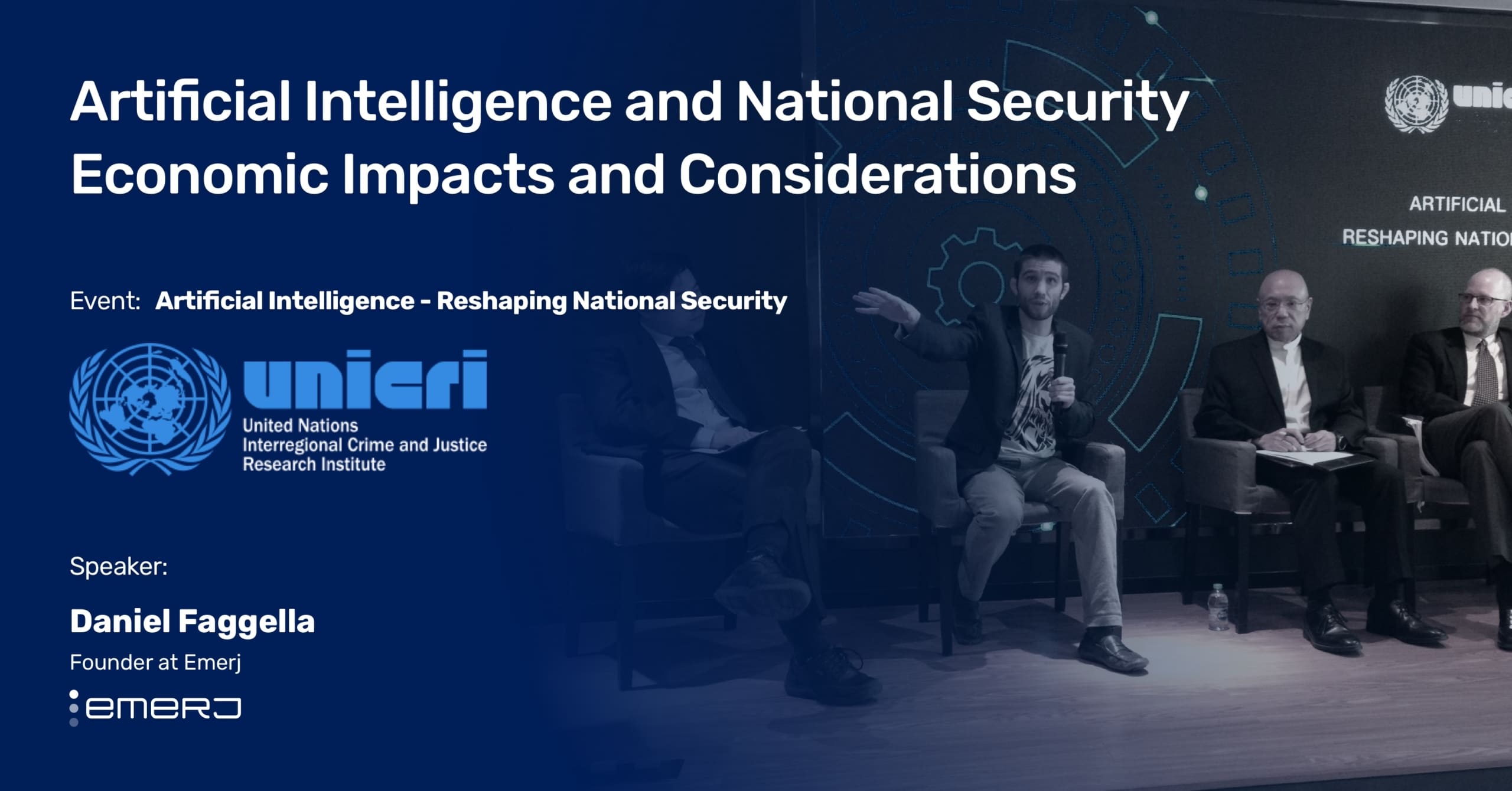
In July 2017, The State Council of China released the “New Generation Artificial Intelligence Development Plan,” outlining China’s strategy to build a US$150 billion Chinese AI industry in a few short years, and to become the leading nation in AI by the year 2030. Other nations followed suit quickly with national AI strategies of their own – with the US trailing behind by nearly two years before developing a semblance of an AI initiative. The proposed 2021 budget for the national security budget in the US is $740 billion – with a billions of dollars being earmarked for AI specifically (learn more: US Public Sector AI Opportunity Report).
