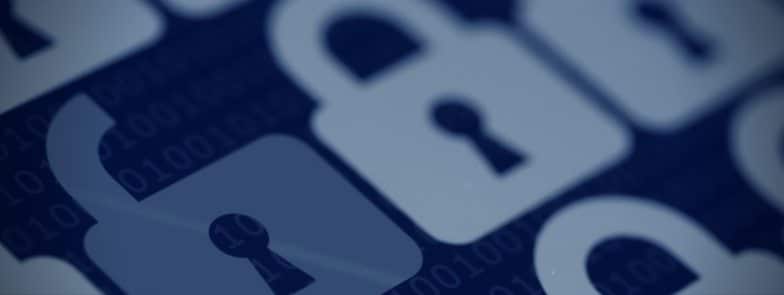
Security is a broad term, and in industry and government there are a myriad of “security” contexts on a variety of levels – from the individual to nation-wide. Artificial intelligence and machine learning technologies are being applied and developed across this spectrum.
While many of these technologies have the potential and have greatly benefited society (helping reduce credit card fraud, for example), the evolving social contexts and applications of these technologies often leave more questions than answers – in terms of rules, regulations and moral judgments – in their wake. Artificial intelligence and security were – in many ways – made for each other, and the modern approaches of machine learning seem to be arriving just in time to fill in the gaps of previous rule-based data security systems.
The purpose of this article is to shed light on current trends and applications, in industry and government, at the intersection of artificial intelligence and the security field. In addition to a spotlight on current uses (by no means inclusive), we also touch on up-and-coming applications and room for innovation (triggered by evolving needs of individuals and the larger population).
We’ve referenced several of our interviews with researchers and practitioners in this space, and their insights and experiences are responsible for some of the applications explored in this article. An overarching theme in their varied responses points to an important implication – using artificial intelligence to stay at least one step ahead of attackers, errors, and system failures. It’s important to emphasize that as threats and social contexts evolve, so too will the technology need to adapt – as well as the rules and regulations that govern the use of such technologies.
This article is broken down into three sections:
- Real-world use cases of artificial intelligence paired with security applications
- Potential future applications
- Basic glossary of artificial intelligence and security terms
The potential future applications is meant to spark ideas about some of the directions in which AI technologies are headed, and also illuminate a handful of key obstacles and challenges that need to be reconciled before the technology can begin to reach its full potential.
Artificial Intelligence and Security Applications – Real-World Examples
1 – Cyber Attacks (Defense Against Hackers) and Software Errors/Failures
The software that powers our computers and smart devices is subject to error in code, as well as security vulnerabilities that can be exploited by human hackers. Potential ramifications are on a grand scale, and range from the safety of an individual to the level of a nation or a region. Dr. Roman V. Yampolskiy, an associate professor at the Speed School of Engineering at University of Louisville and founder and director of the Cyber Security Lab, is concerned not just with human hackers, but with the ways in which AI itself may turn against our systems. “We’re starting to see very intelligent computer viruses, capable of modifying drone code, changing their behavior, penetrating targets,” says Roman.
The need for systems that can search out and repair these errors and vulnerabilities, as well as defend against incoming attacks, has grown out of this urgency, with many projects and eventual companies getting their start in research and/or being funded by the military (i.e. DARPA) and research universities.
ForAllSecure, a startup based in Pittsburgh and launched out of years of research at Carnegie Mellon, created the winning security bot in DARPA’s most recent 2016 Cyber Grand Challenge . AEG (automatic exploit generation) is the “first end-to-end system for fully automatic exploit generation,” according to the CMU team’s own description of its AI named ‘Mayhem’. Developed for off-the-shelf as well as enterprise software being increasingly used in our smart devices and appliances, AEG can find and determine whether the bug is exploitable. Bugs are errors in software that can cause unexpected results or behavior or potentials for security breaches.
If found, the bot autonomously produces a “working control flow hijack exploit string” i.e. secures vulnerabilities. Practical AEG has important applications for defense. For example, automated signature generation algorithms take as input a set of exploits, and output an intrusion detection system (IDS) signature (aka an input filter) that recognizes subsequent exploits and exploit variants.
Historically, signature-based solutions seem able to only get us so far in anticipating cyber security attacks. “The variety of (cyber) attacks, tens of thousands of different variants every day…that’s where the rules-based, the signature-based systems are a problem right now…we’re (cyber security defense) going to move to prescriptive analytics, where machines will do detection and interaction without human intervention,” Igor Baikalov, chief scientist at Securonix.
