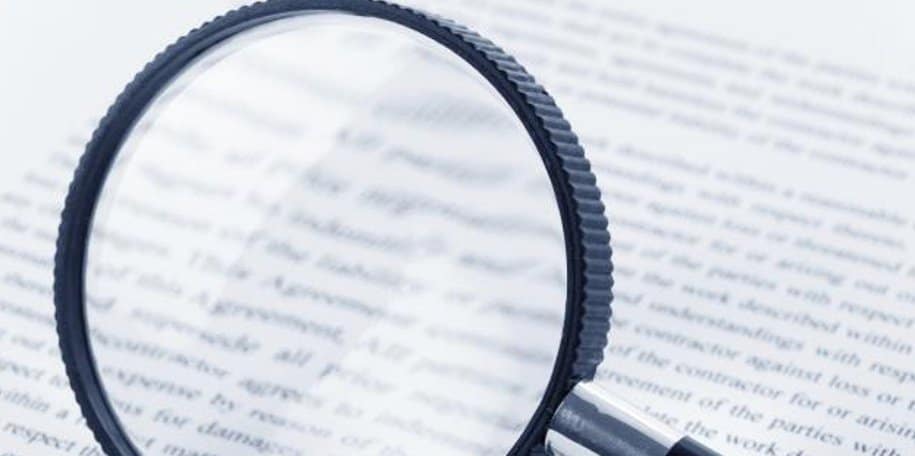
Episode Summary: In this episode of the AI in Industry podcast, we interview Grant Ingersoll, CTO of Lucidworks, about AI developments in enterprise search, and the common challenges of AI adoption in enterprise.
Ingersoll talks about how companies have massive amounts of siloed data, making it difficult to find within enterprise systems. We hope businesses might take away from this interview what is required and what is involved in building search applications to make corporate data more accessible and structured.
Ingersoll will also discuss how data strategies are likely to evolve in the years ahead, and how data scientists and data experts need to collaborate in order to build an enterprise search application.
Guest: Grant Ingersoll, CTO of Lucidworks
Expertise: Search, question-answering engine, natural language processing applications
Brief Recognition: Ingersoll holds an MS in Computer Science from Syracuse University. Previously, Ingersoll served as principal at Lucid Imagination and as a senior software engineer at Promergent. Earlier in his career, he was a senior systems engineer at MNIS – TextWise Labs. Ingersoll is the co-author of Taming Text, co-founder of Apache Mahout, and a long-standing committee member of Lucene and Solr open source projects.
Subscribe to our AI in Industry Podcast with your favorite podcast service:
Interview Highlights
(02:25) What are some of the troubles of people finding what they want in this endless vat of data in the enterprise?
Grant Ingersoll: At the most basic level, there is “where is my stuff? I wrote this document. How come I can’t find it?” For enterprise search, that’s really table stakes these days. Where it starts to get interesting is this “aha” moment once you’ve got enterprise search in place where you realize, “I’ve got all your data in this enterprise search engine, and I’ve got enterprise users interacting with it. What can I do to enable them to take action?
In our day-to-day lives in business, we’ve had this moment where “I’ve looked at the dashboards, I’ve seen all kinds of charts,” but there is always the question of “What do I do next?” I think this next generation of enterprise search engines, at the end of the day, is about how do you take that next best action.
Help me make that decision on sales, help me make on who we should market to. Because search is so pervasive, we want that across the board.
(04:00) People are getting into data lakes but it is tough to get things out. Is there some context on why that is tough and what could address the root?
GI: The first level is garbage in, garbage out. Just trying out a system like HD FAS or having a corporate strategy that is all IT-driven only gets you so far. I can start adding all these data sources into my data lake, but if there is no plan to migrate the applications that were built on those previous data systems to the new data lake, what difference does that make? People who are used to doing their jobs using the individual systems, they cannot all of a sudden move to the data lake. If it takes you a super long time to park those applications, it will never happen because I have to still do my job.
The next level is asking what is in there and how do I figure it out. People will say “I stored this document in here, where is it?” Most of these big data systems don’t go the last mile. They don’t get to the user. How do we help the user? It is not necessarily how to help IT have a bigger budget for their big data system. How do you get answers to the business so they can make their decisions?
(05:45) Do we want business, operating, bottom-line-oriented folks and those taking strategic actions to be in the room when the IT stuff is figured out?
GI: In many ways, it is yet another silo which was supposed to solve them in the first place. Ironically, it has been…I’ve never been one for huge meetings. But I think you are onto something in the sense that the big data movement has been marketed to IT as a panacea for all things wrong with the business, and it left out a lot of stakeholders, at least in the projects I’ve seen fail. The people who are getting value, to your point, they do bring in the business. They think about the last mile. They think about the application I’m going to deliver on top of this, not just how do I store data cheaply, or how do I store more data?
(07:15) What can AI permit in that circumstance where data can be cloudy? What can be done to answers that are more overt and more directly useful in business?
