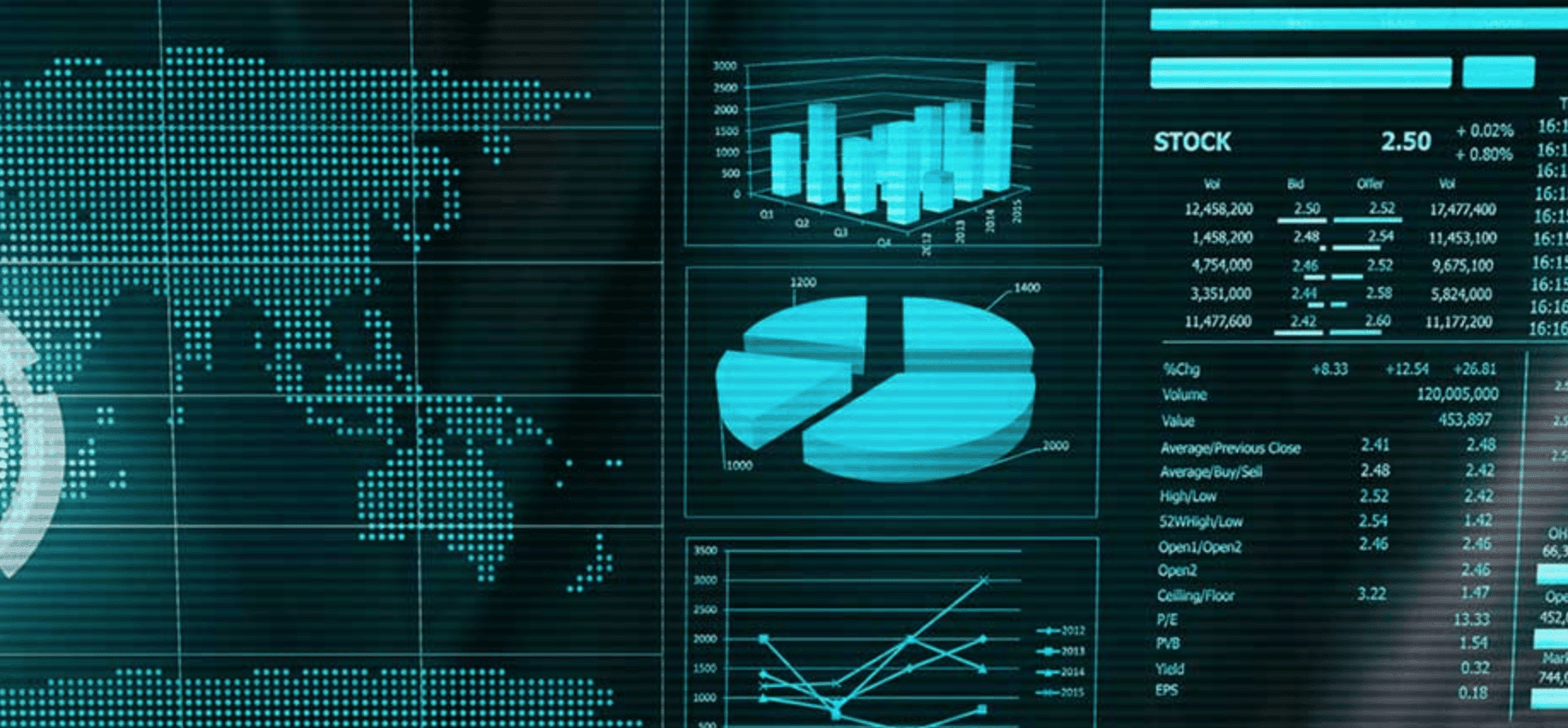
Episode summary: This week on AI in Industry, we speak with Equifax’s Dr. Rajkumar Bondugula about how the dynamics, composition and requirements of the data science team have evolved over the years. Raj also shares valuable insights on how to build a robust data science and machine learning team, use its collective intelligence to solve problems, and retain the team by engaging them with the right problems they expect to solve.
Subscribe to our AI in Industry Podcast with your favorite podcast service:
Guest: Dr. Rajkumar Bondugula, Principal Data Scientist and Senior Director at Equifax
Expertise: Machine learning, big data, data mining, parallel computing
Brief recognition: Dr. Rajkumar Bondugula serves as the Principal Data Scientist and Senior Director at Equifax. He has a doctorate degree in Computer Science with a specialization in Machine Learning from University of Missouri-Columbia. Dr. Raj held previous data science leadership positions at Sears Holdings Corporation and Home Dept.
