The retail industry collects massive amounts of data every day, and this makes its key processes ripe for automation with machine learning. Along with the manufacturing sector, the retail industry likely stands to benefit the most from one particular AI technique in the next few years: machine vision, also known as computer vision.
Machine vision gives computers the ability to “recognize” objects in images and videos. With applications in security, shopping, driving, and more, the average person is likely familiar with machine vision use-cases like in-store cameras, self-driving cars, and smartphone apps that allow people to take pictures of a product and find it online.
The Emerj AI Opportunity Landscape in retail reveals that 43% of the AI vendor solutions available to brick-and-mortar retailers involve machine vision. In this article, we discuss two machine vision use-cases in particular:
- Automated Checkout: Allowing customers to pick up items in a store and leave without having to check out at a register or kiosk
- Inventory Management: Alerting store employees when items need to be restocked
We begin our exploration of machine vision in retail with how it could be used to automate the checkout process.
Automated Checkout
Some retailers are attempting to use machine vision to automate checkout and reduce the need for cashiers and checkout kiosks.
Examples of Automated Checkout
- Card Swipe to Enter: One example of this is in automated stores that require a card swipe to enter. A machine vision algorithm would then track the movement of the person who swiped their card, correlating the person’s body and movement with that card. In this case, a customer might be able to pick up the items they would like to buy and allow the store to charge their card automatically.
- Facial Recognition: In some rare instances, stores require customers to first register their face with the store online. Their face is also attached to a credit card on file. Then, they can have their face scanned by a facial recognition software before entering the store. As a result, the customer can pick up items in the store, walk out with them, and have their card automatically charged.
- Detecting Checkout Readiness: In stores that do not require a card swipe or card on file to enter, machine vision could be used to detect when a customer is standing at a checkout terminal and automatically start the payment process.
The short, 1-minute video from AI vendor AiFi illustrates how machine vision-enabled automated checkout could work:
Inventory Management
Retailers could also use machine vision for managing inventory. Like automated checkout, retailers could attach cameras all along the shelves in their stores. They could also deploy robots that travel down isles and scan shelves, as Walmart did in 2017.
Below is a picture of one of Walmart’s robots scanning a shelf:
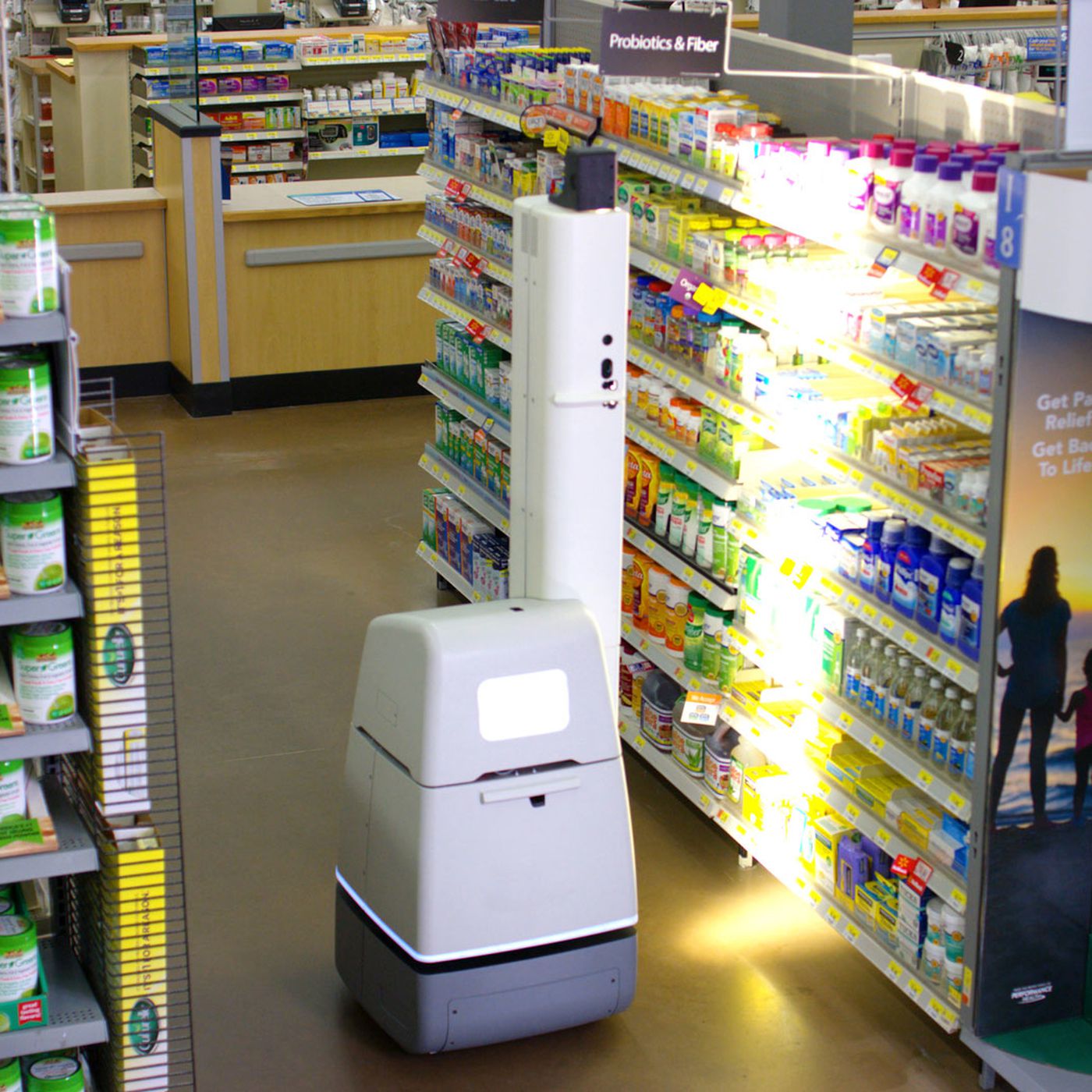
Retailers could train these machine vision algorithms to detect when certain types and brands of products are picked up from the shelves. Instead of charging a customer’s card, these systems would instead alert personnel in back rooms to restock the shelves with the appropriate products.
