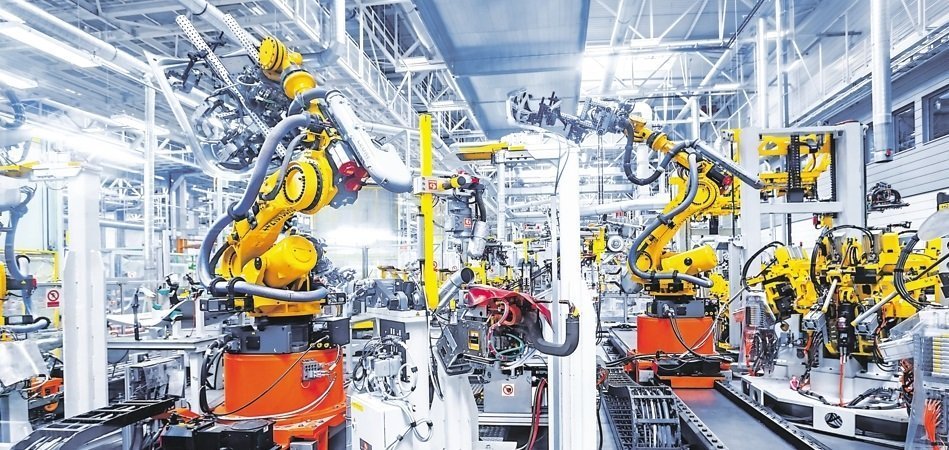
Accenture forecast the Industrial Internet of Things could contribute $10 trillion to the global economy by 2030. The report also suggested that sensors, material tracking mechanisms, 3D printing, automated product design, robotics, and wearables could help manufacturers reduce costs and increase productivity. Predictive asset maintenance could potentially reduce equipment and machinery maintenance costs by up to 30% and result in up to 70% fewer breakdowns.
As of now, numerous companies claim to assist industrial equipment operators and engineers in aspects of their roles from operating and maintaining machinery to improving factory processes.
We researched the space to better understand where AI comes into play in industrial automation and to answer the following questions:
- What types of AI applications are currently in use for industrial automation?
- What tangible results has AI driven for industrial automation?
- Are there any common trends among these innovation efforts? How could these trends affect the future of industrial automation?
This report covers companies offering software across two technologies:
- Industrial Internet of Things (IIoT): the network that connects, collects, communicates, and monitors devices to enhance industrial processes
- Collaborative robots, or cobots: robots designed to work with humans
This article intends to provide business leaders in the manufacturing space with an idea of what they can currently expect from AI in their industry. We hope that this article allows business leaders in manufacturing to garner insights they can confidently relay to their executive teams so they can make informed decisions when thinking about AI adoption. At the very least, this article intends to reduce the time business leaders in manufacturing spend researching AI companies they may want to work with to find an industrial automation solution.
Industrial Internet of Things
Siemens
Siemens is a Munich, Germany-based company that offers a software called MindSphere, a cloud-based operating system that enables machines and equipment inside a plant to collect data. Siemens claims this can help manufacturers monitor the condition of their industrial assets using machine learning-based analytics.
Siemens claims that sensors gather data from various machines and upload them to the company’s database in the cloud. This data is then run through MindSphere’s machine learning algorithm to inform analytics on a dashboard.
For example, production in a battery factory running on the MindSphere platform might be set to produce 100,000 batteries a day, but sensor data might inform analytics that shows that production has dipped in the past two days. This information is presented in the form of visual charts on a facility maintenance manager’s computer screen. MindSphere may further show which specific machine is underperforming.
MindSphere may also run diagnostics on the underperforming machine. Once the machine learning model behind it finds the problem, the MindSphere may inform machine maintenance operators about the problem and recommend to either fix or retire the machine.
Below is a short 3-minute video giving an overview of Siemen’s technology:
Siemens reports that MindSphere can be used in a variety of industrial settings, including the aerospace, automotive, and energy sectors.
Siemens claims to have helped Heathrow Airport improve their baggage handling system with sensors and MindSphere by reducing system downtimes and interruptions. About 76 million people passed through Heathrow in 2016, most of whom had luggage, according to the company.
Heathrow was looking to transition maintenance on the baggage handling system from scheduled maintenance to as-needed maintenance. To do this, Siemens technicians installed sensors on important components of the system to monitor it. The sensors regularly collect data on factors such as acoustics, which might inform a problem with the system, and transmits that data to the MindSphere platform.
