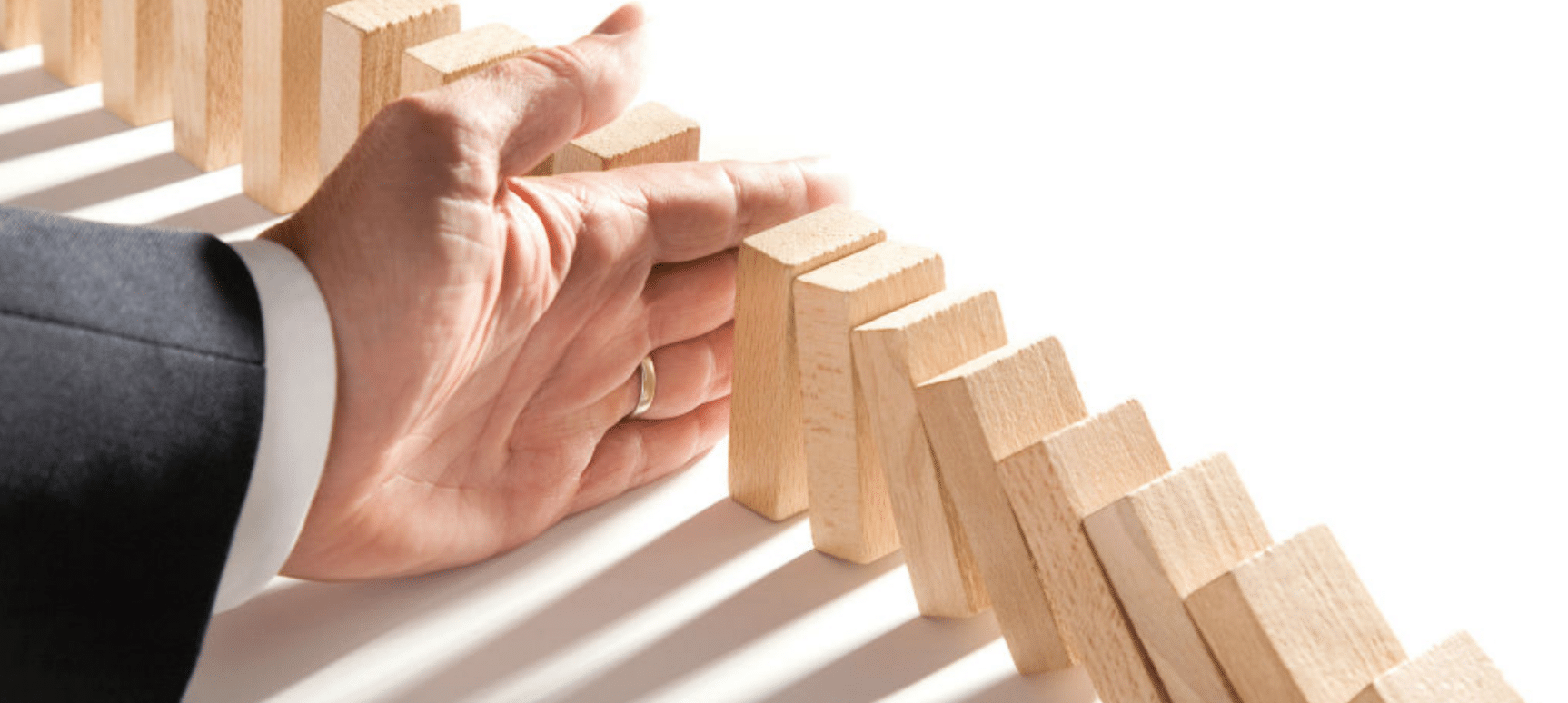
Episode summary: In this episode of AI in Industry, we speak with Dr. Sanmay Das from the Washington University in St. Louis about risk prediction and management in industries like banking, insurance and finance.
Sanmay explores how banks and other financial institutions are improving risk and fraud prevention measures with machine learning. In addition, he explores the ramifications of improved fraud detection in the coming 5 years ahead.
Subscribe to our AI in Industry Podcast with your favorite podcast service:
Guest: Sanmay Das, Associate Professor, Computer Science at Washington University in St. Louis.
