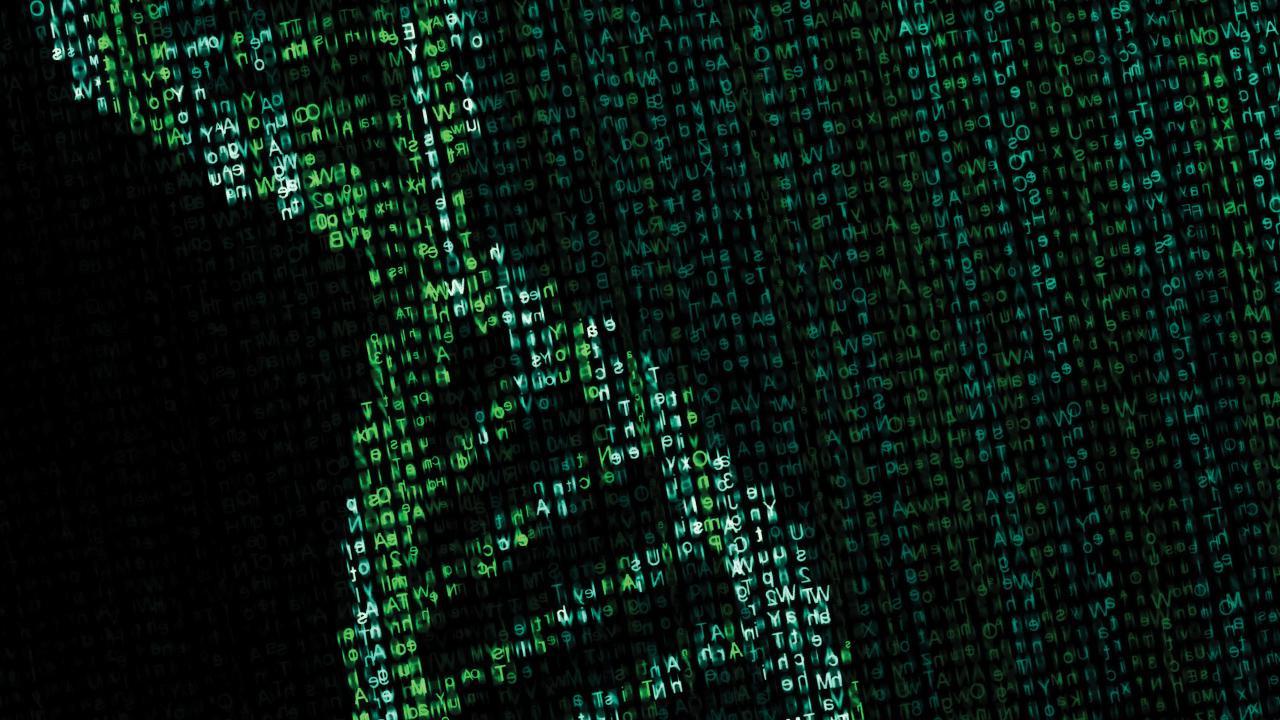
Episode Summary: This episode explores the ways in which artificial intelligence has the potential to revolutionize the field of medicine. This week’s guest, Turbine.ai’s Dr. Kristóf Zsolt Szalay speaks to this topic, discussing research that hopes to create automated learning networks and algorithms designed to predict the development of human cells in response to drugs. This technological innovation would make it possible for near-instantaneous simulations to be run, allowing optimal combinations and optimal doses of drugs to be pinpointed and distributed to patients.
Expertise: Bioinformatics, Biomedical artificial intelligence
Brief Recognition: Dr. Kristóf Zsolt Szalay is the founder and current Chief Technology Officer of Turbine, Ltd. Between 2009 and 2016, he was a research associate at Semmelweis University Department of Medical Chemistry, Molecular Biology, and Pathobiochemistry where he won the Semmelweis Innovation Prize of 2015 for his work—work that a year later would be the foundation for Turbine.
Current Affiliations: Founder and CTO of Turbine, Ltd.
Big Ideas:
The long term goal would be to have a system on hand that can simulate how a single cell would respond to specific chemicals, and whether its response would be to die off or replicate. Using comprehensive machine learning networks and algorithms, simulations could be run that would offer personalized predictions for individual patients, eliminating the need for trial-and-error, and allowing cells to be treated with the optimal dose of the optimal combination of chemicals, a combination that can be predetermined quickly and easily.
To do so, Turbine intends to collect the data on how certain drugs affect different kinds of cell lines, and use this data to create a neural network. They plan to build a model of the human cell, one that allows a clear reading of the signaling part of the cell, which determines whether the cell will live, proliferate, or die in response to its environment.
Their diagram begins with a general human cell, but contains multiple layers of data in order to make it specific to any kind of tissue. The general human cell differs based on a person’s unique genetic code, which determines which proteins are more active than others within the cell. Furthermore, cells that make up one part of the body carry proteins that are not present in cells that make up other parts of the body. Using their diagram and its multiple layers, they can simulate a cell that is specific to a certain kind of tissue.
The artificial intelligence being used is best thought of as similar to how a car’s GPS works: the ability to run and depict a simulation in a second. In this case, rather than a map and a route, the simulation is of a cell and its reaction to chemicals. The artificial intelligence makes an educated guess as to which combinations of drugs in which doses would be feasible to use and tries to find the optimal combination in the optimal dose.