The case study did not provide the results of integrating MindSphere, but Grant Waring, asset manager for Heathrow’s baggage operations said, “Over 80% of passengers are already rating Heathrow as very good or excellent. This positive assessment is both a confirmation and incentive for us. With Siemens as one of our innovation partners, we want to maintain and further improve our high standards.”
Siemens also lists Atos, Accenture, Microsoft, Amazon Web Services, Evosoft, BluVision, SAP as some of their clients and partners.
Roland Busch is the CTO and Managing Board Member at Siemens. He holds a PhD in Physics from the University of Erlangen-Nuremberg.
IBM Watson
IBM offers IBM Watson, which it claims can help mining companies conduct subsurface analytics that could potentially reduce drilling costs, improve predictions about mineral explorations, and accelerate geological insights using image recognition and machine learning.
IBM claims the application enables geologists to conduct subsurface analytics around geoscience data interpretation, reservoir exploration, and mineral exploration. To do this, users may enter a query into the platform’s search interface. The search engine’s algorithms will then run the keywords through the geological data repository, which contains structured and unstructured data from a variety of sources, such as the drill database, block models, geochemistry, geological shapefiles, core photos, and X-Ray fluorescence data. The system then returns predictions about the mineral content as a graph.
We could not find a video demonstrating how IBM for Mining works.
IBM claims to have helped GoldCorp increase the productivity of its existing exploration and production operations by creating a machine learning model to predict gold mineralization within a specific location in the mine. The algorithms searched the repository for various geological information which, if taken together, resulted in a well-informed prediction.
This information included lithology, veining, mineralization, alterations, and structure information, such as faults and folds. The information was collected from multiple sources, such as drill-hole data, chip data, maps, and geological models in and around the target location. The predicted gold content in that location came in a graphical format.
According to GoldCorp, using Watson has helped the company consolidate its 140 block models, drill logs, and other forms of unstructured data into one database. Previously, the company could load only two or three block models at a time.
IBM also lists Sandvik and Valenje Coal Mine as some of its mining clients.
Romeo Kienzler is the Global Chief Data Scientist, Deep Learning/AI Engineer at Watson IoT, where he has served since 2008 in various roles. He holds an MS in information systems, statistics, and bioinformatics from ETH Zurich. Earlier in his career, Kienzler served as a software engineer at CSS Versicherung AG.
General Electric
General Electric offers Brilliant Factory, a suite of hardware assets, software, and consultation services for upgrading factories to industrial IoT. GE claims this can help manufacturers enhance the performance of their factories using predictive analytics.
GE claims that using the data-driven Brilliant Factory suite could reduce costs, improve the quality of products and services, and expedite the production process. The platform enables businesses to monitor the health and performance of factory machinery and equipment and manage the production process.
This is done by connecting machinery and equipment to a single network, implementing sensors on each machine, and collecting the structured and unstructured data in a database where it is stored and organized. The company claims the software works with data from all types of equipment from all different industries.
Brilliant Factory’s machine learning model, which is reportedly trained on data from GE’s history of manufactured assets and services, regularly runs diagnostics on factory equipment to determine the condition of the machinery or quality of the products they produce. If the algorithms recognize any deviation from the benchmarks stored in its database, the operators will be alerted through in a graph presented on a dashboard.
For instance, at a ramen noodle factory, sensors connected to the vat that mixes the ingredients might determine that too much flour is being used. This might be determined by the weight of the noodles or the time it takes to cook them, which the machine learning algorithms compare with the standards recorded in its database. The operators will be alerted to improve the recipe by reducing the amount of flour. The added benefit of this reduction in raw materials is savings on the part of the business.
Below is a short 2-minute video demonstrating how Brilliant Factory works:
GE claims to have helped Premier Foods optimize the performance of its factory, with the help of GE’s partner Factora. Premier’s legacy processes and systems did not have sensors to monitor machines. Processes and plant performance were inconsistent. The company needed to identify the root causes of issues and improve key processes such as water activity, line speed, roller speed, and heat temperatures.
Using iFIX, which is a part of the Brilliant Factory suite, Factora connected Premier’s machines to collect data, model the processes, and analyzed the results, and create a plan of improvement.
