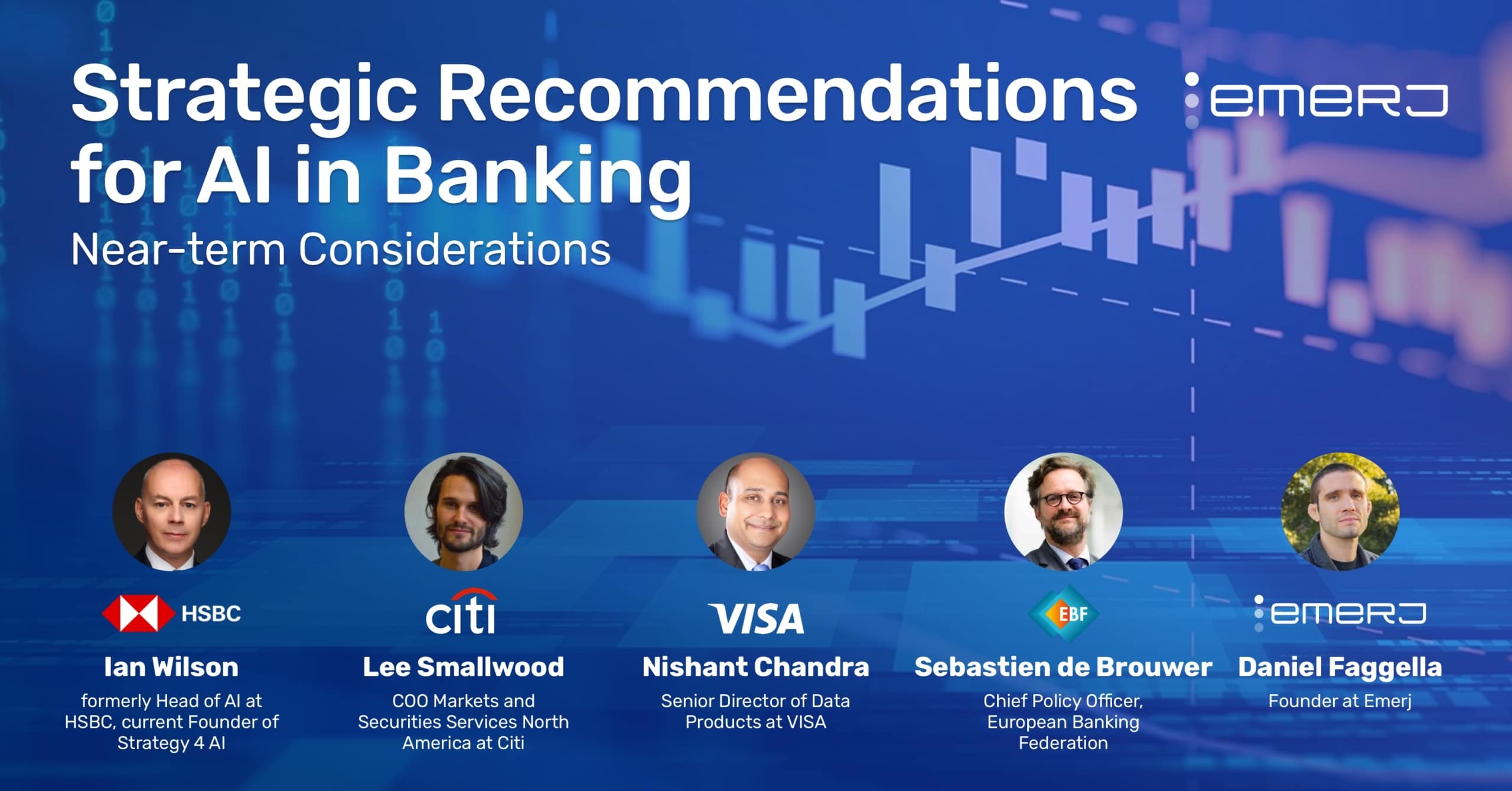
Four months ago we launched our AI in Banking podcast where we covered some of the most critical topics related to AI adoption and implementation in banks and financial institutions each month. Our series was based on interviews with AI industry experts, many of whom also shared their valuable insights during our first comprehensive banking research project, the AI Vendor Scorecard and Capability Map.