AI applications play a considerable role in the direction of technology development in many defense sectors, particularly in surveillance, intelligence gathering, reconnaissance, logistics, command and control, cyberspace, and information operations – but AI’s relevance for national security is just as much in it’s implications for the economy as it is for defense itself.
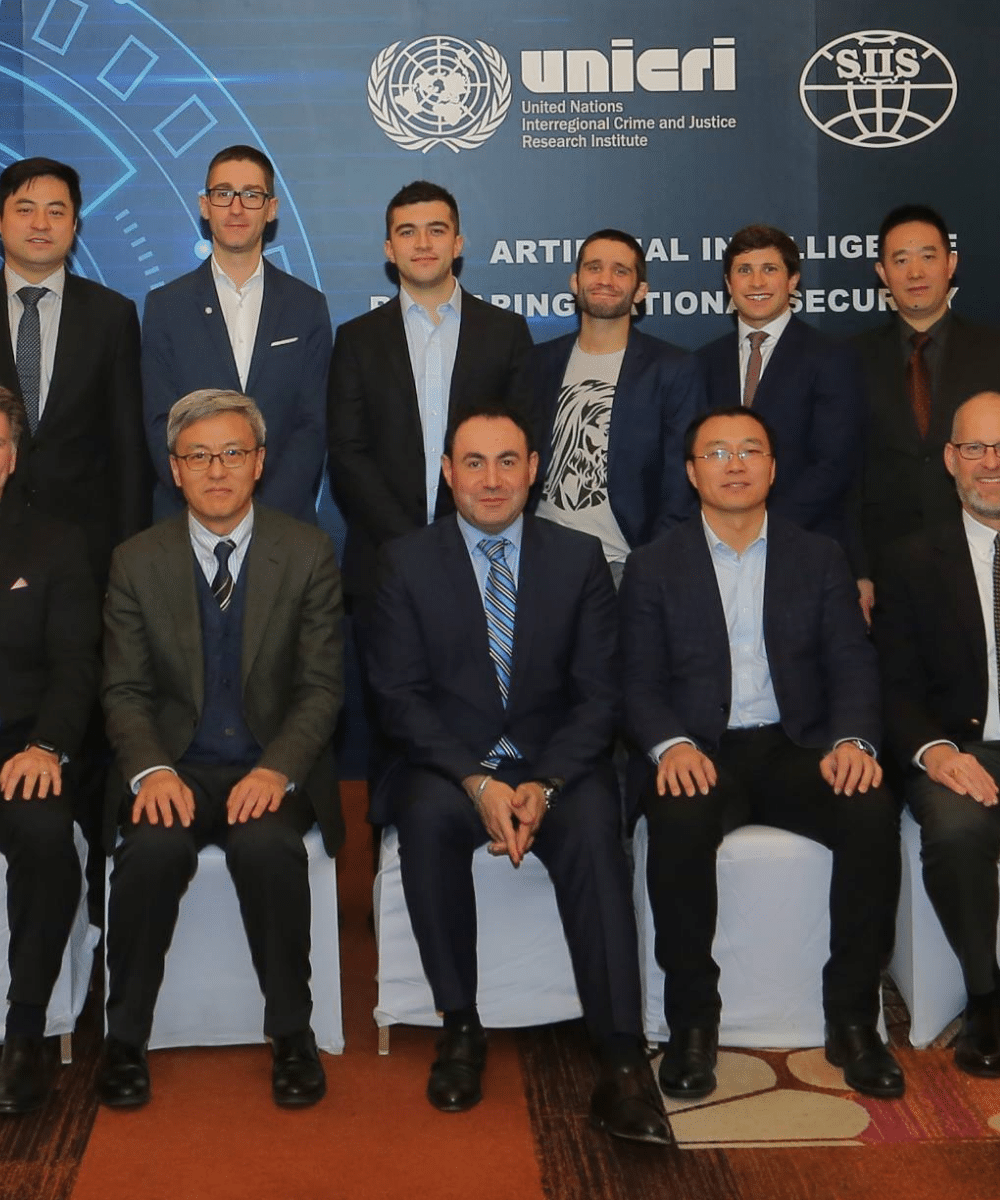
This article is based on my presentation at the UNICRI / Shanghai Institutes for International Studies event Artificial Intelligence – Reshaping National Security – held in Shanghai.