For instance, the solution that Factora recommended helped Premier realize potential sales with a cake snack line. By stabilizing the process, Premier was able to use less raw materials and reduce the calorie count of each snack, an appealing selling point to some customers. Aside from raw material savings, Premier now can track its key processes. This enables them to take action to ensure consistent quality and control and optimize production yield.
GE also lists Subaru, Lek Pharmaceuticals, Spomlek, Cascades Tissue Group, Vale Fertilizantes, and Toray Plastics as some of their past clients.
Collaborative Robots
Rethink Robots
Rethink Robot offers Sawyer, a collaborative robot (cobot) that runs on software called Intera. The company claims that Sawyer can help manufacturers achieve better productivity and higher-quality products using the machine vision.
Rethink Robots claims that Sawyer is embedded with the Cognex Vision System, which enables it to detect objects or inspect parts with object detection technology. This vision system captures images of items to be handled and transmitted to the Robot Positioning System (RPS), an application that looks for changes in the robots’ environment and enables the user to adjust its settings. Users can also integrate external cameras into the cobot.
For example, relocating Sawyer from one part of an assembly line to another means the vision system needs to be adjusted so Sawyer can detect the new locations of items it will pick up, place, and hold in the new work area. Another example is when human operators accidentally bump into tables, conveyor belts, or the cobot itself, the physical location of items to pick or place will no longer match what is saved in the vision system. These slight changes can cause the cobot to make errors in picking up and placing objects. When this happens, the RPS must be readjusted to allow the vision system to detect the new location of the objects.
A tablet, which serves as Sawyer’s “face,” also serves as the interface through which the robot operator makes adjustments.
The Intera software allows operators to train the cobot by moving its arm and demonstrating the movement of pick up, place, and hold. Sawyer can also perform functions such as overseeing a machine while it operates, plastic injection and blow molding, testing, and quality inspection, and tending to the metal stamping or metal pressing process. These are processes usually done in automotive supply chain, metal fabrication, general manufacturing, packaging, and plastics factory automation.
Below is a short 2-minute video demonstrating how the Sawyer cobot works:
Rethink Robotics claims to have helped Assa Abloy automate its production lines that were reliant on manual labor. The company turned to Rethink Robots’ distributor, Active 8 Robots, for help. Assa Abloy’s site was evaluated, and two Sawyer cobots were initially deployed on site. The case study adds that Assa Abloy now has deployed Sawyer cobots in their plants across the globe. According to the case study, Sawyer helped automate the manual assembly and packing of about 18,000 pairs of hinges a day.
Rethink Robots also lists Acorn Sales Company, Rapid Line, Tuthill, Cornell Dubilier, DHL, Moduform, Templasco, and Trellidor as some of its past clients.
Established in 2008, the company has raised $149 million in funding from Bezos Expeditions, Charles River Ventures, Highland Capital Partners, Sigma Partners, Draper Fisher Jurvetson, and Two Sigma Ventures.
Rodney Brooks has been the CTO and Chairman at Rethink Robots since 2008. He holds a PhD in Computer Science from the Massachusetts Institute of Technology. Previously, Brooks served as CTO and Chairman at iRobot for 21 years.
Takeaways for Business Leaders in Manufacturing
The global companies covered in this report, IBM, Siemens, and General Electric, are the prominent players in industrial AI by way of Industrial Internet of Things.
IIoT is combined with artificial intelligence and big data to offer clients deeper insight into the data they collect through IoT sensors by way of predictive analytics. This can help manufacturers make better business decisions.
Some of the companies covered in this report offer predictive and preventive capabilities that help adopters know if their equipment or machinery needs to be repaired before the problem worsens. In the case of IBM Watson, the predictive feature is applied in mining, helping in mineral exploration.
In robotics, vision systems continue to drive what is now known as collaborative robots, or cobots. While cobots are meant to collaborate with humans in terms of supporting or relieving the human operator of repetitive tasks, it is not apparent at this point if the cobots are capable of automating more intellectual tasks or if they can be connected to an IIoT network.
The IIoT makes data available and accessible, but it is only as good as the humans that use it. The companies listed in this report state that factory operators or engineers will need skills to manage the technology and to interpret the data. The companies do not say if data scientists are needed.
The covered companies also did not clarify the integration times, but they explain that sensors can be attached to the already-existing machinery and equipment in factories.
Header Image Credit: Les Echos