GI: First off, I like to be pragmatic but there is a lot of low-hanging fruit that doesn’t necessarily involve AI. I like to make sure that whenever we talk to customers, that we are grounded in reality and we have done the “easy things.” Let’s face it: if you weren’t capable of implementing something that has been around for 20 years and is well understood and you do AI, then it is going to be problematic.
The challenge for AI in the enterprise, especially employee-facing applications, is that they often fail to account for the user. I think that lack of user understanding applies to the AI space as well. The tricky part of AI in the enterprise is you don’t always have the massive user feedback loops like you do in consumer-facing, like what Google, Facebook or Amazon have. You don’t have a million clicks a day that say someone likes this piece of data more than that.
You have to know when and where to pick your battles. When to know when something like deep learning works versus something simpler like logistic regression, a supervised approach versus an unsupervised approach. I look at all of this like I have a pal to whom I can say “ give me a little clustering here,” “ give me some NLP (natural language processing) here, “give me some classification here,” “just give some counting over there,” and mash that all together and feed it into a search engine so I can surface it to users easier. That’s the way to be successful with AI in the enterprise.
(10:02) Are there examples of where one will need AI in business?
GI: The current AI movement unlocks some new data sources that I think the previous versions of AI were not that good at dealing with. Image, video and audio content, smarter NLP capabilities have unlocked AI capabilities that I get excited about because I used to demo that. The demo was always great but the reality of trying to implement it five to 10 years ago was not quite tractable, or tractable for one use case but it could never carry over. Whereas now, there is so much more investment that we can leverage in these techniques; the compute powers there, the data is there, such that we could start to leverage them more and more in the enterprise.
At the simplest level, we struggle with how do you organize your data better. How do we label it, how do we curate it? We talked about compliance earlier, how do we know who can see what, when and where. All of those things have AI applications, It’s just the simplest access questions of “ what do I need to do right now?” That is fundamentally a ranking in question that is going to look at pieces of data and say “you are going to have an interview with Grant. You have to know these three things to know about Grant before you do that interview.” An AI system can learn that.
One of our big bank customers is exactly like this, working with the next best action with their salespeople. Effectively, anything and everything that happens when a customer touches the bank ends up in a system that is driven by search with an AI component, such that one time, a wealth advisor in a big bank can say, “Grant, Dan just had a child. You should talk to him about a 529 plan. Or Dan was just on the site and wants to sell his shares of ACME company. You should talk to Dan.”
At the end of the day, these guys need to serve so many people. They need to know where to spend their time. That brings to me full circle about being human-centric with AI. How do I enable you to make better decisions? How do I enable you to serve more people with fewer or faster, better people?
That’s where that ranking function is a killer and AI feeds into that nicely
(13:35) We are seeing more and more AI applications in banking. There is interest in wealth management and enabling sales folks. We have a lot of moving parts, a lot of activity on site and that should be contextually available to a salesperson or should prompt them to do something? Is this a partially an enterprise search problem? Or is it pulling from various data sources to do decision support?
GI: The enterprise search moniker is a bit outdated. Some people call it search-driven analytics. I like to take a step back and ask how do we reframe how we think about data. I’m sure a huge chunk in your audience know SQL.
Classically, if you put data in excel, you can sort by a particular column. That’s been the notion of relational databases for a long time. A search engine at its core is not that different other than my sort function sorts by what is important. It is often a combination of different factors. That use case about next best action, they are taking in customer recent activity, new products that the bank has, when did you last talk to this person, when did you have life events. They are taking in all these and asking the search function to “rank these things for me,” “could you tell me what is the first most important thing or the second important?”
That is what a search engine was built for since day one. Except that most people think about it as putting in a keyword and getting back a bunch of text. The reality is that these modern search engines enable you to search text data, spatial data, numeric data, categorical data. By the way, you can plug in advanced ranking functions such as a Tensorflow or other deep learning library or something built in-house. You can mash together these things and say, “show me what is important; show me what to put at the top of my list.”
(16:15) We are going to have different proxies for what customer is a hot lead, useful action, or relevant notification in different circumstances. It sounds like there needs to be in-house smarts on what are those signals, probably in-house data understanding on what data could lead to those signals and where to build something of this kind.
GI: I like to deliver a product that gets people most of the way there. There is always this last bit of how do I build my business logic to the data I have such that I can deliver it to my users so that they can consume it. It is not as bespoke as you think. We happen to be up and running for these types of applications in weeks, not months or years. Sometimes it is as little as a few days.
If you are going to cross your data sources, logic needs to go into that system. There is no silver bullet so I won’t claim that there is.
(17:55) When you are talking about accessing data, you need more than IT people. When you are developing a strategy to extract and visualize [that data] to make that operational, we are going to need technology folks, but we also need a lot of subject matter expertise that will be important in that last mile.
GI: You’ve got it nicely summarized. The beauty is your business users are more technical than ever. Your technical users are more business savvy than ever. This is an emerging trend as well. By bringing those two people together, you will be in good shape. AI is the piece that glues together long-term learning about how those folks interact with their content.
Subscribe to our AI in Industry Podcast with your favorite podcast service:
Header Image Credit: Law Society of the Northern Provinces