Expertise: Computer science, machine learning
Brief recognition: Sanmay earned an undergraduate degree in computer science from Harvard and went on to do a Ph.D. at MIT in the same field. Sanmay went on to be a Postdoctoral Scholar at the University of California, an Assistant Professor of Computer Science at the Rensselaer Polytechnic Institute, and an Associate Professor of Computer Science at Virginia Tech from 2006 to 2013. He now serves as an Associate Professor of Computer Science and Engineering at the Washington University at St. Louis.
Big Idea
More data source and more “layers” of information are making financial risk more accurate in real-time.
Sanmay explains that applying ML techniques to the individualized customer data combined with insights from more traditional broad institutional data, AI can today identify strategies that these banks are using to manage credit risk and report on the different outlooks that banks may have on what kind of risks pose a threat to them.
Sanmay adds that today AI is being applied to better assess the risk at the baseline (individual credit risks like with credit cards for banks) that can then become part of these more complex instruments (like measuring the credit risk of an entire economy). He says that as the ability to assess the risk at the individual components of these portfolios gets better, AI will get better at predicting the overall systemic risk, too.
He cites a number of examples:
- Using customer data like the topline figures on monthly statements (outstanding balance, what was previous payment how many days late did you make a payment) and cross-correlating this with data from standard credit bureaus, banks can create datasets for each individual to assess their credit risk with many individual data items recorded per month.
- He cites another use case where a particular bank collaborated with experts in finance and machine learning to assess the bank’s credit risk portfolio and enact an “active management” of credit risk strategy. In this use case, banks may be able optimally to mitigate risk from credit card holders by using machine learning to identify when to lower or raise an individual’s credit line – calibrating the bank’s risk tolerance quickly as the user’s behaviors change.
- Sanmay also believes that incorporating macro-factors (like the broad institutional data from banks) along with the more personalized data tends to improve prediction accuracy for many applications including credit card risk management. For example, knowing what the house price index in a particular area can potentially help predict the levels of credit card default in that area.
Sanmay agrees that the availability of a lot of big datasets today in finance today has meant that the ROI for applying ML is quantifiable. Yet he warns that such a credit risk platforms are not transferable from bank to bank since there are individual portfolio effects and factors like the targeted clientele can also make a difference in the optimal portfolio for each individual bank.
This probably means that it would be challenging for any single vendor to acquire enough data to “solve” the credit card risk problem for any given bank (given the differences in geography, in the type of clientele, in unique risk factors for different banks or banking divisions). This may mitigate the “winner takes all” dynamic that we’ve explored in previous AI investor interviews (notably, our interview with Gary Swart).
While the use AI in finance seems to be growing, Sanmay cautions about the ethical considerations of such technology in the future seeing as this technology could affect societies in ways which don’t understand. While we’ve seen unemployment ranked as the most likely societal risk of AI in the next 20 years (from our consensus research with AI academics), there’s certainly risk in “tinkering” with the financial markets as well.
For example, an AI might choose to cut the credit line for customers who fit a particular type of profile and yet that may not be in alignment with the overall societal goals. Conversations about incorporating the values of fairness and simultaneous business value while using such technology are vital according to Sanmay.
The topic of machine learning “bias” is a hot-button issue in many industries, and it’s one that can’t be avoided. Machines assess reality the same way we do: By seeing many instances of reality, and adjusting their behaviors based on predictions from past experience. In this respect, “bias” and “intelligence” are – in many regards – one in the same.
Interview Highlights with Sanmay Das from Washington University in St. Louis
The main questions Sanmay answered on this topic are listed below. Listeners can use the embedded podcast player (at the top of this post) to jump ahead to sections they might be interested in:
- (2:40) Looking back at the financial crisis, you were involved with a project that was aimed at measuring the credit risk in the country immediately after; what were the takeaways from that project for assessing credit risk today?
- (7:35) How did broad models for credit risk work traditionally?
- (17:50) what was the desired outcome of this project and why was it handy for the regulators?
- (23:07) In the future when Ai for risk management becomes commonplace among banks and other financial institutions, are there any positive and negative consequences to this?
Subscribe to our AI in Industry Podcast with your favorite podcast service:
Header image credit: Procurious