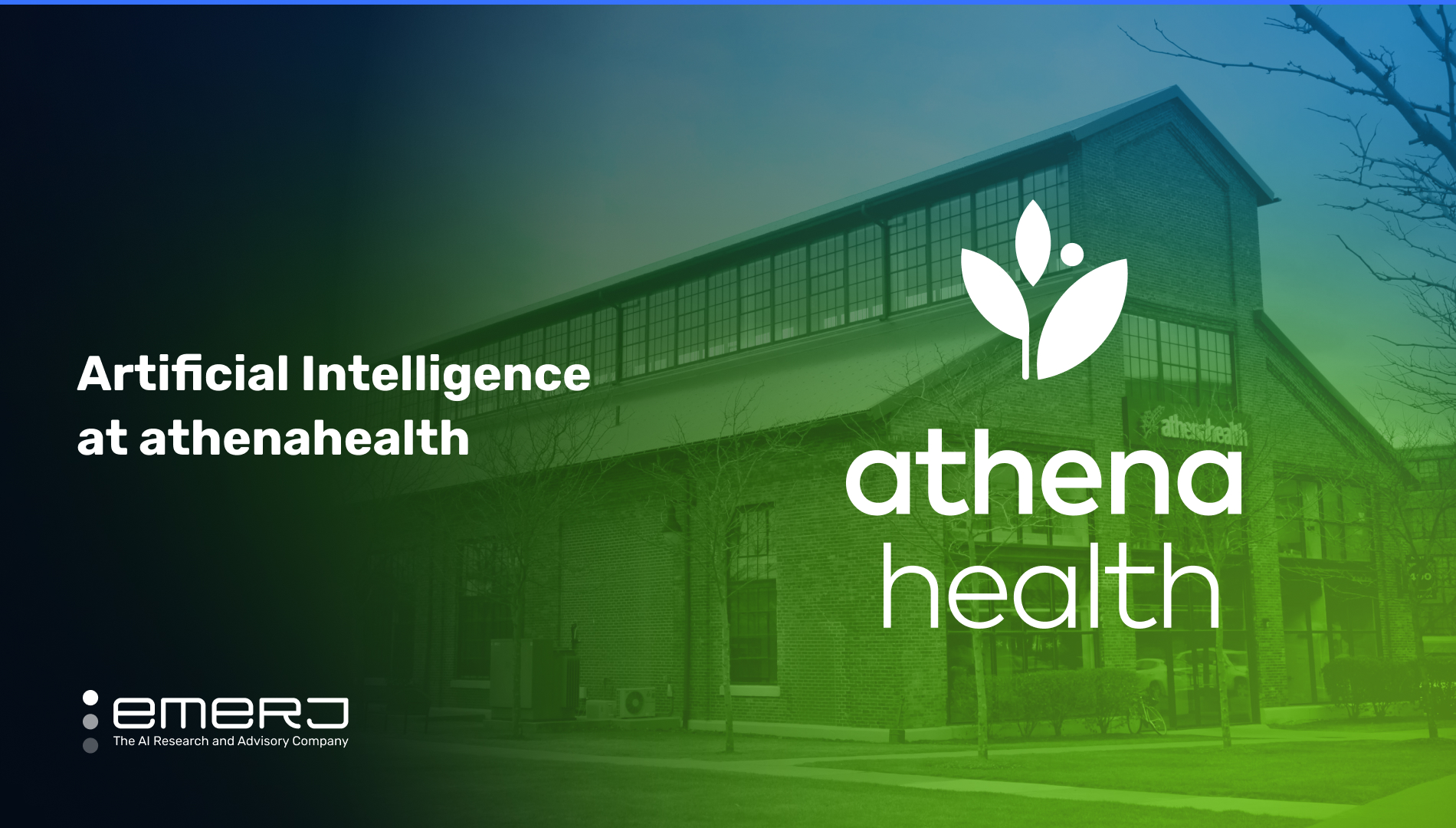
Athenahealth is a private healthcare company specializing in providing network-powered health services and point-of-care mobile apps. The company’s products and services are designed primarily to enhance patient care, improve operational efficiency and streamline administrative processes.
As a private company, athenahealth [sic -ed.] does not release annual reports. The company was purchased by Bain Capital and Hellman & Friedman in February 2022 for $17 billion. It is estimated that the company employs approximately 6,000 professionals. Athenahealth serves more than 160,000 healthcare providers across all 50 US states.
Reportedly, athenahealth integrates several AI technologies in both internally and its product offerings. For example, natural language processing (NLP) for its AI-based electronic health records (EHR) and electronic medical records (EMR) platforms; generative AI for note creation and form filling functionalities; and machine learning algorithms for analyzing and interpreting clinical and patient records, as well as automating tasks like data entry and email responses.
In this article, we examine two use cases that are particularly relevant for executives in the healthcare technology sector:
- Unified search and index: Using analytics, natural language understanding (NLU) and machine learning algorithms to provide a unified context- and behavior-based search to customer service agents, improving satisfaction rates on both sides, as well as case deflections and resolution rates.