While I’m not able to embed my full slide deck from that presentation publicly, I am able to share some of the key ideas from my talk – with a focus on AI job loss and defense implications.
Note: Readers with an explicit interest in AI for defense might enjoy our coverage of AI in defense and military.
In this article we’ll discuss the impact of job automation on national economies, we’ll discuss some specific industries and business process that may be automated sooner than others, and we’ll recommend some next steps for policymakers and business leaders to consider.
The 3 C’s of Job Automation: Context, Connection, Coordination
However, it would be a gross generalization to say that AI and automation only take jobs away from humans. There are plenty of new roles that will be created as AI begins to proliferate across industries. That said, many AI experts agree that job automation is among the largest AI risks of the next 20 years ahead – and for this reason alone it should be on the national security radar for developed nations.
Some jobs are more automate-able than others, and it’s important to have a framework for understanding which jobs are at greater risk.
Emerj.com conducted two years of one-to-one interviews with AI leaders in different industries as well as with people selling and offering artificial intelligence to these sectors. The research revealed common patterns of the types of work that seemed to be eliminated (or most eliminate-able) as a result of AI.
We’ll begin with the first C, “Context”:
1 – Context
Context refers to the number of competencies required to perform a job. It is arguably the most important aspect of work that would dictate the extent of the impact of automation.
Jobs with low context, or those that involve a very limited number of competencies are the easiest to automate. These “automate-able” jobs have three factors in common:
- Very predictable inputs
- Very predictable work steps, and
- Very predictable outputs
These dynamics may be present in both blue-collar and white-collar positions.
Blue-Collar Low-Context: Welding on an assembly line.
This would generally be very low context as it involves manipulating machinery and parts in a very regimented way. A welder would be doing rote work dictated by a similar setup, timing, and directions at any time with very narrow focus. Because the work is repeatable and predictable, it would qualify as low-context work, and automate-able.
Blue-Collar High-Context: Plumber.
However, not all blue-collar work would qualify as low context. One example is plumbing, which is arguable high-context work. Plumbers rely heavily on the circumstances surrounding a problem to do the job properly.
For instance, if a basement floods because a pipe broke, the plumber will need to have the social skills to talk to the homeowner or building supervisor and ask pertinent questions to diagnose the cause of the flooding. The pipe might have broken due to a recent cold spike, or perhaps because of improper installation.
A skilled plumber would know how to think backward from a current problem to apply the right solution. This requires more abstract thinking than would be necessary for some working a conveyor belt welding job, even if the work pays about the same. As such, plumbing work is not repeatable or predictable work, so it is not easily automate-able.
White-Collar Low-Context: Insurance underwriting.
Insurance underwriting might be an example of low-context white-collar work. Typically, this would involve a request for an insurance plan, which would go through a static set of criteria to match the request and select the most appropriate plan. The inputs are demonstrably repeatable, the decision-making is predictable, and it goes through the same workflow in each instance. In this scenario, the insurance underwriting job is at risk of automation in the coming five years.
White-Collar High-Context: Procurement management.
On the other hand, procurement management falls under high-context work. A procurement manager needs familiarity with vendor companies to deal and negotiate with them effectively. He or she would also need to assess inventory levels against the company’s upcoming plans to make better projections.
The procurement manager would also need to be able to respond to situations, such as finding a backup supplier in case the regular one fails to deliver.
A procurement manager and plumber need multiple competencies to function in complex situations where there are no simple sets of input and output. As the work requires high-context, these are jobs that are not currently at risk of automation.
A conveyor belt welder and insurance underwriter, however, would be doing low-context jobs. This makes them vulnerable to automation.
2 – Connection
Connection refers to the ability to relate to other people on an interpersonal level, to empathize and attune to the emotions of others. Some jobs require little or no connection with others to carry out a task, and these would be low-connection work. Other jobs would not be possible without such a personal connection, and would be high-connection work.
Jobs that involve high connection have one factor in common:
- Interpersonal skills are critical for success on the job
Low Connection Work
An example of low connection work would be almost any assembly line worker, or even a software engineer. These jobs would not require any empathy or personal connection to execute, generally speaking. Manufacturing or engineering managers and recruiters might need such skills, but the front line workers likely require very little of it. It might be necessary for a software engineer to attend the occasional meeting, but other than that, these workers simply get the work done.
