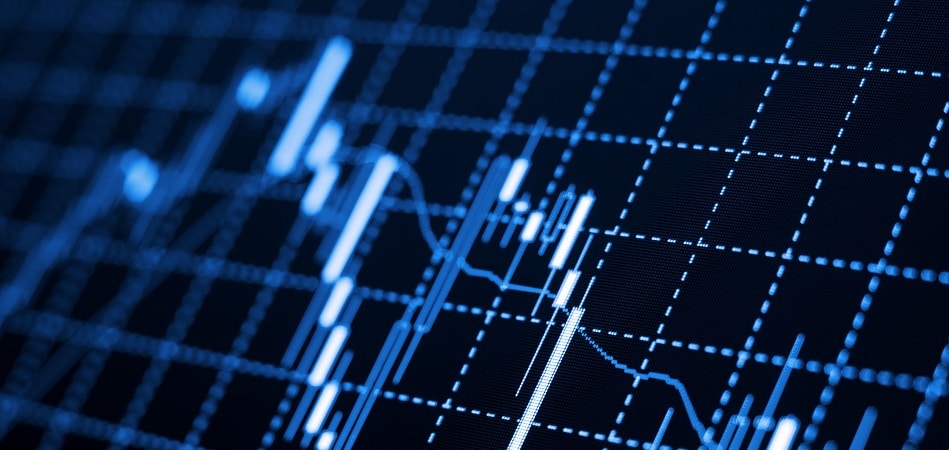
Several high-profile banks are leveraging anomaly detection solutions for fraud and anti-money laundering. While some banks and AI firms provide information on how their solution works or how their chosen solution worked for them, it can be hard to determine which ones are successful today.
In this article, we provide two detailed examples of AI firms that offer evidence of success with their anomaly detection solutions. These solutions are made to detect and stop a variety of fraud methods.
These range from malware and more conventional banking fraud methods to money laundering attempts through receiving and sending payments from suspicious entities. The anomaly detection solutions we explain include:
- Feedzai’s Anomaly Detection-based Fraud Detection Platform: Using AI-powered anomaly detection technology to recognize and stop attempts at bank fraud.
- Ayasdi’s Anti Money-laundering Solution: Anomaly detection used for recognizing changes in customer behavior and analyzing them for patterns related to money laundering or fraud.
We begin our explanation of these anomaly detection solutions with Feedzai’s fraud detection platform and its success with Citibank.
Feedzai’s Anomaly Detection-based Fraud Detection Platform
The AI startup Feedzai sells their anomaly detection platform to banks and other industries for fraud detection. They claim their software can help their clients prevent fraud and money laundering by using detailed risk profiles for customers and scoring their likelihood of fraud based on granular data regarding each customer.
Anomaly detection applications require a machine learning model that is trained a continuous stream of incoming data, such as banking transactions happening in real-time. Feedzai’s models can purportedly be trained to recognize a baseline sense of normalcy for the data within banking transactions, loan applications, or new account information.
Like most other anomaly detection applications, the software can alert a human employee of any deviations from the typical pattern so that they may give it a closer review for fraud.
The employee can choose to accept or reject this notification, which the machine learning model can use to recognize if its determination of fraud from a single transaction is correct. This further trains the model to understand that the deviation it recognized is acceptable, or indeed an indication of fraud.
The image below provides a simple depiction of how Feedzai’s platform works. The raw transaction data flows from the real-time data stream, large data stores referred to as “data lakes,” and APIs into the OpenML Engine platform.
This platform then routes the data towards the endpoints necessary to conduct experiments and real-time analysis. At the same time, this data funnels into the “Feedzai risk studio,” a database the company uses to improve future ML models, products, and software development methods
Feedzai’s software platform can purportedly complete these tasks by monitoring each transaction for unusual payment behavior or any discrepancies in the information used to validate, authenticate, or otherwise confirm them. It would then need to analyze each anomaly before determining if the transaction is likely fraud. If the transaction proved to be legitimate, the software would then clear it to continue through the client bank’s payment processing system.
Feedzai claims their OpenML Engine, a user interface for creating new machine learning models, can help data science teams at banks to customize new ML algorithms using provided samples.
This can be invaluable for banks that are trying to build a data science team and train them to have expertise with banking data that could indicate fraud. Our readers can find a demonstration of how one might use this interface here, although Feedzai does require some identification information before displaying the video.
