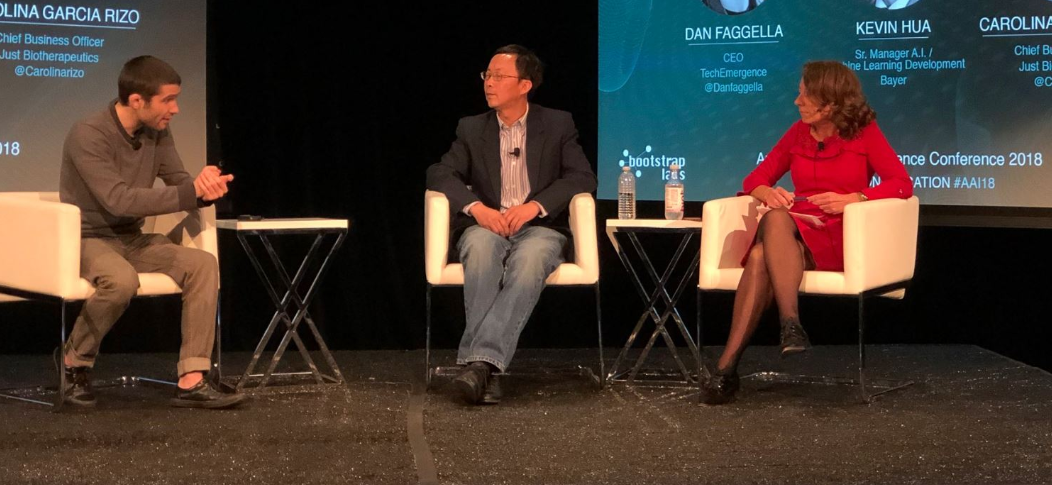
This article is based on a panel discussion facilitated by Emerj (Techemergence) CEO Dan Faggella on the state of AI in the healthcare industry. The panelists were Just Biotherapeutics Chief Business Officer Carolina Garcia Rizo (representing healthcare startups) and Senior Manager for A.I./Machine Learning at Bayer Kevin Hua (representing big pharma). This session was part of the Applied Artificial Intelligence Conference by Bootstraps Labs held in San Francisco on April 12, 2018. The video of the panel is provided below:
The healthcare industry has for a while now been a bit of a hotspot for artificial intelligence. It is particularly primed to benefit from the potentialities of AI because it deals with huge amounts of data on a daily basis. Patient information, medical histories, diagnostic results, hospital billing, and clinical studies are just a few of the data sources available in healthcare.
This huge pool of data can train AI and machine learning to detect patterns and come up with predictions, recommendations, or conclusion, whatever the case may be. As it is now, much of that does not go through any type of processing at all.
We surveyed more than 50 executives from healthcare companies leveraging AI shows that the AI in healthcare space is still wide open. Some believe that by 2025 AI will be “ubiquitous” in the healthcare space. The demographics of the sample are detailed in the graphic below:
(See the full “ML in Healthcare Executive Consensus” report here)
Most people understand that AI in healthcare is mostly in the pilot phase. However, what most people don’t know is that for any given list of a hundred AI healthcare companies, only 1 in 3 has the requisite academic or marquee company background in AI to even do something with the science.
Of those with some promise, only about 1 in 4 have some kind of meaningful traction with a pilot. These companies have successfully collaborated with big pharma or a hospital to confirm their legitimacy. Overall, only about 1 in 10 AI in healthcare companies have any degree of actual traction in the space. It looks like there are more legitimate AI and healthcare companies than there really are. The situation may be different in 2 or 3 years, but that is where AI in healthcare is today.
Difficulties in Healthcare AI Adoption
The industry is receptive to new ways to improve diagnostics, patient care, and financial efficiencies. However, these AI healthcare companies contend with some significant challenges with regards to widespread AI adoption in the healthcare.
Case Study Conundrum
A major friction point with widespread healthcare adoption of AI is the need to convince industry stakeholders about the positive return on investments in Ai and machine learning. They need to have a better understanding of the value proposition to make a decision to buy into it.
The best way to illustrate ROI is to present as many case studies as possible, which presents a chicken-and-egg type of situation. AI companies need more case studies to convince decision-makers in the healthcare industry to get on board, but they need more healthcare companies to get on board to produce case studies.
Below is a graphic displaying our research into what healthcare AI executives feel are the difficulties with selling into the healthcare industry:
(See the full “ML in Healthcare Executive Consensus” report here)
Black Box Issue
Another major friction point is the lack of understanding of the deep technical workings in machine learning. In most industries, the inability to understand how a system works is not a deal breaker as long as it brings results.
In healthcare, however, stakeholders need to know how a system comes up with a diagnosis or recommendation because it will be the basis for making important decisions about patients.
Stakeholder Complexities
One other issue in the adoption of AI/ML in healthcare is complex stakeholder relationships, especially in the hospital setting. In a pharma setting, it is only necessary to convince the upper echelon of the company about the ROI of the system to close the deal. In a hospital, however, it only starts with the hospital executives.
