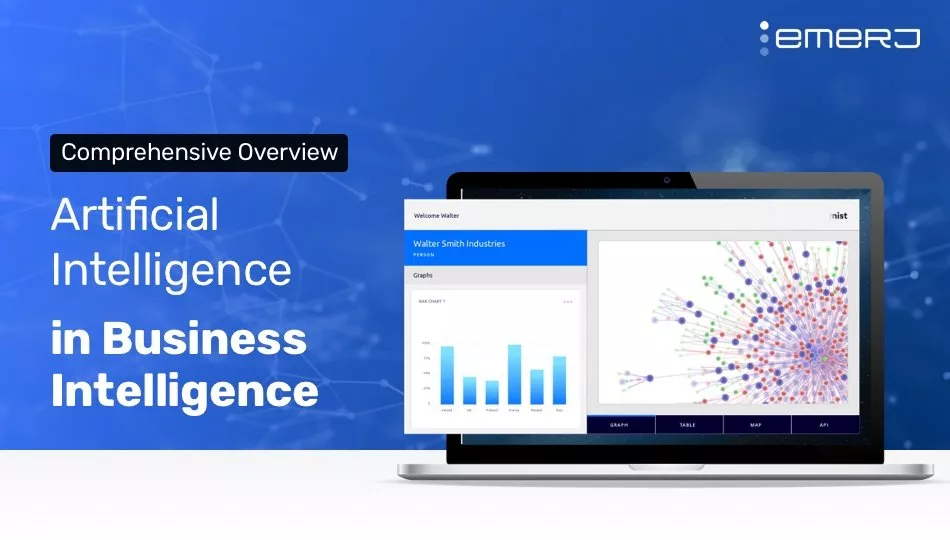
Enterprise seems to be entering a new era ruled by data. What was once the realm of science fiction, AI in business intelligence is evolving into everyday business as we know it. Companies can now use machines algorithms to identify trends and insights in vast reams of data and make faster decisions that potentially position them to be competitive in real-time.
It’s not a simple process for companies to incorporate machine learning into their existing business intelligence systems, though Skymind CEO and past Emerj podcast guest Chris Nicholson advises that it doesn’t have to be daunting. “AI is just a box,” he says. “Math and code. If this, then that. That is the simplest way to describe it.” Organizing data collection and testing an algorithm with this data for accuracy over the first few months are is where many businesses get stuck.
But as AI has gained momentum, prominent application providers have gone beyond creating traditional software to developing more holistic platforms and solutions that better automate business intelligence and analytics processes. Major vendors— including General Electric, SAP, and Siemens—offer such software suites and operating systems, but there are a growing number of emerging providers in the market as well. In this guide, we provide six examples of AI platform providers. Each example covers the following:
- Brief overview of the product or service
- Successful case studies
- Potential use cases in industry
Based on our past interviews with executives and investors in the field, we predict that business intelligence applications will be one of the fastest growing areas for leveraging AI technology over the next five to 10 years.
Business Intelligence Apps Built on Machine Learning
SAP – AI for Turning Databases into Useful Intel
HANA is SAP’s cloud platform that companies use to manage databases of information they have collected. In short, it replicates and ingests structured data, such as sales transactions or customer information, from relational databases, apps, and other sources.
The platform can be installed to run on-premise through a company’s servers, or via the cloud. HANA takes in information gathered from access points across the business—including mobile and desktop computers, financial transactions, sensors, and equipment at production plants. If your sales staff uses company smartphones or tablets in the field to record purchase orders, data from those transactions can be analyzed and understood by HANA to spot trends and irregularities.
Walmart, for example, has been using HANA to process its high volume of transaction records (the company operates more than 11,000 stores) within seconds. At a conference hosted by SAP in 2015, then-CIO Karenann Terrell described why Walmart chose to use HANA in order to operate faster and control back office costs by consolidating the processes and resources needed to handle the work.
How Walmart uses HANA
Unexpected differences can crop up most anywhere in the process of conducting business; it might be an excess product order that seems odd for a particular customer or machinery at a factory that starts to run slower than it should. Machine learning can be used to automatically call attention to such variances. For example, if a factory manager has an application installed on their computer to monitor the equipment on an assembly line, data from a slowdown in production could be collected and processed through HANA. The gathered results can be queried to determine if a new course of action is needed, such as a service inspection of the equipment.
SAP says that HANA performs differently from comparable platforms by storing replicated data in RAM rather than on disk. That makes it possible to access the data in real-time for use with applications and analytics built on top of the HANA platform for faster decision making.
The intent with HANA and other machine learning solutions is to make data-driven decisions that are potentially better informed. It is possible for small and midsize companies, not just enterprises, to explore using this kind of technology in different segments of their business—if the solution can fit within their budget. “This can be found in every organization whether it’s for HR, risk, or marketing,” says Ronen Meiri, CTO for DMWay, a provider of machine learning for predictive analytics.