Interview Highlights on Hiring and Retaining a Data Science Team
Listed below are the most important points Raj talked about in this interview:
(Note: this is not a word-for-word transcription but certainly close. The answers are paraphrased, without taking the most important elements out of context. For the full interview, please listen to the podcast embedded at the top of this article.)
How Can We Compose a Winning Data Science Team?
We need individuals who can work with variety, volume and velocity of data. Business requirements have dramatically changed over the past few years. In the past, we looked at data and analyzed what had changed in the past. Now, we are looking to the future with predictive analytics and ask what will happen. We are predicting alternative futures and we pick what is best for us. What should we do with this information? The problem here is that same data cannot answer these questions.
Varieties of data have significantly increased. For example, in eCommerce, we can analyze the past user behavior and use predictive analysis to provide better recommendations. A log that captures your entire activity answers a whole lot of questions. Which brand are you loyal to? How long did you take to decide before buying a particular product? And based on this data, we can make better recommendations. For that, we have to extract information from a variety of different sources.
Technology for extracting features from big data is different than that in traditional RDBMS, where you have structured data with rows and columns. The nature of the incoming data is broader right now and different skillsets are needed to extract information out of this variety of data sets, such as image data, numbers, etc. The volume of data in RDBMS is just a couple of millions of rows. It has exponentially expanded. We are talking hundreds of terabytes of data.
So, we can no longer use traditional RDBMS or a single computer. A whole new set of tools to manipulate such large data, like Spark and Hadoop, are needed. A whole new set of skills are needed to just deal with the volume, variety and velocity of data itself. An example of high velocity data would be real-time data, like streaming data, weather data, etc – which needs to be handled and considered for business decisions as it’s coming in (i.e. Weather data must be taken into account in real time if we’re making decisions about where to route delivery trucks or planes).
What Are the Skills Necessary for a Well-Rounded AI Team?
An example of different skill sets: For distributed computing, handling distributing storage, etc., we need big data engineers who are good with distributed systems and computing. We might need different big data engineers for manipulating information from streaming, real-time data.
With the technology becoming more standardized than before, the skill sets are transferable between industries. Training companies have cropped up to train workforce in new technologies. For example, if you know how to use SQL, can you do what you do with SQL for a much larger data set (in the distributed database) is the question. You take the skills you already have and apply those skill sets to work on larger data sets. In fact, this means that when you use the same tools for different domains, you understand what tools are better in which domain.
Where Can Data Science Talent be Found?
It is very difficult because the right person you have in mind for the job already has a lot of offers, and it is a bidding war, really. Not good dynamics. One way to make sure that more such people have the right skill sets you have require for the job is to consult with the Industry Advisory Board in the universities and tell them that this is our needs. Another way to do this is we talk to the students and inspire them early on to learn new technologies and develop the right skill sets.
Yet another way is, we transition and train the existing employees in new technology. If you are a traditional Java/SQL developer, you can be trained to do Java/SQL on Spark. We need companies to build training capabilities to retain their existing workforce. There are certain areas where experience definitely matters.
Existing employees know the workflow. They know who manages the data, who manipulates the data, who inputs the data, who owns the data, who needs to make sense of the data, etc. This really matters because they understand the big picture. This is where institutional knowledge comes handy.
How Can We Retain a Data Science Workforce?
Most people nowadays are changing jobs within 2 years of starting with a new company. Conceiving a problem to putting it into production, in my experience, is a 3-year journey in enterprises. Bringing in large groups of people does not make sense if, as a company, you do not have clear technical objectives – and an ability to retain data science staff.
Maintaining a balance in the data science team architecture is important. There are many factors to be taken into consideration here.
For example:
- Who would run the team?
- Does the team have enough work?
- Is the team being overworked?
- Are they being given the right problems that are aligned with the companies’ business and technical goals?
- How about the organizational readiness?
- Has your company transitioned to a centralized database?
Your admin team is also an important factor here. Mature support is needed from the admin team for the data science team.
Subscribe to our AI in Industry Podcast with your favorite podcast service:
Header image credit: NE Big Data Hub