Such an application has the potential to increase revenue by reducing the amount of time a shelf is without stock.
The Challenge of Applying AI to the Physical World
Installing a machine vision-based camera system within a retail store can be a challenging endeavor.
A retailer would need to set up cameras at multiple points along every shelf in the store. The machine vision algorithm behind the cameras would need to be trained to recognize each product the store sells down to the brand label.
It’s not enough to train an algorithm to detect when a customer picks up a pack of paper towels, for example; the algorithm needs to detect when a customer picks up a pack of Brawny paper towels versus when they pick up a pack of generic store-brand paper towels.
Herein lies the difficulty in applying artificial intelligence to the physical world: physical space is subject to change in unexpected and subtle ways, such as location and lighting.
As an example, say a large grocery chain wants to train its machine vision algorithms to recognize when customers pick up a gala apple versus when they pick up a Honeycrisp apple.
These apples look similar, but they can vary in price. An algorithm that intends to recognize the slight physical differences between these two apples needs to be fed thousands of images and video clips of people picking up these apples in different lightings (including at different times of day if the apple display is near a window) and at different levels of foot traffic in the store (people might be blocking the camera’s view of the display).
Even after all of this, the algorithm may still struggle to recognize the differences between these apples in the split seconds before a customer picks it up, puts it into a bag, and places it in their cart.
Further complicating this is the tendency for customers to place store items on shelves and in displays they aren’t intended to go. The grocery chain may want to train its machine vision algorithm to recognize apples picked up from one display as gala and another display as honeycrisp. Although this might be more technically feasible, it means that customers may be able to trick the algorithm.
They could put a honeycrisp apple in the gala display, leave to continue their shopping, and then come back and take the honeycrisp apple they left from the gala display, thus tricking the algorithm into believing the customer took a gala apple. The customer would then pay less for the honeycrisp apple they actually took.
Adopting these solutions could provide cost reduction benefits to retailers, but if these systems fail to work a certain percentage of the time, they could have trouble delivering an ROI.
Emerj for Retail Companies
The retail and eCommerce industries were among the first the adopt artificial intelligence. Retail leaders use Emerj AI Opportunity Landscapes to determine where AI, including machine vision, can be used at their company to deliver the highest ROI. AI Opportunity Landscapes also help retailers assess the AI vendors in their industry, allowing them to select partners for bringing AI to life at their companies and potential acquisition targets.
Header image credit: Deal Street Asia