The anticipated benefits of using machine learning platforms for business intelligence include infrastructure cost reductions and operational efficiency. In a report sponsored by SAP, 10 organizations that use HANA said they expect to realize an average five-year, return on investment of 575%. They also projected an average annual benefit of $19.27 million per organization by using HANA, compared with average annual investment of $2.41 million over five years. International Data Corp., a subsidiary of IDG, conducted the survey and produced the report on behalf of SAP. The names of the organizations surveyed were not disclosed.
DOMO – AI for Business Dashboards
It is not just the giants like SAP developing machine learning platforms for business. Domo, a fast-growing business management software company that’s raised over $500 million in funding, has created a dashboard that gathers information to help companies make decisions. The cloud-based dashboard can scale with the size of the company, so it can be used by teams as few as 50 or by much larger enterprises. There are more than 400 native software connectors that let Domo collect data from third-party apps, which can be used to offer insights and give context to business intelligence.
This gives companies using Domo a way to pull data from Salesforce, Square, Facebook, Shopify, and many other applications that they use to gain insight on their customers, sales, or product inventory. For instance, Domo users who are merchants can extract data from their Shopify point-of-sale and e-commerce software, which is used to manage online stores. The extracted information can be used to generate reports and spot trends in real-time, such as in product performance, which can be shared to any device used by the company.
In March, Domo announced Mr. Roboto, a set of new features for the platform that draw upon AI, machine learning, and predictive analytics. The expectation is for Mr. Roboto to offer recommendations and insights to decision makers at companies. Once these features are rolled out, expected in late spring 2017, the platform is supposed to issue new alerts and notifications for significant changes, such as the detection of anomalies or new patterns in data (similar to approaches used in cyber security already).
Detecting these changes and patterns is expected to fuel the predictive analytics side of Mr. Roboto and help companies predict the return on investment for marketing in real-time, customer churn, and sales forecasts.
According to Domo, companies such as MasterCard, Univision, eBay, the Honest Co., and SAB Miller use its platform. Television broadcaster Univision offered up a testimonial about the way it uses Domo to give more visibility to its own data, which is then used to unify and focus targeted campaigns. Univision said it uses the Domo platform with connectors for such applications as Google Analytics, Facebook, and Adobe Analytics to get more value from its programmatic advertising. “By launching Domo, we were able to quickly optimize and achieve an 80 percent growth in yield during our first quarter,” said David Katz, Univision’s VP general manager for programmatic revenue and operations.
Apptus – AI in Sales Enablement
There are numerous ways for machine learning to enhance applications, including those from Apptus, which offer recommendations on actions that companies can take to boost their sales channels. Apptus says it specializes in the connection between a customer’s intent to buy and the realization of revenue by a company.
The Apptus eSales solution is designed to, among other features, automate merchandising based on a predictive understanding of consumers. The software combines big data and machine learning to determine which products might appeal to a potential customer as they search online or get recommendations.
For example, when a customer visits an online store that uses Apptus eSales and starts to type in search terms to look up products, the machine learning solution can predict and automatically display related search phrases. It can also display products associated with those search terms.
Companies of varying size use Apptus, such as automated bookseller Bokus.com in Sweden, which according to its testimonial has about 30 employees. Bokus, which needed to keep overhead at a minimum, said that tapping into automated technology to convert customers is a way to help achieve that goal. For instance, the eCommerce company reported that its average turnover of customers increased 100% for each open of its digital, personalized recommendations newsletter.
AI and machine learning platforms are getting better at predictive tasks, such as determining what customers might want based on the information that they are fed. In an interview strictly for this article, Nicholson stated that deep learning, a subset of machine learning, is in many cases hitting an accuracy of 96% in interpreting data. “That is the upper limit of what humans can do,” he points out.
Though the technology is still in its early days, Amr Awadallah, founder and CTO at machine learning and software company Cloudera, says deep learning is already adept at prediction and anomaly detection. It is not perfect yet, but it is getting easier for deep learning networks to understand what information is relevant. “You don’t have to tell the algorithm which things to look at,” he says. “You can give it the raw input data and it can figure it out for itself.”
Avanade – AI for Business Insights
Avanade is a joint venture between Microsoft and Accenture that leverages the Cortana Intelligence Suite and other solutions for predictive analytics and data-based insights.
Insurance company Pacific Specialty tapped Avanade to build an analytics platform with the intent of giving its staff more perspective and insight on the business. The goal was to use customer and policy data to help the team drive more growth. By understanding policyholder behavior and trends through analytics, the idea was to better advise the development of new products.