On the predictive side, MIT’s Computer Science and Artificial Intelligence Laboratory (CSAIL) and machine-learning startup PatternEx recently developed an artificial intelligence platform called AI2 that they claim predicts cyber-attacks significantly better than existing systems by continuously incorporating input from human experts. The technology is leveraged by a continuous loop of feedback between the human analyst and AI system, called Active Contextual Modeling, and is able to learn in real-time. In a peer-reviewed paper submitted to IEEE, PatternEx researchers compared a purely machine learning-based solution to the PatternEx solution and found that their algorithmic system increased attack detection rate by a factor of 10 over machine learning-only solutions.
2 – Security & Crime Prevention
New York Police Department’s CompStat (Computer Statistics) may be called an early form of “artificial intelligence”. First implemented in 1995, it is a systematic approach that includes philosophy and organizational management, but depends on underlying software tools. In essence, it was the first tool used for “predictive policing”, and has since spread to many police stations nationwide.
Predictive analytics and other AI-powered crime analysis tools have made significant strides since those “pioneering” times. California-based Armorway (recently reorganized as Avata Intelligence after diversifying its applications into healthcare and other arenas) has been using AI with game theory to predict when terrorists or other threats will strike a target. The Coast Guard uses the Armorway software for port security in New York, Boston and Los Angeles, drawing on data sources that includes passenger load numbers to traffic changes, and creating a schedule that makes it difficult for a terrorist to predict when there will be increased police presence.
3 – Privacy Protection
During its developer’s conference in June, Apple made an unexpected announcement in its pursuit of differential privacy methods for continuing to ensure customer privacy (a hallmark of Apple), but also with an eye on the value of using data to provide a customized user experience. Differential privacy has been written about for some years, but it’s a relatively new approach with mixed feedback as to its scalability.
A recent National Academies study (2015) reached the conclusion that there are not (yet) technological alternatives to bulk collection and analysis of civilian metadata. Differential privacy offers a way to maintain private data on a network, while providing targeted “provable assurances” to the protected subpopulation and using algorithms to investigated the targeted population. This type of solution can be used in trying to find patterns or indications of terrorists in a civilian population, find infected citizens within a larger healthy population, amongst other scenarios.
In a Big Data and Privacy Report to the President in 2014 (which is not longer online as of our September 2017 update of this article), his Council of Advisors on Security and Technology stated, “Assurances about privacy are much more precarious. Since not‐yet‐invented applications will have access to not‐yet‐imagined new sources of data, as well as to not‐yet‐discovered powerful algorithms, it’s much harder to provide, today, technological safeguards against a new route to violation of privacy tomorrow. Security deals with tomorrow’s threats against today’s platforms…But privacy deals with tomorrow’s threats against tomorrow’s platforms, since those “platforms” comprise not just hardware and software, but also new kinds of data and new algorithms.”
The issue of data mining and privacy are particularly complex, essentially because the machine-learning and data-mining technologies posting the threats are oblivious to the consequences of exploitation or trespassing on personal privacy laws. Good policy is key in the long-term, as machine learning software evolves and data is accidentally stumbled upon or intentionally mined by analysts across industries.
Potential Future Applications in Industry and for Consumers
1 – IoT Systems Security
Earlier this year, we had a chance to talk with AT&T about some of the ways in which they’re using artificial intelligence to transform their services. The ability to predict and prevent is “what’s exciting about machine learning and AI, it’s not about something happening right now; when something happens, or happened, it’s too late…it’s exciting to create an autonomous and intelligent network where we foresee and predict these events from happening before they become disasters,” said Mazin Gilbert, Assistant Vice President of Intelligent Services Research at AT&T Labs.
AT&T is experimenting with how to use and scale predictive services across their data centers. For example, the communications giant has implemented large-scale ML capabilities that allow them to pull data from contacts, chat and voice operations; process data to make predictions in near real-time; and provide that intelligence to managers and supervisors. Managers and supervisors can then look and monitor to identify anomalies, asking important questions along the way – were my customers happy or not? If I put them on hold, did that make them unhappy? Did my agent solve their problem the first time?
Using ML to determine customer sentiment in real time (for example, why they called, will they call again, etc.) and customizing service or taking other necessary measures is one of a large number of predictive capabilities that will likely be implemented at scale across industries in the future. Another area in which AT&T has been experimenting with predictive analytics is in the maintenance of their fleet. If a van breaks down and a customer ends up unhappy about the delay, the company faces two losses. Wouldn’t it be better if a connected vehicle could monitor and analyze its performance and bring itself into the shop in anticipation of a necessary repair or malfunction?
