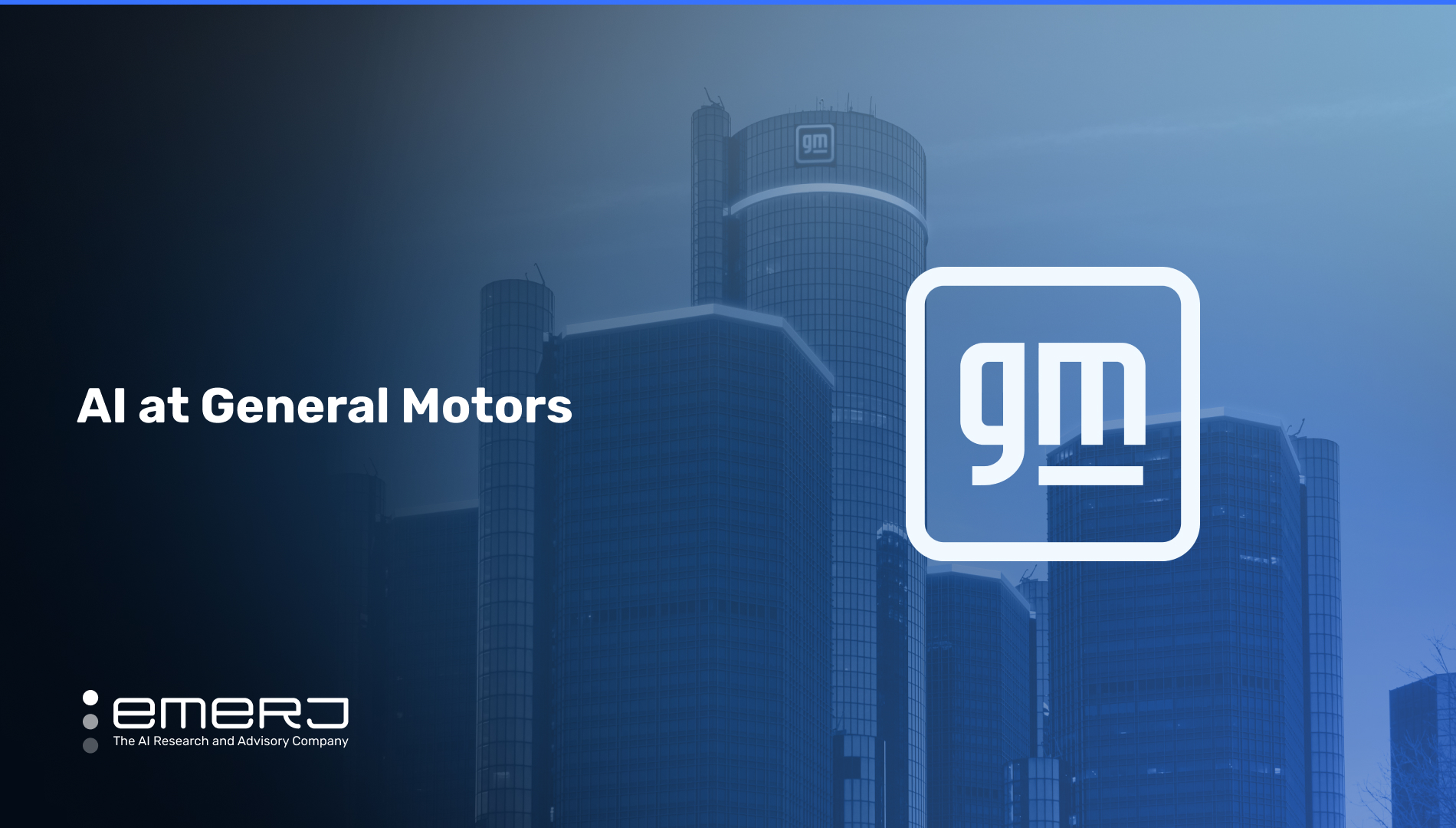
General Motors (GM) is an American multinational auto manufacturing company. For much of the 20th and 21st centuries, GM was the world’s largest motor vehicle manufacturer (since superseded by Toyota). The company produces a wide range of vehicle types, including cars, trucks, SUVs, and vans. Automotive brands owned by GM include Chevrolet, Buick, GMC, and Cadillac.
The company reported a net income of $9.9 billion in 2022. GM employs over 165,000 people across six continents. General Motors is traded (symbol: ‘GM’) on the NYSE.
In this article, we discuss two relevant AI use cases for industry leaders, including:
- Enhanced Driver Assistance: Powered by natural language processing and advanced intent recognition algorithms, the Onstar product is now equipped with features such as automatic crash response, turn-by-turn navigation, and destination prediction.
- Vehicle Lightweighting: Using natural language processing and advanced machine learning algorithms to improve and expedite vehicle design and manufacturing processes is a significant part of reducing vehicle weight and enhancing fuel efficiency.
We begin by examining the first use case: enhanced driver assistance.
