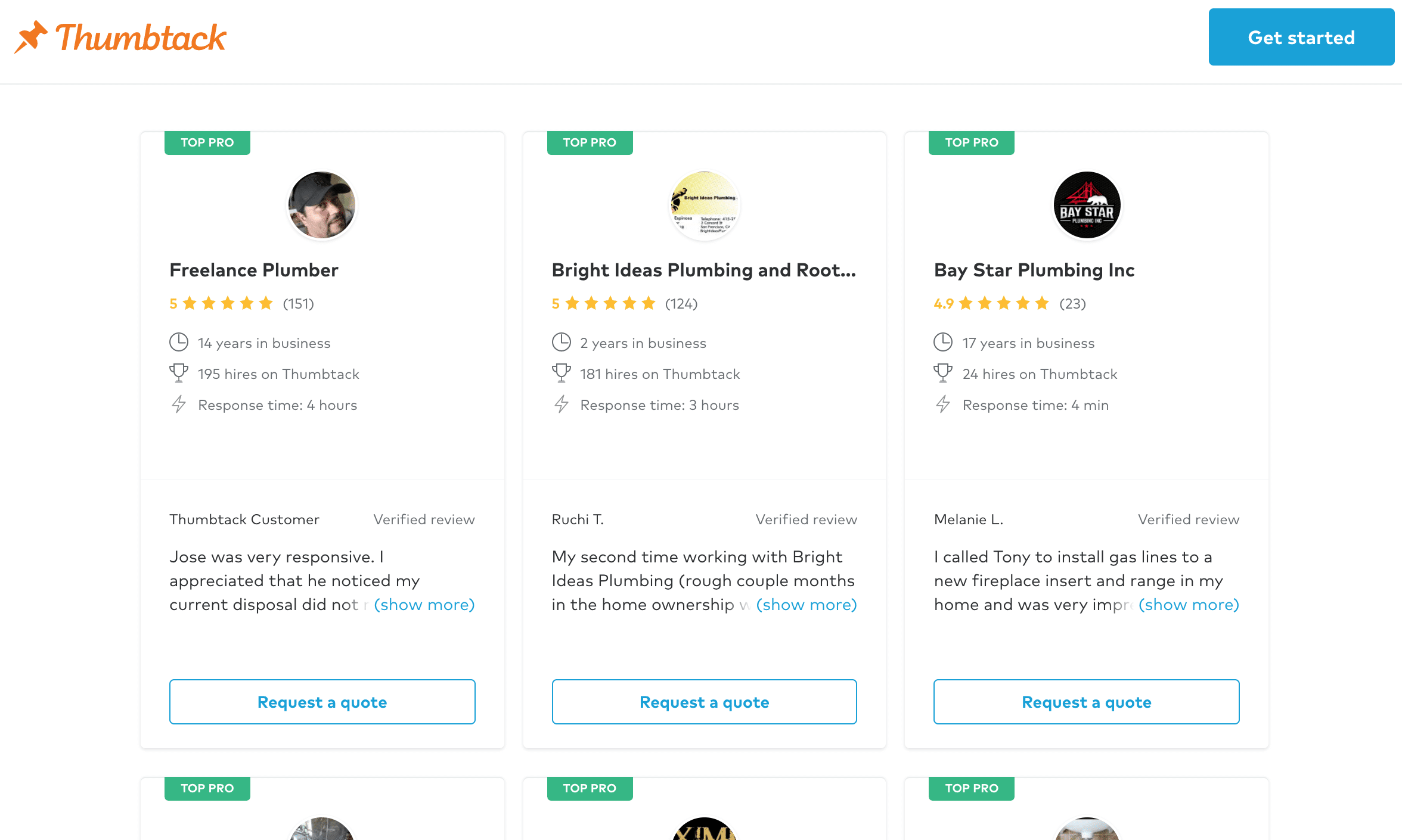
The Future of Voice Search and Machine Vision
While Clickworker needs to handle client requests for micro tasking today, the company obviously needs to have a finger on the pulse of where the industry is heading (so that they can fulfill on future demand and catch opportunities as they develop).
This is one of the reasons I like to ask executives about the near-term future of their industries – because it’s insight that they live and die by, and they can often bring insights to the table that an outside analyst might never find online.
Vito identified voice and image recognition as important burgeoning areas of interest from companies developing search applications.
As for voice, training systems on different languages seems to be a task well suited for crowdsourcing. Vito explains:
We are training machines to recognize people speaking with different accents. Clickworker has crowd in 140 different countries – about 33% in Asia, 33% in North America, and 33% in Europe. So we often record data sets ot train voice searches in different languages. With English, we might train a system on a UK accent or Australian accent – depending on [the client’s] geographies.
If a device like Amazon’s Alexa wants to begin selling in Finland or Mongolia, it would be unrealistic for Amazon to hire dozens of native Finnish or Mongolian speakers. Rather, a company like Amazon might have a good sense of the most common phrases and tasks for Alexa, and request that various permutations of those popular phrases be recorded (by crowdsourced workers) and processed by a machine learning system to get it started.
Vito states that Clickworker has seen a significant increase in demand for machine vision training:
I think that machine vision is the most popular field gaining steam in 2018. We have a lot of companies in need to tagging images and identifying objects and applying categorization… We see this trend in eCommerce companies to recognize products in an image, or security companies who are marking images from drones or unmanned aerial vehicles.
As it turns out, machine vision has some strong intersections with search optimization, and Vito believes that the two will only be more interconnected as search applications become more sophisticated and intuitive.
For example, when an eCommerce company has photos tagged by crowdsourced workers, people searching for a certain keyword might be able to see images related to that keyword more quickly (i.e. A user searching for “red rain boots” might find more images and products that match that description thanks to searchable images).
In the future, it’s possible that users will be able to take images on their phone and have AI systems find those same products online (applications of this kind exist, but aren’t yet widespread), or users might be able to find existing online images and load them into a machine vision system to find products (from clothing to tech gadgets and more) that they might like to buy.
Subscribe to our AI in Industry Podcast with your favorite podcast service:
This article was written in partnership with Clickworker. For more information about content and promotional partnerships with Emerj, visit the Emerj Partnerships page.
Examples of queries above are for illustrative purposes and aren’t drawn directly from Clickworker’s clients or case studies for the purpose of confidentiality.
Header image credit: Inside HR