High Connection Work
For high connection work, a good example is that of an elementary school teacher. While it is possible for machines to show up to class and teach children math and reading, they are not yet capable of building a personal connection with their students. Studies show that empathy and personal connection has a direct effect on learning, especially for small children.
Salespeople would also need the ability to connect on a personal level to do their job. They need to persuade and convince consumers to seal the deal, so it is important for them to read nonverbal cues or identify subtle changes in tone of voice to figure out how to bring the transaction to a desirable end. High connection jobs require amorphous, intuitive human skills.
People need to feel a personal connection with their service provider in some instances, and not in others. Jobs with low-connection requirements are therefore more easily automate-able.
3 – Coordination
Coordination refers to the ability to work with teams and resources to reach goals. It also requires interpersonal skills, but not to the same degree as connection.
Coordination is a much less emotional facet of human interaction. It requires more of a cerebral response to accomplish a task in conjunction with other workers. A job with low coordination would be one that does not require working with other workers in general. High coordination jobs would require constant orchestration of multiple tasks executed by a group of workers.
Low Coordination Work
A good example of low coordination work is that of a nurse. Generally, a nurse would execute tasks on his or her own without having to coordinate with anyone else. It might involve a lot of connection, but it would not involve coordination.
The same thing could apply to a software programmer or engineer. While there is a lot of creative work in those cases and involves significant context, the job itself does not require marshaling the resources of the business.
That is not to say that all jobs with low coordination are automate-able. However, it is one step in that direction.
High Coordination Work
High coordination jobs include that of a sales manager and chief technology officer. In both cases, the job requires extensive coordination with team members. A sales manager is not just going out and doing sales. He or she is determining the number of salespeople to hire, how to set up commissioning programs, and how to motivate individual people.
A chief technology officer gives subordinates their direction when developing or using technology, as well as determine training requirements, to make the most of company resources and reach goals. It would be accurate to say that sales managers and CTOs live for coordination.
Coordination is a complex task in most cases. When it comes to the impact of automation on the job market, coordination probably has a higher consideration than connection due to its level of complexity.
I cover context, connection, and coordination in greater depth in my 2018 TEDx talk at the University of Rhode Island:
It’s important to note that no one factor (one of the 3Cs) predicts the automate-ability of a job. It is generally the case that the less Context, Connection, and Coordination a job requires, the more automate-able it is – but a job requiring almost no connection may still be human-reliant if Context or Coordination are high.
A Note on People Skills and Automation
The 3Cs of job automation describes in general terms the type of work that are most and least likely to be safe from automation. In other words, people in jobs that require high levels of context, connection, and coordination are probably safe from automation.
In fact, it might even take only one of those three factors to make it very challenging to automate with artificial intelligence in that requirement is very high. It might seem, then, that jobs that require people skills are safe from automation. However, that is not necessarily the case.
Customer service representatives, for example, use significant amounts of people skills daily. The same might be true for millions of checkout clerks and cashiers.
While people skills might be involved in these jobs, they might still be at risk for automation, in part because connection is not critical to those roles. It might improve the customer service or checkout experience when the person on the other end is pleasant, but that is not the goal of the consumer.
An AI-powered chatbot or automated checkout system can do the job just as well. In fact, many people today have no problem dealing with a chatbot for simple customer service concerns, and some even prefer it because of shorter wait times and simpler interactions.
In other words, if connection and/or coordination is absolutely a requirement for the job, then it is much more challenging to automate those positions. Jobs predicated on coordination and connection are relatively safe from automation, but jobs where connection or coordination are ideal, but not necessary for the job, are less safe from automation.
This distinction applies to most industries and sectors and in most countries, although there are variations as discussed in the next section.
Sector-Specific AI and Automation Impact
The impact of AI on specific sectors such as agriculture, banking, and retail should be expected to vary from country to country based on a variety of variables:
- Processes/management norms
- Trade agreements with other countries
- Education systems and relative AI talent density
- Timeline to AI/tech adoption
- Labor costs (high cost = high automation incentive, lower-cost = lower automation incentive)
Sector-specific impact of AI and automation varies from nation to nation because they have different forces at play. Agriculture, for instance, might have different processes, management, and products.in Croatia as compared to China or the United States. Trade agreements with other countries, labor costs, and technology adoption would also come into play. In Croatia, there may be more incentive to adopt automation due to high labor costs.
