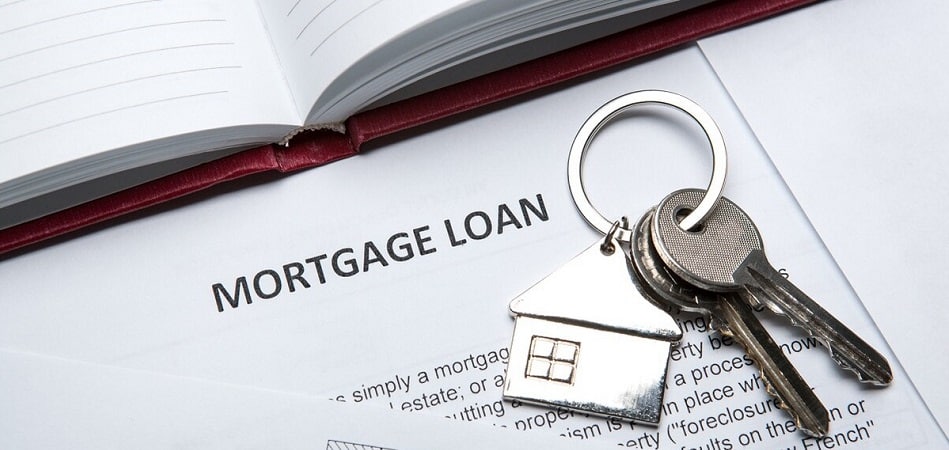
Many large banks and financial institutions are beginning to digitize parts of their business processes to prepare for future initiatives in automation and machine learning. This is particularly true with loan processing. These functions could become faster and more accurate if they use digitized data that is more easily accessible than paper documents.
In this article, we will explain how to effectively use AI to digitize loan processing. Our guide covers the following topics:
- The Problem of Paper Documents: For example, maintaining regulatory compliance in keeping customer data private, yet accessible to those who need to access it for legal reasons.
- How AI Could Facilitate the Loan Processing: Using methods such as document digitization and text mining to digitize the loan processing workflow.
- Challenges with Adopting Document Digitization Solutions: Including the need to tag digitized loan documents with metadata.
We begin our guide on how to use AI to digitize loan processing with an overview of the difficulties paper documents can cause in this area:
The Paper Problem in Loan Processing
Many banks and financial institutions still use paper documents for loans and other core business processes. This can cause problems that slow down loan processing within different business areas. These companies face the following challenges in continuing to use paper documents:
- Maintaining Regulatory Compliance
- Slow Time to Loan Origination
- Disorganized Underwriting of Loan and Mortgage Documents
In order to stay compliant with government regulations such as GDPR or the California Consumer Privacy Act, companies need to keep all of their customer data accessible and traceable. If a customer asked a bank to remove all information it has on them, the bank might have difficulty fulfilling that request and proving it.
In most cases, the company would not be able to track down every physical document containing the customer’s information because they had not been keeping track of each individual page for years. This puts the bank at risk of noncompliance, which can result in millions of dollars worth of fines.
Paper documents can also slow down time to loan origination. Once a customer qualifies for a loan, they must provide extensive documentation to prove their income. This includes the purchase and sale contract, W-2 forms, and bank statements. If they are refinancing an existing mortgage, the customer will need to provide mortgage statements as well.
Lenders that have to work with paper documents will take longer to originate loans because they spend more time flipping through pages and manually referencing each document. If one or more of these documents are misplaced, it may take a full business day or more to relocate it before the loan can be completed.
How to Facilitate Loan Processing Functions with AI
Each of the previously discussed business processes requires some form of searching through enterprise data – a task that requires data to be processed and harmonized beforehand (which often takes much longer than business leaders presume).
AI solutions could facilitate the search process and speed up underwriting and loan origination through digitizing all physical documents and subsequently making the company’s intranet more accessible and easy to use.
Two AI capabilities, in particular, could make such improvements possible:
- Document Digitization
- Text Mining and Automated Metadata Tagging
Digitizing Customer Data Stored in Paper Documents
AI document digitization applications require machine vision technology equipped to a camera that can produce high-resolution images of paper documents to accurately convey the information within them. Additionally, optical character recognition (OCR) can also reproduce the written word in a digital format. The new digital version of a document can then be saved to the company’s database.
Banks could benefit from using machine vision to digitize documents necessary for loans and underwriting such as:
- Purchase and sale contracts
- Paper or scanned PDF applications for mortgage loans.
- Physical and scanned PDF checks
- Bank statements for customers proving their banking and credit history
Wealth managers may see a rise in productivity if all of these types of documents could be digitized. This is because all of the data necessary to qualify and onboard new loan customers would be accessible in the bank’s intranet. They would not need to leave their office or search through filing cabinets to reference the data required to make important business decisions such as an applicant’s credit history. That said, the newly digitized data would still need to be easily searchable for this method to be as efficient as possible.
Organizing and Tagging Data for Accessibility and Traceability
AI applications for text mining could allow companies to search through their intranet to access legacy customer data and information from newly digitized documents. This type of application usually leverages natural language processing (NLP) technology because it can make sense of text within a document and correlate it to search keywords.
Once integrated into a bank or financial institution’s database, a text mining software could provide accurate search across all document types.
Text mining applications could also correlate search keywords with metadata attached to the documents within a database. For example, consider a wealth manager who is looking specifically for older loan agreements from specific customers. The software could eliminate all documents but loan agreements with those customers within a designated timeframe.
Challenges with Adopting Text Mining and Metadata Tagging Applications
Text mining applications may require all documents within a database to be tagged with metadata in order to function properly. Document digitization and enterprise search applications will require a company to renovate their data infrastructure to be compatible with the software. That said, this can be a time-consuming process companies should consider before moving forward with any AI initiative.
Some machine learning solutions can automatically tag documents with metadata, but the client company will still need to set the parameters for that metadata. The algorithm behind the software correlates meta-information over time such as dates and identification numbers, and could apply it to future searches.
Header Image Credit: Military Times