We spoke about how banks may benefit from this type of AI tool in an interview with Lee Smallwood, COO of Markets and Securities, North America at Citi, on our Podcast, AI in Banking. Smallwood emphasized his view on how banks should approach building and leveraging their data science and AI teams. He focused on how banks can create a culture of innovation so that their company can find success and benefits outside of direct ROI. When asked how a large bank might be able to do this, Smallwood said,
…Probably the biggest difference in that kind of culture of innovation is … a comfort with failure that I think a lot of large financial institutions still struggle with. If you want to attract or attain good people you need to have an environment where people are able to move quickly, and that failure is welcomed as part of the process of getting to success. I’m not saying that we’re comfortable with everything going wrong, but that people aren’t going to be reprimanded for making a couple of errors on the way to something that could be really exciting.
Smallwood claims that creating a culture of innovation means that the c-level staff and other bank managers will need to be comfortable with some failures when working on applied AI initiatives. This is especially true for AI applications because of how long they take to develop and install into the enterprise’s tech stack. Some of these types of applications make take several months of research and development in order to determine whether the bank can use a machine learning model in an application that drives value.
Business leaders at established banks may not be able to appreciate the fact that this is how long it might take to develop an AI solution. However, Smallwood offered us more ideas about how someone may be able to communicate this effectively. He mentioned how some established companies are beginning to open corporate innovation departments in their company for this and similar reasons. Additionally, he explained that having a place where AI researchers and data scientists can work on new initiatives and experiments can show upper management exactly what it takes to build an AI software. Regarding this, Smallwood said,
Putting a few technologists and a ping pong table in a room does not an innovative bank make. But I think having a sort of ring fenced area where people are able to try new ideas, are able to come into the business and talk about what they’re working on [is beneficial.] A lot of these groups from what I’ve seen have more external relationships with the startup community and the venture investment community and so having examples of that is helpful in sort of initiating this cultural change.
Feedzai’s Success with Their Platform
In addition to a case study in which Feedzai details their success with a large retail bank adopting their solution, there is a lot of news surrounding their 2018 partnership with Citi. The bank claims they intend to adopt Feedzai’s platform into its current payment services such as accounts payable. This may help clients take more control over risk management for all of their transactions.
Manish Kohli, Global Head of Payments and Receivables, Citi’s Treasury and Trade Solutions (Citi TTS), mentioned that this project is exemplary of Citi’s commitment to innovating the banking space using the newest technology.
It may appear that Citi is focused on providing risk mitigation and fraud detection without slowing their claims processing workflows. In addition to the near constant innovations in payments and fraud detection, cyber attacks and malware are also always improving.
Citi claims that their clients are aware of this, and so expect faster and safer transactions all the time. They claim their adoption of Feedzai’s software and platform will help their clients stay aware of new threats without spending any more time.
Ayasdi’s Anti Money-laundering Solution
Ayasdi offers an anomaly detection based solution for detecting and stopping money-laundering transactions. They claim the software analyzes the entities customers are paying or receiving payments from to make sure the funds are coming from legitimate sources.
Additionally, they claim to accomplish this by analyzing customer behavior over time and detecting potentially harmful patterns as they appear. The Ayasdi AML offering contains the following four capabilities:
- Auto Feature Engineering: Automatically detecting aspects of transactional data that are likely to reveal potential fraud patterns.
- Intelligent Segmentation: Creating thresholds that form customer segments based on their transaction history and real time behavior.
- Behavioral Insights: Keeping track of daily changes in customer behavior and creating lists of customers showing the most deviation.
- Intelligent Event Triage: Recognizing which transaction events require further investigation and which ones can be treated as acceptable deviations.
Auto Feature Engineering
The Auto Feature Engineering tool within Ayasdi’s solution allows the software to recognize the attributes within data that contain “signal.” What Ayasdi likely means by this is data points that can actually be considered deviations from normal transactional behavior, but to a more granular degree so as to recognize potentially harmful patterns.
These patterns can be early indicators of money laundering, and so it would be important to keep track of these patterns as they emerge. Ayasdi claims that these specific aspects of transactional data along with the patterns they form are used to create thresholds of deviation relative to the machine learning model’s baseline sense of normalcy. This would feed into their intelligent segmentation solution so that the software can organize potential threats by the likelihood that they are actually attempts at money laundering.
Intelligent Segmentation
In order to break up large amounts of real time data into segments that the solution’s machine learning model can analyze, intelligent segmentation can create thresholds that divide customers based on changes in banking behavior. As previously stated, the solution creates these segments by analyzing changes in customer behavior and determining how likely they are to be related to an attempt at money laundering.
