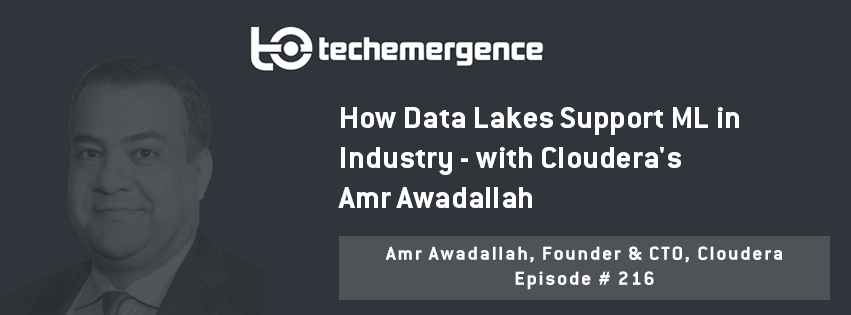
Episode Summary: If you’re going to apply machine learning (ML) in a business context, you need a lot of data, and algorithms across the board perform better with more recent, rich, and relevant data. Today, there are companies whose entire business models are predicated on helping others make sense of and use of this type of information, as more entities look for the first place to apply ML in their organization. In this episode, we speak with the CTO and Co-Founder of one such company—Palo Alto-based Cloudera. CTO Amr Awadallah, PhD, speaks with us this week about where he sees “data lakes” (or “data hubs”, Cloudera’s preferred term) and warehouses play an important role in ML applications in business. Based on his experiences helping a variety of companies in many countries set up data lakes, Amwadallah is able to distill and communicate these uses in three broad categories that apply across industries as companies look to apply ML applications to solve tough problems and ask more complex questions using unstructured data.
Expertise: Internet infrastructure/systems architecture, distributed systems, advanced analytics and data mining, search (as in web search), application layer protocol design
Brief Recognition: Before co-founding Cloudera in 2008, Amr Awadallah was an entrepreneur-in-residence at Accel Partners. Prior to joining Accel, he served as vice president of Product Intelligence Engineering at Yahoo!, and ran one of the very first organizations to use Hadoop for data analysis and business intelligence. Amr joined Yahoo after they acquired his first startup, VivaSmart, in July of 2000. Amr holds bachelor’s and master’s degrees in Electrical Engineering from Cairo University, Egypt, and a doctorate in Electrical Engineering from Stanford University.
Current Affiliations: CTO and Co-founder of Cloudera
Big Ideas:
1 – Data hubs enable building and scaling “segment-of-one” data models.
Personalized segmentation at scale helps correct the data world’s earlier challenge of false positives.
2 – Rich and relevant data inputs is more important than the complexity of the machine learning algorithm.
This type of big, meaningful data is helping change the way that businesses and industries operate, as well as consumer behavior.
Interview Highlights:
The following is a condensed version of the full audio interview, which is available in the above links on Emerj’s SoundCloud and iTunes stations.
(2:09) How do you folks draw a distinction between the definition of data warehouse and this newer terms that people may have heard some buzz around, the “data lake”?
Amr Awadallah: The industry overall does refer to it as data lake, we at Cloudera use the term data hub instead….but they are really the same thing…the main difference really is the enterprise warehouses were focused on SQL, SQLl was their genesis, and the characteristics have two sides: one side is the data itself tends to be very structured…and the only way you can really ask questions is using the SQL language itself, so the data lake is essentially an evolution that became a natural progression of the fact that we have so many other types of data besides structured data.
…And they needed a place where they could keep all this data side by side…but then also ask the bigger questions out of that data; we wanted to be able to go beyond SQL for the problems and types of questions that cannot be answered by SQL.
(5:05) Walk us through some of those examples that may have been impossible in SWL but is possible now with these alternative ways to store information.
AA: First, I will say it’s not impossible to do in SQL, which has so many extensions…it’s just very hard, it’s not natural…if you want to do a predictive model…SQL is not really built for that…it becomes much more natural and easier to be using something like Spark or using MapReduce. So, for example, we have a large hospital that we work with and they built a system that can collect all of the signals from a (premature) baby…they analyzed all of the signals coming out of the baby; some of these signals are the temperature, the heart-rate, some of them are motion…and by analyzing these signals and using some nurse experts…they came up with a predictive model that can show, on a screen, words that explain what the baby is going through…so we’re able to put words in the baby’s mouth using the signals that are coming out of their body…
(8:35) Most people are familiar with the way the old databases are done; where are are we tucking all of that information now that’s so hard to quantify in the same basic way as a transaction?
AA: To explain the question, I’d like to go to an analogy that I use to communicate the power of this new platform. I refer to this new platform, the data lake (the data hub as we prefer calling) as the “smartphone of big data”…the power of our platform is that it can take pictures, meaning it can do SQL but it can do a lot more than that at the same time, which is the same with a smartphone. The power and convenience of a smartphone is…once I capture that data, meaning take a picture…I can now do many, many things with that picture; I can process the picture, I can email it, upload it in Instagram, etc. versus when you take a picture with a digital camera, that’s it
…in other words, in this example of the neonatal intensive care, we still use SQL at the beginning to aggregate the different signals coming in…but then we can now shift and use Spark, a distributed processing programming language, to analyze these counts that came out of SQL and produce the predictive model that decides whether the baby is upset wth the light or the temperature or wants to eat, so the power of this platform is really…that it can bring different types of computation together to solve bigger problems.
(11:55) What is the role of a data lake in those bigger ML applications, and are there any other examples that you can walk people through?
AA: This (Cloudera) is a very powerful platform that can be used for many things…across many different industries…that said, we worked with many companies over the last eight years, and three key themes have emerged…and these these themes fall into these three categories: 1) The first one is what we refer to as customer 360, or how can we drive better customer insights, 2) The second one is the IoT, which is an acronym of how we can use our data from the real world to make our products and services much better at what they do, 3) And then the last key category…is how can we lower business risk, and lowering business risk includes a number of sub-use-cases like fraud detection, like cyber security, like risk modeling for economies….
(Tune in to listen to Awadallah’s fascinating business uses cases in-depth, including finding anomalies in lots of data. MasterCard, for example, has one of the largest data lakes—according to Awadallah—and stores the location of customers who are using its mobile app; this information helps the giant payment solutions company to more accurately predict whether a transaction is real or fraudulent in real-time.)