- Clinician dictation in EHR/EMR: Integrating natural language processing (NLP) and machine learning into EHR and EMR systems for more natural-sounding clinician-system interactions, increasing provider satisfaction and improving documentation accuracy.
We begin by discussing and analyzing athenahealth’s unified search platform.
Use Case #1: Unified Search and Index
Athenahealth was reportedly grappling with significant challenges in its knowledge management processes and goals, primarily due to knowledge fragmentation across multiple repositories. This fragmentation obstructed support agents from accessing necessary information within a single view, thereby undermining their ability to offer accurate and timely customer support.
Athenahealth sought a solution capable of searching for and providing information to agents across knowledge bases. The company ultimately partnered with. Coveo for this purpose, according to Coveo’s own case study. According to Coveo, the AI-enabled search and index cloud platform, ‘Coveo Relevance Cloud,’ was connected to athenahealth’s “entire knowledge base.”
Based on Coveo’s case study documentation, the unified knowledge base combined with the search optimization afforded by machine learning algorithms, enables athenahealth’s agents to receive more relevant search results and recommendations.
The study also claims Coveo was able to achieve a marked improvement in the relevance of results, a purported byproduct of Coveo’s machine learning model. The AI-enhanced system reportedly creates a single, unified index of disparate systems and user behavior signals, meaning that search results are not only based on the query but on past user interactions and behavior, resulting in more relevant and personalized outputs.
Coveo Relevance Cloud is integrated into athenahealth’s existing CRM system, Salesforce, via API. This feature seemingly eliminates the agent’s need to log in to multiple systems or switch between applications, achieving the unified knowledgebase and search that athenahealth sought to implement.
In the approximately five-minute video below, Coveo the features and value proposition of the Relevance Cloud product:
According to the video and use case documentation, the solution appears to take in the following data:
- Agent queries: Queries related to customer cases they are handling or sought-after information.
- Content repositories: System AI accesses a unified knowledge base consisting of multiple repositories, internal platforms, Microsoft SharePoint, and an internal hard drive storing release notes.
- Agent behavior: Behavior-based analytics track agent behavior such as click patterns and search history.
These inputs are then processed using AI algorithms to generate outputs such as:
- Search results: The primary output; a list of search results relevant to the agent’s query based on Coveo’s algorithms.
- Content recommendations: Documents or articles that the AI predicts may be useful to the agent based on the query and behavior analytics.
- Self-service content: A searchable customer knowledge base covering common potential call-in issues and more.
- Performance metrics: Metrics related to agent performance, including the time taken to resolve a case, the number of cases resolved, etc.
Regarding business results, Coveo reports the following:
- 35% increase in knowledge base articles attached to cases (indicating more success finding the right information)
- 18% increase in manual search
- More than 75% of all clicks on documents “boosted” by Coveo’s machine learning model (indicating the model is effectively identifying and promoting relevant documents)
Use Case #2: Webinar and Lead Optimization
Athenahealth encountered challenges in optimizing its marketing webinars, the primary source of lead generation and sales touchpoints for the company. In uncovering inefficiencies, athenahealth also sought to increase user engagement during webinars to generate higher quality leads.
To achieve these goals, athenahealth partnered with ON24, a technology company providing webinar software and virtual event solutions. Per ON24’s value proposition, the company claims their platform enables users to create, scale and personalize webinar and other content experiences that drive engagement and generate first party (read: potential customers) data.
Further deducing processes from the case study documentation, Webcast Elite appears to take in real-time user engagement as input data. Interactions during the webinars – questions asked, polls answered, items downloaded, and others.
Ostensibly, the AI-enhanced system then processes this information, generating output in the form of account engagement profiles, content insights, dynamic dashboards, and metrics. A user engagement score is then generated, which appears to consider the following variables:
- Engagement level (high, medium, low)
- Total engagement (in hours, minutes, seconds)
- Total touches
- Business Interests
Per ON24, its “AI Engine” determines personalized content recommendations to “ensure informed follow up.” It is likely that this engine uses a combination of AI technologies, including:
- Natural language processing: to analyze and understand text-based interactions.
- Predictive analytics: to generate insights and recommendations in determining which webinar content is most likely to engage users.
- Machine learning: to process and learn from large quantities of data and improve the system’s predictions and recommendations over time.
The user workflow for the platform appears to involve the integration of ON24’s solution with athenahealth’s Eloqua marketing automation and Salesforce customer relationship management (CRM) platforms. There also appears to be one centralized login with a central point of access across systems.
The following 1.5-minute video demonstrates some of the product features and value proposition of the Webcast Elite product:
Reportedly, the business outcomes for athenahealth have been positive. Among the reported results:
- Better access to leads: Logging more than 100 meetings with leads after one webinar about a new healthcare regulation.
- Less time interpreting and managing data: The report describes the solution providing a better flow of data between systems, automated data collection and analysis, and AI-driven insights. All of which the report claims saved athenahealth’s marketing team “hours of time” but does not specify details.