Use Case #1: Enhanced Driver Assistance
Likely because its existing Onstar technologies were aging (the tech was introduced in the 1990s) and the uncovering of the massive possibilities offered by advanced natural language processing and predictive analytics, GM sought to enhance the capabilities of its Onstar product through the company’s stated commitment to build a $25 billion subscription service by 2030.
Relating to the first point, the original OnStar product faced some issues and limitations that made it outdated and insufficient for the 2020s/AI era. Here are two key points:
- Service complaints: There have been a number of complaints about the Onstar service. Some users reported issues such as the inability to add a 12-month “data pass,” unacceptable service quality, and instances where the service did not work as promised.
- Limited predictive capabilities: The early versions of OnStar lacked predictive capabilities. In the 2020s/AI era, there is a growing demand for AI-driven predictive technologies. The ability to anticipate and warn drivers about potential vehicle issues before they occur is an important feature that earlier Onstar versions did not possess.
Regardless of motivation, GM partnered with Google Cloud to integrate AI-based technologies into Onstar, resulting in the launch of the Onstar Interactive Virtual Assistant (IVA) in 2022. According to a GM press release, the IVA integrates Google Cloud’s Dialogflow technology, featuring sophisticated algorithms capable of detecting intent.
Google’s Dialogflow is capable of recognizing the intent behind the speaker’s words. Based on these inputs, the IVA generates personalized and responsive outputs, such as routing and navigation assistance. Dialogflow documentation claims that the technology learns the driver’s behavior patterns with repeated use over time, improving the system’s accuracy and efficiency.
Additionally, the system can answer questions related to GM vehicles and product features as well as identify speech that may indicate an emergency before routing the call to a human agent.
The video below demonstrates the functionalities of Dialogflow.
GM reports that the integration of Google Cloud’s Dialogflow technology into existing Onstar capabilities has improved the conversational abilities of the IVA, allowing for more accurate and personalized responses to user inputs.
The report also states that Google Cloud’s AI makes the Onstar system more efficient by better understanding driver requests on the first try, which would enhance the customer experience. Relatedly, GM reports that its customers have reacted positively to no holding times, made possible by a naturally-sounding virtual assistant.
According to GM, the OnStar virtual assistant is now receiving over 1 million customer inquiries monthly from the U.S. and Canada. The initiative appears to be part of GM’s goal of building a $25 billion subscription business within the next few years.
Use Case #2: Vehicle Design Efficiency
A significant issue facing automotive manufacturers is producing more efficient vehicles. Efficient vehicles reduce fuel consumption and carbon emissions, a critical element in meeting increasingly strict environmental regulations. AI-based generative design is also a crucial technology for developing zero-emission vehicles.
Moreover, consumer spending patterns appear to be shifting their spending to more sustainable, environmentally-friendly products – and motor vehicles are no exception.
GM has reportedly partnered with Autodesk to integrate generative design software into its design and manufacturing processes. Generative design involves the use of algorithms and computer programs to create and optimize designs based on a set of parameters.
Generative design stands in contrast to traditional design methods, involving small and incremental changes to an existing design until it meets the design criteria — a more time-consuming and laborious process.
AI-based generative design technology works by taking in parameters from the designers, including the design concept, material types, manufacturing methods, and cost constraints. Algorithms then generate a large number of potential designs and evaluate each one based on how well the developed design meets the manufacturer’s performance criteria.
An iterative process further refines and optimizes the process until the final design is achieved. The designer’s expertise is critical during the iteration process, as they must evaluate each design, determine which ones are most promising, and make the necessary adjustments to parameters to produce the ideal form of the product.
A short video (1:47 in duration) shows Autodesk’s value proposition for its generative design product.
According to Autodesk, its generative design technology is a “design exploration process” that permits designers to input their concept (i.e., sketch/drawing) in conjunction with parameters as described above. As such, it appears that creative ideas are still an essential aspect of interior and exterior automotive design.
To support designers in this new endeavor, Autodesk offers an AI Lab that focuses on improving the way that designers and engineers interact with 3D data. This may suggest that Autodesk is working towards developing tools and resources to help designers and engineers use the technology more effectively.
A design engineer reviews options for a seat bracket. (Source: Autodesk)
According to GM’s Director of Additive Design and Manufacturing, generative design has allowed the company to produce part-design solutions that would have been “impossible” with the designers or engineers working alone. If true, we can reasonably extrapolate that generative design has had a positive, albeit immeasurable, impact on GM’s business outcomes.
Potential business and financial implications of the new technology include cost savings through mass optimization and better market positioning for future automotive products. According to the company’s financial reports, GM has experienced significant year-over-year increases in operating income in recent years’ data, dating back to 2019 before plateauing and decreasing marginally in 2022.
Additionally, we can point out two significant factors that could lead to impactful business results:
- The consolidation of eight different components of a seat bracket into one 3D-printed part not only streamlines the production process but likely results in cost savings as the process eliminates having to rely on multiple suppliers, reducing complexity and potential supply chain issues.
- The ability to create custom parts in-house eliminates the need for a significant capital outlay each time a new, customized tool is required. This could lead to considerable cost reductions and increased flexibility in vehicle design and production.