The Internet of Things (IoT) is enabling cost-efficient implementation of condition-based maintenance for a number of complex assets, with ML playing a current driving role in the analysis of incoming data. A Finnish energy company, for example, was having critical turbine failure in energy industry, used IoT and ML to get to root of the underlying problem ( it turned out to be oxygen feed optimization during the production process), and has had no major issues since.
With solutions rolling out like IBM’s Watson for IoT, network wide analytics platforms that are able to predict in real-time failures and recommend maintenance based on an asset condition, whether it be related to a property, vehicle, or other network component, seems poised to become industry standard over the next decade.
2 – Security and Crime Prevention
AI is already “a thing” in security and crime prevention – as described in current applications – continue to move the industry forward, as outlined by Stanford in the 2016 report of its One-Hundred Year Study on Artificial Intelligence (AI100).
The Transportation Security Administration (TSA) is pursuing a project to overhaul and reformulate airport security nationwide. Called DARMS, short for Dynamic Aviation Risk Management Solution, the smart system will integrate information across the aviation sector to tailor a personalized security profile for each person, on a per-flight basis. A smart tunnel will checks people’s security while they walk through it, eliminating the need for inefficient security lines. DARMS is intended to be introduced into US airports in four to 10 years.
The European Union, through its Horizon 2020 program, currently supports AI-simulated security training efforts through projects like LawTrain, where the next steps are to move from simulation to real investigations that utilize such tools in machine-human collaborations.
Many other cities have already added drones for surveillance of potential criminal activity, and while the debate about ethics and rules and regulations will stay strong, it seems likely that smart drones able to detect crimes-in-the-making and alert authorities will be used in the future to reinforce security at major crossways such as ports, airports, industrial facilities, and others.
It’s worth emphasizing that AI prediction tools introduced with the proper precaution sand regulations in place have the potential to lessen or remove human bias as opposed to substantiating its effects.
3 – Analysis of Consumer Information (A Focus on Health Data)
“Digital disease detection” and “infodemiology” typically involve the collection of large-scale health data to inform public health and policy. These analyses of anonymized data – whether publicly disclosed or privately held – are capable of yielding valuable results and insights on public health questions across populations. The federal HIPAA regulations limits the release of personal healthcare records, information that could be useful in many large-scale aggregate studies. The reasons for limiting this information are valid.
Some methods and models might be aimed at making inferences about unique individuals that could drive actions, such as alerting or providing digital nudges on social media apps or other means, to improve individual or public health outcomes; this idea, while done with ‘good’ intent, can undermine a basic goal of many privacy laws—to allow individuals to control who knows what about them.
As suggested by a Microsoft research team, even outside of healthcare records there is room today – thanks to big data – to infer health conditions and risks from non-medical information, whether through informational or social contexts. This provides ample opportunity for reflection on directions in balancing innovation and regulation.
Although much data is already online, and machine learning has the potential capability to predict future health status from health-related and other forms of information, Microsoft’s team emphasizes the point that we do not currently have the laws, customs, culture, or mechanisms to enable society to benefit from these types of innovations currently. Perhaps the most important innovation in this arena is developing good policy i.e. how to use data and audit-able and accountable systems, rather than the machine learning technologies used to collect and analyze this information.
Brief Glossary of Basic AI and Security Terms
- Cyber Security: Involves protecting information and systems from major cyber threats, such as cyber terrorism, cyber warfare, and cyber espionage.
- Intrusion Detection System (IDS): A type of security software designed to automatically alert administrators when someone or something is trying to compromise information system through malicious activities or through security policy violations.
- Game Theory: The science of strategy, or at least the optimal decision-making of independent and competing actors in a strategic setting.
- Differential Privacy: Approach that allows data scientists to extract insights from a database while guaranteeing that no individual can be identified i.e. it guarantees that the answer one gets from any query on a database is not perceptibly different if any one individual is excluded from the database (privacy for opt outs). This latter task is accomplished this guarantee by adding noise to any answer returned by the database.
- Cryptography: A method of storing and transmitting data in a particular form so that only those for whom it is intended can read and process it.
Related Emerj Data Security Interviews
If you enjoyed this article about artificial intelligence and security, you might also enjoy our previous articles about artificial intelligence in marketing, and artificial intelligence in IoT.