Turning Insight Into Action:
Turbine aims to match patients to the drugs they need, drugs that cater specifically to the design of their cells. Being able to administer the exact amount of the exact chemical to a cell in order to treat it would revolutionize medicine, allowing for prescriptions of extreme, unprecedented precision, specified to the needs of the patient. The ability to run simulations digitally would also reduce the necessity for tests in labs, allowing easy insights to be gleaned on the viability of certain chemicals on cells.
Interview Insights with Kristóf Zsolt Szalay
Daniel Faggella [Dan] (1:35): What is currently possible in the domain of replicating biology in its response to chemicals or drugs?
Kristóf Zsolt Szalay [KS]: The big area here is predicting the response of certain cells to drugs. What actually can be done here is to get a lot of data on how a certain drug will affect different kinds of cell lines and build a huge neural network from this kind of data set. Basically, you use these huge machine learning networks and algorithms to make some kind of prediction from it. In many cases, it actually works quite well for critical areas so that you don’t really need to go and see what happens inside a single cell.
For example, in the case of cancer, every single type of cancer is different. Cancer occurs because something is wrong in the signaling pathways inside the cells. For cancer to develop, you need multiple different mutations. Every kind of cancer is a bit different, so there is not a solid single huge data set usable for machine learning. You won’t be able to easily get that kind of insight.
So, what we do is build a model of the human cell, and mainly the signaling part of the cell, which decides if the cell will either live, proliferate, or die according to inputs from the environment. In doing this, you can keep track of the wiring diagram of a cell so we can give a lot of prior data just based on the decades of knowledge available from biochemistry.
Dan (5:12): How do you determine which cells to build replicas from, and how do you build a cell digitally?
KS: We have a diagram of a general human cell, which is the same as each and every cell for all of us. To make it specific to any kind of tissue, we add multiple layers of data. So this is akin to a wiring diagram of cells, and is based just on the biochemical knowledge of which proteins can connect to other proteins. It basically describes the possibilities, but these possibilities are then limited in individual cells. On one hand, everybody carries a different genetic code, which in turn makes some of these proteins more active than others and others less active.
That’s how we can create a model for a specific person or specific kind of cell line. On the other hand, the genetic code for the cells in your eyes, skin, and hair are basically the same, but to make them different is something called transcriptomics. There are some proteins that are present in the cells of the heart but are not present in the cells of the eyes, and this is another area of data that we can get. This is the second layer data used to customize our simulated cells. With these layers sandwiched together, we can give you a simulated cell that is specific for a certain kind of tissue for each patient.
Dan (7:50): Would this system simulate how a single cell would respond to specific chemicals and why it would be likely to die off or replicate?
KS: Yes, and in the long term that’s what we’re shooting for: to be able to give personalized predictions for individual patients. But I’d like to stress we are doing the opposite of that. We are not matching drugs to patients; we are matching patients to drugs. So what we usually have in our project is a compound and we have to test it through multiple cell lines or some kind of patient tissue so we can see what gives the best response.
Dan (9:08): Understood. So if a drug company doesn’t want to go through a gazillion lab tests they might first see how [their drug] performs under certain simulations under the circumstances in which we think it’ll have an effect. And from there, you’re able to tease out insights that might indicate it’s worth testing in a real biological model.
KS: What actually happens is that most of our projects are not compounds that require pre-testing, but many are in the clinical phase. The kind of projects we’re getting are [like this]: Take a drug. Let’s say it’s in phase two, and it just failed one of phase two’s trials. [Drug companies] will come to us and let us know that there’s a group of patients that didn’t respond [to the drug], but should have based on what happened inside of the lab. We will then build these simulated cells and run some predictions.
(Readers who are less familiar with the phases of clinical trials may want to review the FDA’s drug approval page, which includes a short video and article that explains the process succinctly.)
Dan (12:10): Why would AI be required to simulate how a cell would respond to chemicals? Where is artificial intelligence critical in this process, and how would you explain this to a business audience?
KS: The simulation part doesn’t use AI, and we don’t use the type of algorithm where AI is most used, the pattern recognition/neural network AI. We do use some kind of AI to setup the parameters for simulations, but that critical part is search AI, which is different from standard AI. It’’s not a machine learning kind of AI; it’s more similar to what’s inside of your GPS.
Basically, what the AI does is allow one simulation to take a fraction of a second to run. In that way, the AI is a layer above the simulation, and it guides that simulation. Basically, it makes an educated guess of which combinations in which doses would be feasible to run, and it tries to find the optimal combination in the optimal dose.
Dan (15:50): What does it look like five years in the future? As we become more and more accurate in replicating real biological cell responses to different chemicals, what ramifications and implications does this have? And what difference does this have in the realm of healthcare?
KS: In a decade, if we understand how cells work, which is the holy grail of biology, I think it would kickstart some sort of biotechnology revolution similar to what happened when computers and the Internet came about. We can see that something big is coming, but we have no comprehension of the sheer size of the impact.
Related Articles on Emerj
Our editorial purpose here at Emerj is to provide the most insightful, most useful AI insights for business leaders. Our audience depends on us to make strategic and procurement decisions every day, and we’re proud to provide the objective data, thorough research, and expert insights that they need.
This interview with Turbine.ai is literally the tip of the iceberg when it comes to our coverage of AI in biomedicine. See some of our other popular interviews and articles below:
- Article: 7 Applications of Machine Learning in Pharma and Medicine
- Article: AI in Pharma and Biomedicine – Analysis of the Top 5 Global Drug Companies
- Interview: Qrativ’s Murali Aravamudan on “What’s Possible” for AI in Drug Discovery
- Case Study: Pharma Giant Understands the Consumer Concerns by Mining Online Forum Data
Header image credit: sciencemag.org