For the fifth month, we reached out to the research advisors who had a hands-on role in helping us with our research with the aim of having them speak directly to banking leaders to help them understand how to prepare for AI disruption in banking. Our research advisors have real-world business experience in AI projects working for large banks and financial organizations.
In this article, we aim to combine the insights we gained from our interviews with these advisors, along with our consistent research in the banking and finance sector over the past year to help break down strategic recommendations for banking leaders when it comes to preparing for AI implementation. Business leaders at large banks and financial institutions might find the themes presented in this article helpful in staying on the right side of AI innovation presently and in the future.
We breakdown the strategic recommendations and insights gained by analyzing the three interviews we conducted this month for our AI in Banking podcast into the following sections:
- Attracting and Retaining AI Talent in Finance
- Embracing AI at a Leadership Level
- Preparing for a Business Model Pivot
Special thanks to our interviewees:
- Ian Wilson, former Head of Artificial Intelligence at HSBC
- Lee Smallwood, COO Markets and Securities Services North America at Citi
- Nishant Chandra, PhD, Senior Director of Data Products at VISA
- Sebastien de Brouwer, Chief Policy Officer at European Banking Federation
You can listen to our full playlist of episodes in our “Strategic Recommendations” playlist from the AI in Banking podcast. This article is based in large part on all three of these interviews:
Subscribe to the AI in Banking podcast wherever you get your podcasts:
We begin our analysis with how banks should go about hiring and retaining AI teams and how they can develop a culture of innovation across their organization.
Attracting and Retaining AI Talent in Banking
Attracting AI Talent
According to Smallwood, the first step to building a functional AI team might be is attracting skilled data science talent. C-level executives at large banking and finance firms need to understand that hiring data scientists is a challenge due to the small size of existing AI talent pools.
What this means is that data scientists are highly sought after by technology companies such as Google, Amazon or Facebook. Additionally, these companies are often working on more interesting, complex AI projects that have a more tangible business impact; this can be more attractive to AI talent than the kinds of projects banks require them for.
Smallwood discusses how banking business leaders can adapt their hiring strategies to attract skilled data scientists when he says:
This really comes down to building relationships with universities and building pipe-line industry collaboration projects. Not to say that this can’t happen at more senior levels. It has to happen at the senior level, but it might be more about building the talent pipeline. The impact that a senior leader from a bank talking to university students about what kind of opportunities they might have in banks is underappreciated right now. Banks need to attract students by making them aware of the interesting industry problems they can work on.
Data science and data engineering are skills that require a high level of technical skill and time to learn and apply effectively. Banks might need to hire AI talent for these roles by identifying how good potential applicants are at working with several different kinds of data with varying volumes and data generation rates. Dr. Rajkumar Bondugula, Principal Data Scientist and Senior Director at Equifax, gives an example of what kind of data challenges banking leaders can expect:
Business requirements have dramatically changed over the past few years. A whole new set of tools to manipulate such large data, like Spark and Hadoop, are needed. A whole new set of skills is needed to just deal with the volume, variety, and velocity of data itself.
An example of high velocity data application would be closer to real-time data, like customer interaction data, which needs to be handled and considered for business decisions as it’s coming in (such as analyzing past user behavior on different communication channels such as phone, email, messaging or the website and use predictive analysis to provide better product recommendations.
Retaining Talent
Even when banks manage to hire skilled data scientists, retaining them is another challenge because other firms will attempt to poach data scientists with more money or more interesting challenges.