Making a generalization on the impact of AI and automation in any sector is very challenging and disingenuous., Any broad paintbrush of that kind would be misleading. The real landscape is much more complex.
On any given space, there will be varying levels of AI and automation adoption, and divergent emphasis of AI capabilities under varied political and economic conditions. To illustrate, a recent delve into the application of AI in public healthcare sponsored by the World Bank indicated that AI integration in different countries varied widely according to their current needs and available resources.
That said, extensive research in the US focused on sectors other than public healthcare. According to McKinsey’s Research, the following sectors are ripe for automation in the near term:
- Accommodations and food service
- Manufacturing
- Agriculture
- Transportation and warehousing
- Retail
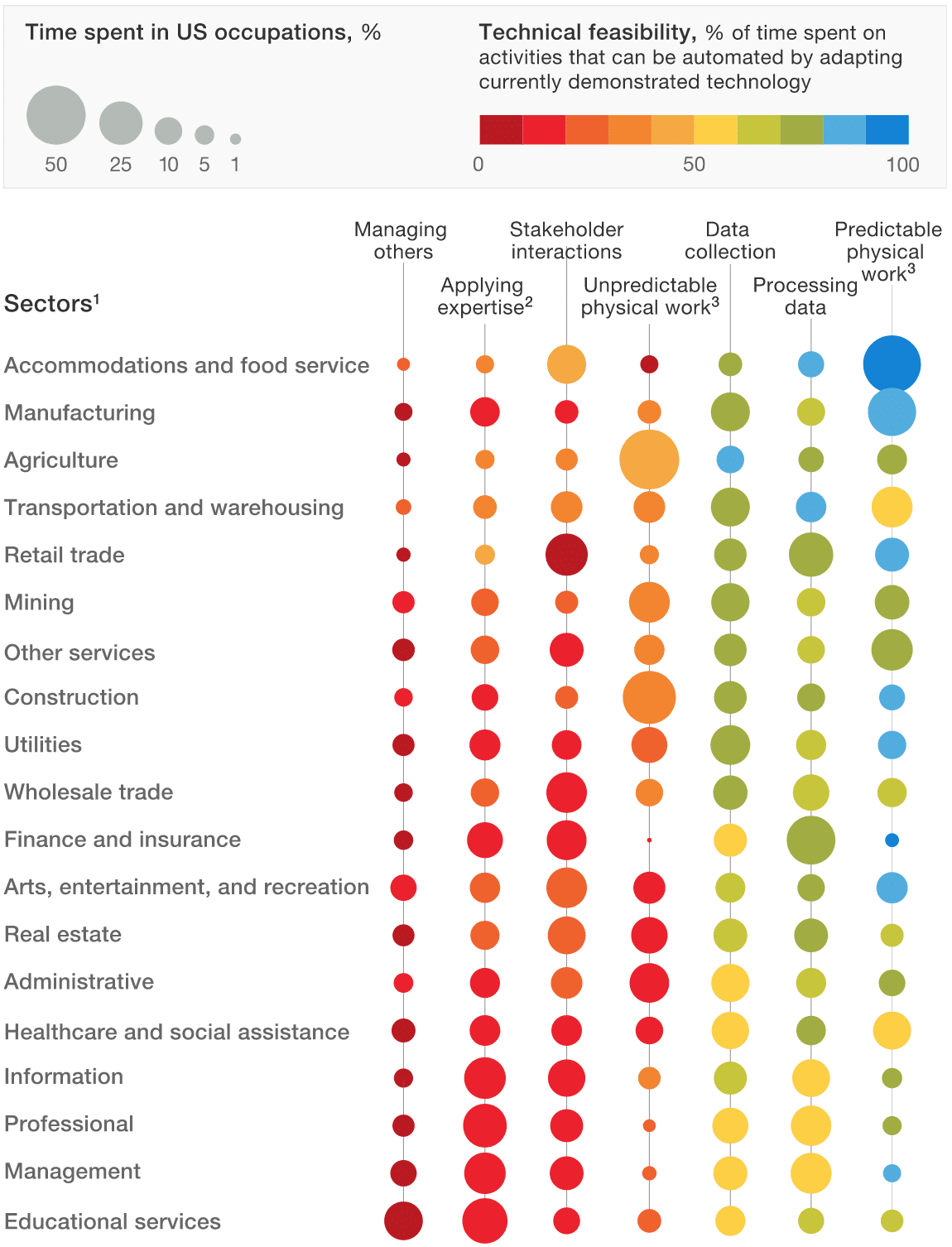
Bloomberg research identifies specific jobs that are at highest risk:
- Cashiers
- Waiters
- Retail salespeople
- Sales reps
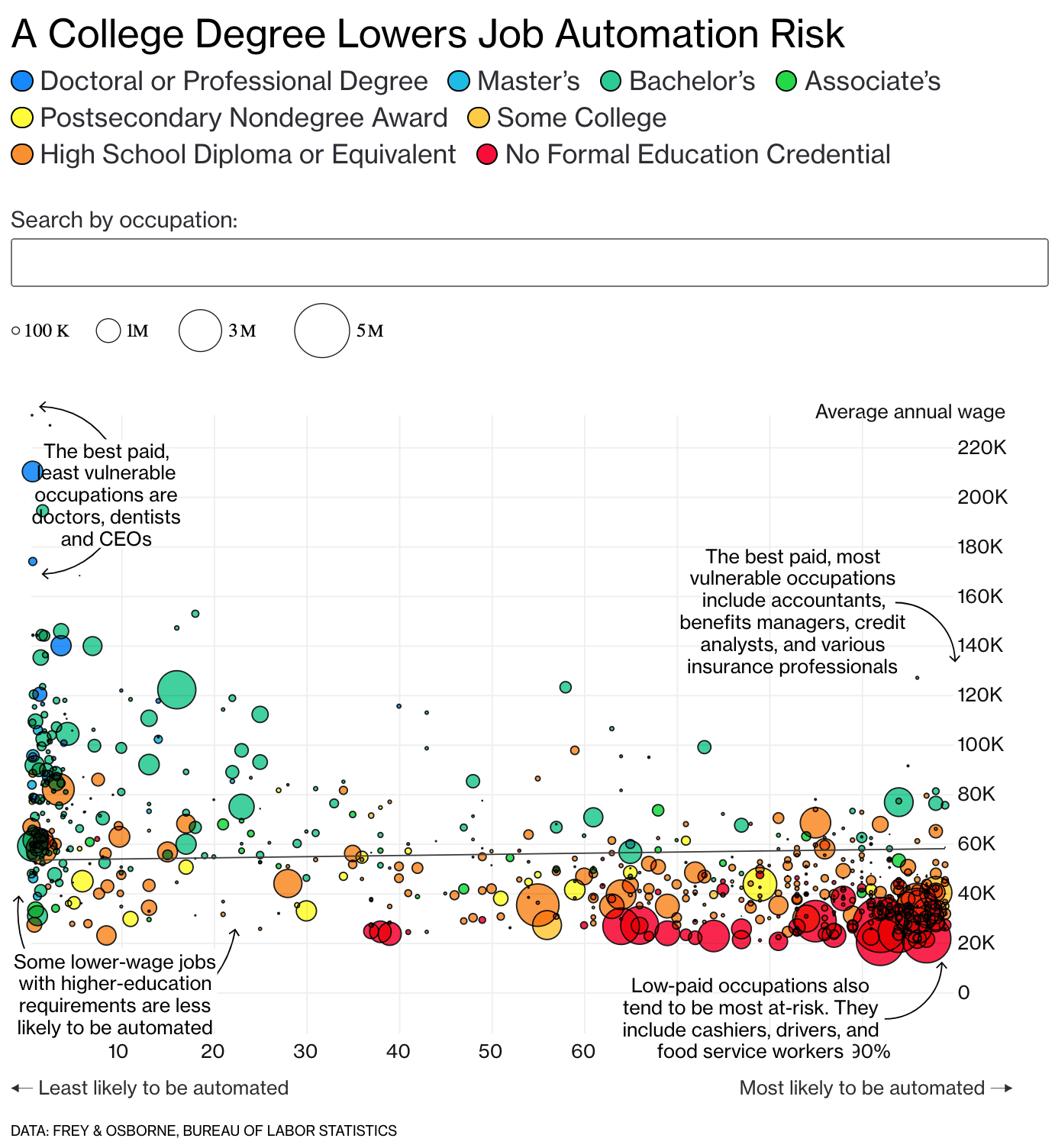
The research shows that jobs that entail physical work in accommodations and food services and in manufacturing are high in terms of automation potential. COVID-19 certainly hasn’t made things easier in these sectors, and we expect food services AI/automation to kick into even higher gear in the next two years as we pull out of the economic tumult.