It is necessary to educate the doctors and other healthcare professionals about it, and train them to use it. It is also necessary to explain to patients how they can benefit from it. At any point in the chain, a stakeholder is resistant makes it all the more difficult for adoption.
Current Trends
Despite these sources of friction, AI companies persist in developing systems for various domains in healthcare. According to the survey, these companies focus on diagnostic SaaS (35%), operational efficiencies for hospitals (24%), consumer apps for behavioral adherence (29%), and physical products such as medical devices (11%). The full graphic is below:
(See the full “ML in Healthcare Executive Consensus” report here)
Diagnostic AI is particularly popular with AI in healthcare companies, so much so that it will become commonplace in a few years. This is a good thing as it will make AI easier to accept in other domains, such as back-office efficiency software is doing right now. However, AI in healthcare companies will have to find another way to differentiate themselves from their competitors.
A panel discussion with two top executives from pharma companies at the Applied Artificial Intelligence Conference provided some insights into possible differentiators in this space. Spoke with Just Biopharmaceuticals Chief Business Officer Carolina Garcia Rizo and Bayer Senior Manager for A.I./Machine Learning Kevin Hua, and asked them a few key questions.
Question 1: How and where has AI affected the work of subject matter experts in your space?
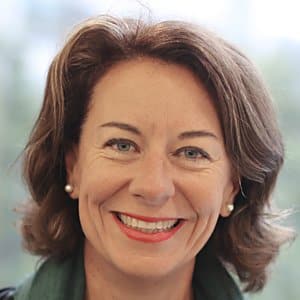
Garcia Rizo: So, in our case, it’s actually freeing up lots of time. So when there were some comments before on whether AI is reducing the possibilities of work for us, it’s actually the opposite because it opens up much more possibilities. People can focus now on what they do best. The repetitive work is taken by AI.
Hua: In our case, we work closely with our domain experts. We have the best domain experts in the industry. But we figured out we still have room to improve because the amount of data is huge.
So we need to combine domain expert knowledge with machine learning models to get a better model.
Question 2: How is AI changing the workflow or the skill set required for existing subject matter experts?
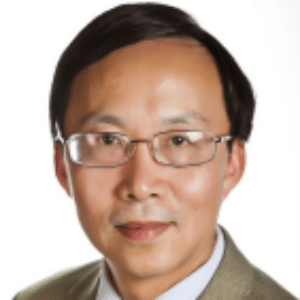
Hua: There will be some change in management process because that will somehow disrupt the existing way of doing business. So our plan was to have domain experts continue to do their own daily work, and then we use machine learning models to help them to justify their decisions.
If there’s a discrepancy, our models can interpret it and explain why there is a discrepancy so that we can determine which prediction to use in the end.
Question 3: How are your end users responding to the innovations you are trying to push forth in AI? How does it work in your experience?
Garcia Rizo: For us, you have to consider that this industry is very regulated, right? So the FDA has a very strong opinion. One of the biggest questions that we get is, “what about the FDA?”
We are working very closely with the FDA. We have a GMP manufacturing facility phase 1, phase 2 in the company, and we have worked very closely with the FDA. We are teaching them and collaborating with them, which is a really good collaboration to have with the FDA. That said, I think that there are all kinds of customers that are excited because all of the sudden they can say, “Well, I’m collaborating with this company that is the leading technology because it has AI.”
Hua: I think it’s a common reaction from everyone. If you don’t understand the theory, you tend to be skeptical. Our customers are internal, and we do have some people who are more skeptical. Some are more open to new technology. So, I think we have the smartest people at Bayer because if you look at life science companies we have the highest percentage of PhDs in industry. So if you explain the technology, they can easily understand it. Also when we build models we should make it interpretable and we should make it something that can be validated. So those are the types of measures we take on how to get people to accept our knowledge.
Question 4: When do you see that government agencies will be onboard with AI in healthcare?
Garcia Rizo: I think that the FDA is really learning pretty fast. I’m really confident that we are living in a time where finally we have put the patient in the center, which is where it has to be. And I think that everyone is really working together towards this solution for the patient that is really trying to have better health and to try to cure the disease and hopefully to use machine learning to predict those diseases so they’re not a patient anymore, right?
Question 5: What advice do you have people in life sciences to make that transition to AI in healthcare easier?
Garcia Rizo: Never underestimate the power of education for your clients. I mean think about healthcare. There are all different stakeholders, and each one is taking their own way, driving their own forces. So education is key. Also, show the market that you have positive case studies, and display what you have you done through those case studies. What have you achieved, and how and why is it important? What is the benefit for the patient? I think I will not emphasize that enough. Education is really important when you are doing some innovative, cutting-edge technology.
Hua: So in our case, they actually come from the high-level management and we believe in technology and we have started a few new analytics groups. We are hiring people and it’s just up to us to show them how these technologies are going to work, how it’s going to help us improve our daily business.
Header image credit: BootstrapLabs