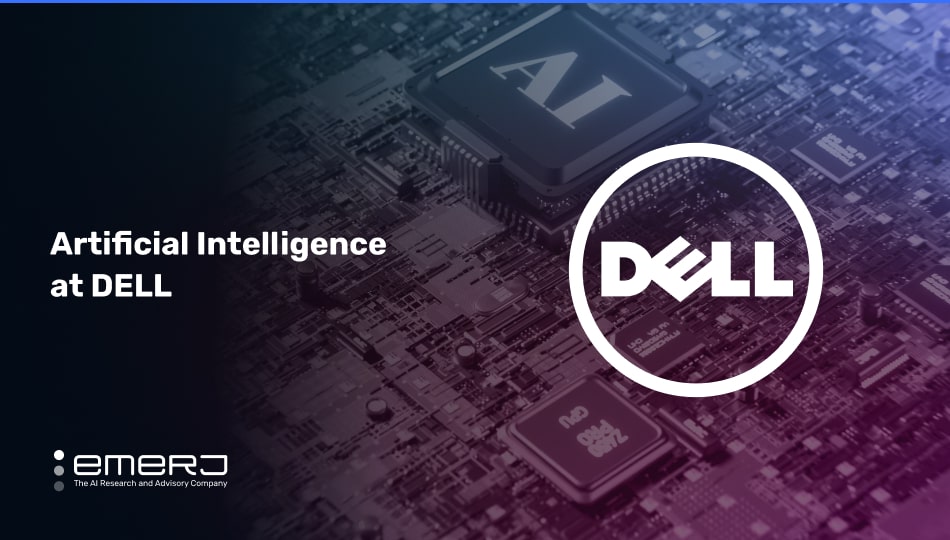
Dell Technologies Inc., or simply ‘Dell’, is a Texas-based multinational technology company. Dell is predominantly known for its personal computing products, particularly its desktops, laptops, and workstations. Gradually, the company branched out into other product and service offerings, including network equipment, software and cloud solutions, and automation services.
In its 2023 annual report, Dell claimed revenues of approximately $102 billion. The company employs about 133,000 globally. Dell is traded on the NYSE (symbol: DELL) and consistently ranks among the top companies in Fortune’s annual ‘Fortune 500’ list.
Perhaps the most significant strategic operational shift at Dell is the company’s decision to incorporate AI and machine learning into its products and services. The move aligns with broader industry trends, where companies increasingly integrate AI to remain competitive and meet the evolving demands fueled by the ongoing digital transformation.
In this article, we focus on two valuable use cases for executives in the technology industry:
- Increasing marketing copy management: Natural language processing (NLP) and machine learning to optimize marketing copy, enhancing consumer engagement across email and Facebook marketing channels.
- Demand forecasting in supply chain engagement: Advanced analytics and predictive modeling to refine inventory predictions, improving product availability and operational efficiency across distribution networks.
We begin with how Dell leverages generative AI to increase engagement with its marketing copy.
Use Case #1: Increasing Marketing Copy Engagement
Dell needed to grapple with a decline in engagement from its marketing communications, a critical factor in connecting with small businesses and consumers. In an era where data analytics and machine learning have heightened the importance of personalized marketing, Dell faced the challenge of enhancing customer engagement to maintain a competitive edge.
According to Persado, Dell’s partner for this project, the challenge was three-fold:
- Stagnant response rates that negatively affected downstream sales metrics.
- The difficulty of customizing language for different audience segments is due to a need for actionable insights.
- The challenge of scaling marketing operations stemming from disconnected data sources.
To tackle these problems, Dell engaged Persado’s AI-powered platform, which is reputed for its language and optimization capabilities in marketing communications. The platform leverages vast data sets and employs advanced AI, deep learning, and a proprietary decision engine to develop marketing copy designed to engage Dell’s potential customers more effectively.
The data processed by the Persado platform encompasses:
- Customer engagement metrics, such as email interaction rates and conversion statistics, to enhance email campaign effectiveness.
- Sales data, including revenue and customer lifetime value, to link specific language choices with purchasing behaviors.
- Results from A/B testing to continuously refine and improve the language used in marketing messages.
- Feedback from customer surveys and social media interactions to adjust calls-to-action and messaging styles to audience preferences.
Motivation AI Platform Workflow (Source: Persado)
The output from Persado’s AI provides Dell’s marketing team with predictive insights for crafting marketing messages. The technology analyzes historical data to identify patterns, which helps predict language that may align with the preferences of specific audience groups. Consequently, this process generates a series of marketing messages aimed at enhancing engagement and the potential for conversions.