This represents a large proportion of the current labor force in these sectors. This is useful to know, even though it only represents the situation in the United States, because it indicates a level of risk in those sectors in general.
According to our own cross-industry interviews at Emerj, there is a relatively predictable pattern of the types of jobs that are likely to be automated in major sectors like healthcare, finance, and heavy industry:
- Data entry and basic data manipulation roles – Much of what is now handled by business process outsourcing (BPO) companies.
- Low-context customer service and sales enablement roles – The lowest rung of phone center roles, for example.
- Extremely wrote processing jobs – Insurance underwriters, lending underwriting, handling invoices, some manual bookkeeping work
- Search and discovery assistants – Paralegals, and their equivalents in other sectors
These low-context jobs (and jobs like them) have a reasonably high likelihood of near-term automation. It is worthy to note that this research focused on large enterprises and B2B firms such as IBM, General Electric, and General Motors.
However, a large proportion of many businesses employ people to do purely customer-facing and entry-level work with high automation potential in in the relatively near term. Any job that involves repetitive handling of paperwork and rote tasks will eventually make way for automation – as humans skills shift more and more towards higher complexity in context.
Planning and Preparation for Automation
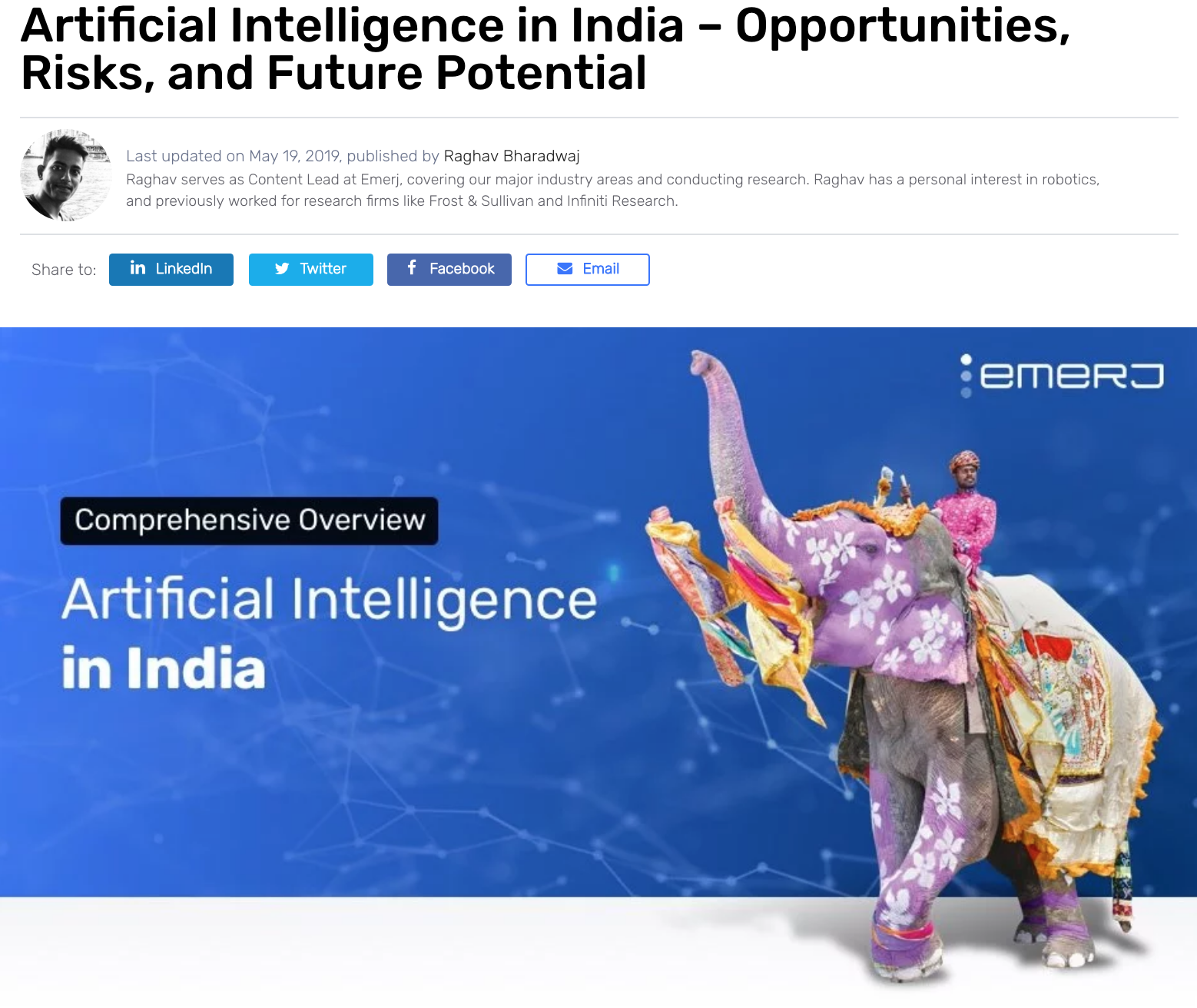
Given that automation would be in probability inevitable for some jobs, it would be important to determine sectors of relevance.