The company claims their approach to segmentation can help banks reduce false positives in their anti money-laundering initiatives. The software can purportedly analyze large amounts of data faster because it can base its analysis on a certain percentage chance that each transaction is fraudulent. This means the analysis of each customer segment requires much less time than if the client tried to analyze an entire day’s worth of data at once, for example.
Below is an image of the intelligent segmentation user interface. A data scientist can compare customer a range of customer segments and view specific attributes about each simultaneously:
The segmentation results can deliver more specific customer groups when the software is provided data from more varied sources. Ayasdi claims their AML technology does not require labeled data in order to create customer segmentation, although how this is possible is unclear.
In order to get machine learning algorithms to recognize data as a specific aspect of a transaction, all data contained within the transaction would need to be labeled. One way this could be possible is if the AML solution came with a tool that automatically labeled the aspects of banking transactions that relate directly to principal money-laundering methods, although Ayasdi does not state this.
Behavioral Insights
Ayasdi’s solution produces daily lists of any changes in customer behavior across each day’s transactional data. These changes in behavior can include metrics such as how far they deviate from normal behavior over time, or how that compares to other customers in the same segment.
The image below shows Ayasdi’s behavioral insights interface. Analysts can organize transactions with deviations in behavior based on how much their patterns have changed since the opening of their account. They can also view them according to the dollar amounts each transaction is worth and compare that to the deviation rate:
Additionally, the behavioral insights tool can show when customers move to a new segment and provide and explanation for why. The tool can also compare these deviation metrics to any customer information provided by large customer data resources such as CRMs or a KYCs.
Intelligent Event Triage
Event triage is a technique which allows the AML solution to identify the most valuable transactional data for investigators to pay attention to or analyze in the future. This purportedly results in the software highlighting a relatively smaller amount of events than another solution might. However these results would have a higher potential value of discovering money laundering or important indicators thereof.
Ayasdi’s Success With Its AML Solution
We have conducted a case study on HSBC Bank’s adoption of Ayasdi’s AML solution, in which the company claimed to have reduced the bank’s false positives by 20%. They also stated that they found numerous cases and behavioral patterns that were directly related to fraud.
This was accomplished by HSBC’s IT team working with Ayasdi to give them access to their AML data. Additionally, their internal modeling review team helped ayasdi create customer behavior models that the bank could recognize according to the terms they are used to reading.
Some banks see building a talented internal team for data science and AI as a serious challenge to overcome in the age of AI. This can include training their internal modeling team to understand these concepts. We spoke to Adam Hunt, CTO of Risk IQ about this on our podcast, AI in Banking. When asked how banks can continue to improve their tech stack with AI in addition to finding the best talent, Hunt said,
Training is number one. … There’s plenty of courses out there to train people on basic machine learning. We do a lot of advanced stuff to cover the entire internet … with a very high precision in our models. Because every false positive, you are ringing the alarm bell. But inside of a bank you may have laxer requirements. That is something that you may not need a machine learning phd to tackle all the problems. There are certainly some that you do, but for the large majority, [you’ll get a lot of value out of] a basic course in machine learning and some basic Python skills.
While it may seem difficult to help banking staff grow and adapt to new AI initiatives, it is important to note that some experts advise to start small. With basic machine learning training, an internal modeling team at a bank may be able to cooperate with an AI vendor in a similar way to HSBC’s project with Ayasdi.
Ayasdi’s website provides another case study in which they help an established correspondent bank adopt an anti money-laundering solution. The bank had a goal of improving its efficiency in detecting money-laundering attempts by 3-5%, and needed to adapt to the complexity of adopting an AI solution which was a wholly different experience from their previous manual operations.
The bank and Ayasdi worked together to analyze data from their customers’ Swift messages, or a financial message sent through banking code. The bank had never attempted this before, and the inclusion of this extra data source allowed them to find multiple new criteria to analyze.
These included specific attributes of transactional data such as type, direction, value, originator, and date. Additionally, this included geographical and chronological data that created more distinct customer segments.
Ayasdi claims the bank was about to reduce the volume of human money-laundering investigators required to function efficiently by 20%. They did this over an eight-week project included two data scientists, one of Ayasdi’s project managers, and two domain experts from the bank. The case study states that the bank decided to move forward with Ayasdi’s solution and deploy it globally.
Header Image Credit: SpaceTime Insight