Newly hired data scientists will also need time to increase their knowledge about existing systems at banks and finance firms. Existing IT employees at banks are probably the ones that understand the internal data and workflows the best. Banks need to identify a way to effectively use this institutional knowledge along with the skills of data scientists to build AI systems that might give them any benefits. Bondugula added his viewpoint about retaining data scientists:
Most people (data scientists) nowadays are changing jobs within 2 years of starting with a new company. Conceiving a (data science) problem to putting it into production, in my experience, is a 3-year journey in enterprises. Bringing in large groups of people does not make sense if, as a company, you do not have clear technical objectives – and an ability to retain data science staff.
Smallwood seemed to agree that banking leaders might need to have a lot of flexibility while dealing with how to retain data scientists. Firstly, they would need to develop their understanding of how AI is applied to businesses in order to give clear directives about data science projects. He gave the following tips for business leaders about how to retain data science staff:
Being flexible with people and having frank and honest conversations with data scientists is necessary. While identifying the problem that can be solved with AI, banking business leaders might need to leave room for creativity. For instance, NLP experts might want a different challenge and work within other aspects of machine learning and AI. It is really easy for C level executives in banks to talk about AI talent as a pool, but these are individuals and it might be better to take this on a case by case basis.
Handling Job Disruption
The integration of data science teams will likely cause a disruption in existing workflows within a bank. The AI talent in these teams needs to work closely with subject-matter experts who feed the data scientists relevant business problems and existing IT teams that can take AI systems developed by the data science team and integrate them into existing systems. This ‘connective tissue’ of teams that support AI teams on both sides is required.
Nishant Chandra was of the opinion that banks should have a firm pulse now and in the future about how AI will affect the jobs and functions in different departments, saying:
Many banking officials are fully aware that there may be significant job disruption as a part of the transition to AI, but might not want to go ahead and talk about this openly. Banks might be forced to become more efficient, to do best by their shareholders, they should have an idea about how to repurpose, and train talent to adapt to future AI circumstances. In order to stay in business, they may have to make drastic changes.
Some of the factors that banking leaders might need to think about while initiating measures to retain data science staff include:
- Fixing a clear organizational structure for the AI team between the data scientists, subject matter experts, and existing IT employees.
- Identifying the right business problems that are aligned with the overall business goals while still being interesting to the data scientists
- What Data infrastructural changes need to be deployed before any AI project can be feasible
- How to build a sustainable process for IT teams to support data scientists while still working with existing systems in the banks
Embracing AI at a Leadership Level
Understanding AI potential.
The decision-makers at large banks and financial institutions need to understand what to expect from AI adoption. For instance, how to assess cost and resource requirements. Nishant Chandra puts the onus on banking leaders to understand the potential of AI:
When the leader looks at the outcome of AI system when the system has become leaner and asks what next? The first questions they must ask themselves should be – How can we create actionable benefits out of this AI project. How do I hire people who can act on this action? How can we upgrade the skills of existing team member who can tell me the pros and cons of this project.The real impact of AI projects might not lie in saving man power, but rather in providing new opportunities and it is the onus of the c-suite in banks and financial institutions to act on this opportunity and build new teams
Banking leaders need to access what commonalities exist between the various possible benefits that can be gained through AI and the current business challenges where the bank might need or benefit significantly from such projects. In some cases, leaders might find that in order to gain experience with an AI capability and develop the level of skill in that particular application (which will give the largest benefit in the long term), banks might need to start with smaller pilot projects that might not be the most profitable in the immediate sense.
Proper Expectations for AI
Banking leaders need to understand the short-term and long-term AI Investments they need to make, including what ROI they can realistically expect from AI and over what time frames. In order to do this, they will also need to understand what critical capabilities are being touted as beneficial by AI vendors and other banks and financial institutions.
An effective way to understand these capabilities is to study existing case-studies from vendors and banks and imbibe what is possible with AI in different banking applications. The next step is to identify the business challenges within the bank in which these capabilities can be applied. What is critical to understand is that AI is often thought of as a magic solution that can solve almost any problem, which it certainly is not.
But from our research, we believe that even the Silicon Valley tech startups with a high level of AI skills also commonly lack the understanding of how to develop a sustainable AI team structure, and executives in these tech firms also seem to be struggling with setting expectations for what AI can and cannot do. Tried and tested decision-making frameworks for what type of benefits can be gained from a level of AI investment do not exist at the moment.