From a user perspective, this technology changes the workflow by reducing the trial-and-error aspect of content creation. Marketing professionals can now rely on AI-driven recommendations to craft messages, streamlining the content development process and enabling more targeted communications. The platform’s interface allows for more accessible selection and deployment of these optimized messages, fundamentally changing the marketing team’s approach from one that is intuition-based to one that is data-driven.
While financial details of Dell’s investment are not disclosed, the reported improvements in engagement metrics suggested a positive impact on Dell’s marketing efforts:
- A 59% increase in email campaign click-through rates.
- A 79% increase in email campaign conversions
- A 24% rise in Facebook ad click-through rates, along with a doubling in conversion rates.
These metrics indicate that Dell’s utilization of Persado’s AI platform has contributed to an enhanced digital marketing strategy.
Use Case #2: Demand Forecasting in Supply Chain Management
Due to the scale of Dell’s operations, difficulties in understanding demand and consumption across business lines are, at times, problematic. Fluctuating variables, market trends, consumer behavior, economic conditions, competition, technological advancements, and unexpected events significantly affect demand forecasting accuracy. These challenges are not unique to companies such as Dell but are prevalent across business sectors.
The company sought a way to improve forecasted demand signals, including:
- Active Service Units (ASUs): Projects the number of products currently under service contracts.
- Dispatches: Forecasts technician or part dispatches needed for service fulfillment.
- Contracts: Estimates future sales or renewals of service contracts.
- Ship Quantity: Predicts the number of products to be shipped.
- Service Requests (SRs): Anticipates customer service inquiries, informing staffing needs for support centers.
To attempt to overcome these supply chain challenges, Dell reportedly tasked its applied data science and engineering team to find a workable solution. Gradually, the team developed a machine learning algorithm leveraging the ARIMA time-series demand forecasting model.
(Source: Dell)
Data fed into the forecasting model includes comprehensive datasets from a Hadoop environment, which are processed using tools known as Hive and Impala. Dell’s report specifies that the input data, referred to as ‘assets & contracts,’ covers several key areas:
- Attributes such as geographic locations, product details, warranty entitlements, sales channel information, and fields related to dispatches and service requests.
- Information about the total number of active contracts during a specific fiscal week.
- Various demand signals encompass Active Service Units (ASUs), contracts, shipments, service requests (SRs), dispatches, and renewals.
This data is methodically processed through a series of steps designed to forecast demand signals accurately. The model examines the input data, which includes the detailed attributes and total contracts, and employs an ARIMA time-series model to estimate future demand on a weekly basis.
The forecasting process includes several stages, starting with an initial contract forecast that business teams review and refine. Forecasts for ASUs, dispatches, and SRs follow this. The final results are presented in a format called an ‘Intermediate Cube’ for further review and analysis by business teams. The model also incorporates several refinement techniques:
- High and low-volume forecasting
- Normalization
- Holiday significance analysis
- Discontinuity detection and correction
The output for the user is a comprehensive forecast of various demand signals presented in a cube format. The end-user workflow is improved in that a predictive view of demand across the five demand signals is provided.
According to Dell’s report, the ARIMA models achieved:
- Lowest Mean Error (ME): -0.18
- Lowest Root Mean Square Error (RMSE): .52
- Lowest Mean Absolute Error (MAE): 0.266
- Lowest Mean Absolute Scaled Error (MASE): .752
No financial results were reported. Provided that Dell is accurate regarding the model’s performance and that the model outperforms previous method(s) of demand forecasting, the following business impacts could be expected:
- Operational efficiency: With lower ME, RMSE, and MAE, the ARIMA model could provide more accurate forecasts. This could lead to better inventory management, reducing shortages and overstocks, potentially leading to cost savings and more efficient resource allocation.
- Financial planning: A lower MASE score indicates the model’s accuracy relative to the baseline model. As such, economic forecasts and budgetary adjustments may be more reliable.
- Customer satisfaction: Accurate forecasts of SRs and dispatches ensure that Dell can allocate the right resources to meet customer service needs promptly.
- Supply chain resilience: Accurately predicting demand signals means Dell can better respond to market trends, consumer behavior, marketing conditions, and unexpected events.
- Strategic decision-making: Comprehensive demand forecasts enable decision-makers to plan more effectively for procurement, staffing, and other operational needs.