Avanade says the world is headed towards a future populated with smart technologies where machines take on more of the work that people traditionally do. According to a study commissioned by Avanade, a survey of 500 business and IT leaders from around the world revealed that they expect to see 33% increases in revenue as a result of smart technologies. They also believe this will lead to new and redefined job roles for employees, as well as more benefits to customers. The survey, however, did not specify which specific jobs could be changed by the adoption of smart technology.
So far, these use cases point to machine learning largely used in service sectors, such as insurance and retail, to address tasks related to customers, sales, and operations; however, AI has also merged with BI applications in manufacturing and industrial domains.
BI and AI – Applications in Heavy Industry
General Electric – Predicting Repairs and Upkeep for Machinery
The increasing prevalence of sensors in machinery, vehicles, production plants, and other hard equipment spaces means physical equipment can be digitized and be monitored by artificial intelligence, a topic we’ve covered before in machine learning applications in industry. The Internet of Things is not just about consumer gadgets; commercial trucks, trains, oil rigs, and cargo ships can all be digitized, monitored, and assessed via networks. Oil and gas, aviation, and other industries, for example, have been using General Electric’s Predix operating system, which powers industrial apps to process the historic performance data of equipment. That can be used to discern a variety of operational outcomes such as when machinery might fail.
Predix is not just for rudimentary, small-scale logistics management; it can take vast amounts of information recorded over time to develop its forecasts. This is done through apps developed by GE as well as third parties. Accenture’s Intelligent Pipeline Solution, for instance, is used to monitor millions of miles of oil pipelines around the world. That includes taking data from pipeline assets and external sources to manage safety and how resources are used.
Aircraft operators use apps, such as the Aircraft Landing Gear Prognostics from GE and Infosy, built on Predix as well. The prognostics app lets airline engineering crews see how long the landing gear can remain in service before a plane needs to be put in for service. Creating a maintenance schedule based on that information is intended to reduce unexpected equipment issues and flight delays.
Predictive analytics, says Awadallah, forecasts the future as a function of the past. It can calculate when maintenance is needed for devices, cars, trucks, and drilling machines and then schedule repairs and upkeep before a severe failure occurs. Navistar, a manufacturer of commercial trucks, has sensors in its products that analyze brakes, lights, and engines, says Awadallah. That adds value to maintenance services by detecting when mechanics need to get under the hood. This can minimize downtime, and even schedule maintenance as part of a truck’s regular travel route and reduce costs of operation.
Applying machine learning can also boost the performance of some equipment. In one instance, eCommerce and shipping provider Pitney Bowes built a software solution on top of Predix that the company expects to increase its machine yield by 20%.
Machines built by Pitney Bowes include postage meters, sorters, printers, and inserters for producing and moving mail. By installing sensors into its machines, their performance can be more closely monitored through the Predix platform. The company claims that much of the 150 million pieces of mail produced daily in the United States pass through its machines.
Siemens – AI for Monitoring Machine Fleets and Factories
The importance of monitoring how industrial equipment will perform has compelled other software providers such as Siemens to put their machine learning technology to work in this space. In March 2016, Siemens launched its MindSphere open industry cloud platform in beta.
MindSphere was designed to provide monitoring of machine fleets for service needs through machine tool analytics and drive train analytics. The application can be used by industrial companies to keep track of machine tools at plants around the world and see performance stats of their assets. This can help schedule preventive maintenance and manage how their equipment is used to improve their operational lifespan.
Comparable to Predix, MindSphere works with machines and plants regardless of the manufacturer. The intent is to help plant operators increase the uptime of their equipment and make maintenance more efficient by assessing when a piece of machinery is expected to breakdown. Furthermore, machine builders may see reductions on expenses related to warranty repairs by virtue of their machines running smoothly for longer.
Siemens says MindSphere, developed with SAP, companies that use MindSphere get a box that connects to their machines and collects data to show how the machines are operating.
Closing Thoughts on AI in Business Intelligence
This is a potential threshold moment for business and industry, where machine learning might weave its way further into how operations are handled, the way decisions are made, and resources get managed. It will depend on whether or not businesses collectively find real value in AI; the investment in the technology must prove its worth.
Nicholson notes that though the accuracy and capabilities of deep learning have increased, the technology is still trickling out into the world among early adopters. The next phase, he says, will be about whether or not such resources will flow more freely and be embraced by the business community at large. “The future is already here,” he says, citing cyberpunk Author William Gibson, “It’s just not widely distributed.”
Emerj for Enterprise Leaders
Enterprise leaders use Emerj AI Opportunity Landscapes to validate a powerful AI strategy, or to select high-ROI AI projects.
Clients might begin with an area of focus – such as business intelligence – or they may simply be looking for the best low-hanging fruit opportunities for AI to deliver value. Using Emerj’s scored and sorted map of industry-specific use-cases and ROI data, leaders gain confidence in their AI investments that would be impossible otherwise. Contact us to learn more.