1 – Critical Industries for National Security Interest
The first step is to assess industries that matter most for national security or international economic competitiveness and their potential for growth in the next 10 years.
We believe that it’s critical for policymakers to identify industries that overlap and intersect with national security in important ways, or sectors that involve technologies that might be repurposed for defense and some security objectives. This is relevant because AI-based technology and development is primarily a commercial enterprise. It would possible to have a landscape of repurposed technologies in mobile, retail, and other spaces for use in defense (see our full article on dual-use AI applications for defense: Weaponized Artificial Intelligence – Critical Dual-Use Applications).
2 – Automate-able Industries and Workflows
The next step is determining the processes and aspects of work that might be most automate-able, essentially work that lack the three Cs.
For example, the agriculture sector might benefit from automating the task of planting seeds, weeding, or collecting crops. In the services sector, automation may apply in customer service or rote paperwork processing. In manufacturing, automation would be most likely in assembly jobs and some kinds of maintenance work.
It is our hope that organizations like the OECD or the World Bank will keep track of international trends around job automation, and help to develop a set of playbooks for policymakers. It will be near-impossible for one country to keep an accurate pulse on the shifting nature of work and the progress of automation in different industries and nations – and we believe that this is an important opportunity for intergovernmental organizations.
I’ve been proud to be involved with the OECD in the development of their AI Policy Observatory – a resource you can learn more about at OECD.ai.
3 – Build Pathways Toward Data Dominance
My popular AI Power article titled Data Dominance covers a very important dynamic. The idea came out in 2017 in a series of interviews with Silicon Valley investors, who naturally want to invest in companies with the greatest chance of becoming industry-leading giants (“the next Google” or “the next Facebook”). The dynamic can be explained this way:
How Data Dominance Works
- Acquire more users, customers or installs
- This leads to more data
- More data leads to more learning and more AI applications
- More learning and more AI applications lead to a better product
- A better product that is widely known leads to acquiring more users
- This leads to more data
- (And on and on and on…)
In a nutshell:
More users, customers, installs >> More data >> More AI capability >> Better product >> More users, customers, installs
Central to the issue of artificial intelligence and economic defense and competition is a concept called the proprietary data plume (a quote that I grabbed during an interview with venture capitalist Ben Narasin).
When the company has sustained defensibility in collecting proprietary high-value data and leverages it continuously, it can potentially monopolize the market, much in the way Google has done with internet search, or Facebook has done for social media, or Amazon has done for general eCommerce.
The same dynamic can be applied at a national level – capitalizing on the industries and business processes that the nation has the most access to and expertise in.
In our market research on the AI ecosystem in India (linked above), we argue that India should be leveraging its position as the “back office of the world” – and turning more of its business process outsourcing and IT services work into high-value AI applications, not merely low-cost labor.
Almost any nation can think through the same data dominance dynamics and consider the policies and incentives it can use to provide its most important sectors with the best chance of growing – and having a prominent place the globally competitive AI ecosystem.
AI for Strategy Leaders
The AI Opportunity Landscape is the flagship market research service at Emerj Artificial Intelligence Research. It is the only comprehensive market research service designed to map the private sector AI ecosystem, and the known AI deployments of governments and global organizations – ranking and scoring AI applications by Evidence of ROI, Ease of Deployment, and other strategic factors.
Are you developing an AI strategy, or trying to decide the AI applications with the best near-term ROI for your organization? Watch our 2-minute video on the AI Opportunity Landscape services here.
Header image credit: UNICRI / James Hardy