According to Ian Wilson, setting the right expectations from AI projects goes hand-in-hand with understanding the capabilities of AI. C-suite leaders at banks are largely not talking about AI from this perspective today. Wilson provides further detail to this concept when he says:
The key question you’ll be hearing at the C-suite in a bank particularly is ‘What’s next? What comes next? Where is the ROI?’ And often there is no answer to that question. There is no ‘what’s next’ because there was nothing strategic put in place. It was just throw some mud at the wall and see what sticks.
Embracing Risk
According to Smallwood, the challenges in developing a culture of innovation gets more challenging the larger an organization gets. But he lists out some common tenets that apply to both large and small banking and financial institutions when it comes to succeeding in AI projects. He stated that being comfortable with failure might be one of the biggest challenges for large banks and financial institutions when it comes to AI projects.
What business leaders in banking need to understand from Smallwood’s statements is that AI projects are inherently going to be ridden with some sort of failure. The environment within data science teams should be one that welcomes creativity while knowing that failure is going to be a part of this development process. This doesn’t mean that banks should be completely ok when every aspect of an AI project is going wrong, rather that creative data scientists who took an educated risk aren’t reprimanded for a few small errors along the way. He explained it as follows:
In my experience this is usually different for each institution and must come from the leaders of each division. Successful business leaders will be those that are self educated in AI, who have taken an interest and are open to new ideas. This doesn’t mean that the bank managers need to learn to code tomorrow.. But understand what is possible with the technology. Corporate innovation teams have a role to play as well. These groups have tie ups with external startups and venture community that helps in building a culture of innovation as well.”
Chandra also mentioned how business leaders in banking can tackle the associated risks that come with AI projects he stated:
“If we solve for the right problems the risk might be reduced because you might see benefits at some point. In banks and finance the benefits are usually pretty fast. For example in the insurance industry for the longest time was about assessing risk such as Insurance firm can insure shipping company and hope that no oil will be spilled or no ship will sink.
Insurance firms are leveraging AI to find out what something is happening and proactively mitigate that risk. This can be through sensor data on the ships to identify if all the ship parts are working well. They can then have a handle on what leads to risk allowing them to find a way to mitigate it.”
Preparing for a Business Model Pivot
Large banks are organizations that have existed for a long time and bankers are usually risk-averse and set in traditional ways of doing things. In this environment, banks now need to figure out how they can make innovation a part of their business culture. Chandra seems to suggest that the fact that these banks have survived for such a long time is by inherently having a culture of transformation He explain this with several examples:
For example GE build the six sigma model which is in business process which helped them adapt to a transformation. Microsoft Pivot themselves to cloud platforms, apple pivoted on people where they brought in design thinking is all processes. Transformation in processes, platforms and people has already happened and transformation will happen in AI.
Nishant went on to say that bankers might need to help develop AI teams that can create innovative ways of finding the impact of AI projects within the bank’s existing business challenges. Bigger finance firms who want to do a better job of pivoting and taking advantage of change seem to be facing challenges in the speed of implementation of AI systems into existing banking workflows.
What this means is that taking an academic example where AI is being applied to a particular problem and then transferring that system to solve a problem for the industry is fraught with challenges. But Nishant believes that this issue of agility in implementation will be solved very soon and when the knowledge to apply this in a professional setting is realized will be a flood of new AI applications.
De Brouwer sums up the main themes from throughout this past month’s series well:
It’s certianly challenging for banks…I think we have to acknowledge that AI is still a bit in its infancy and so there are a lot of uncertainties…I think thre is also a lot of uncertainty still on how much clients theselves wil adopt and at what speed AI technology…Another challenge for banks is you need to have the right skills to develop an AI solution. It’s good to have data scientists…but you need to make sure you have also people educated or trained to support aterwards the underlying infrastructure of AI…so how to build the infrastructure ot make use of the full AI in the bank.