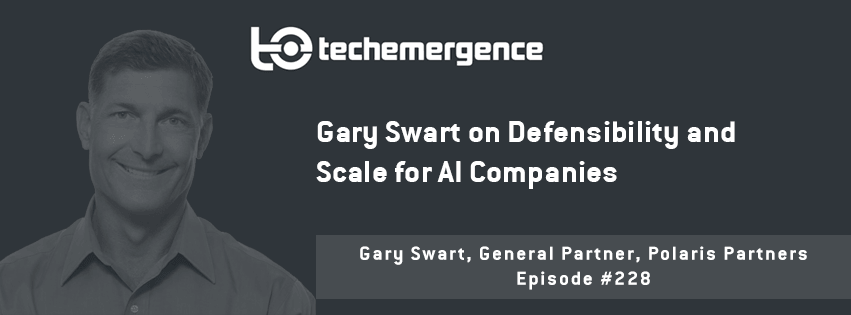
Episode Summary: This week we interview Polaris Partners‘ Gary Swart, who gives his perspective on companies that are doing “AI right” i.e. laying strong foundations for using AI applications optimally. Swart provides valuable examples of how he’s seen companies use AI as a tool to build more defensible and durable business models in an increasingly competitive landscape. Getting an investor’s perspective in AI is always a good idea for companies looking to raise money, particularly when it comes to understanding the types of AI trends that excite VC’s. Even more broadly, an investor’s perspective can point to emerging factors in how AI is going to impact a particular industry, shining a light on industry developments and commonalities that matter for companies across industries who are leveraging an increasing number of AI tools and applications.
Expertise: Future of work, entrepreneurship, online marketplaces/platform strategy
Brief Recognition: Until April 2014, Gary Swart was the CEO of oDesk, the predecessor to UpWork and the world’s largest online workplace. Swart guided the company to industry-leadership and through a merger with Elance, while serving as a leading voice for the future of work and the emerging online work industry. Drawing upon his unique position as a witness to disruption in work, he provides mentorship to entrepreneurs and growing startups on how to craft a successful path — giving more than 30 fireside talks last year alone. Prior to oDesk, Swart’s career grew from early entrepreneurial ventures to a top executive position at IBM, and he has managed thousands of people during his career on teams ranging from small and entrepreneurial to large teams for global enterprises. He holds a BS in Business from University of Maryland College Park.
Current Affiliations: General Partner with Polaris Partners
Big Ideas:
1 – Building a Defensible Moat in a Competitive Market
The last decade’s phenomenon of SaaS, a primary investment for Polaris Partners, has unearthed two increasingly valuable business assets—unique data sets and actionable insights drawn from unsupervised learning methods. An increasing number of companies are using AI software to collect hordes of observations of user and consumer behavior i.e. a proprietary data plume, to which no other company (other than the vendor) has direct access. ML algorithms are able to draw correlations between variables and drive strategic action in ways that weren’t possible just a few years ago. For example, prescriptive analytics insights might point to an increasing likelihood of closing a sale in Atlanta when the Braves win a baseball game the night before. This data can then be incorporated into simple but more effective sales strategy for sales reps—who to call and when to call them. Swart uses several examples in sales to illustrate how these SaaS approaches are helping to define new best practices in sales and other industries.
Turning Insight into Action:
Imagine you could put the capabilities of data scientists into the hands of any business professional, machine operator, etc. in order to improve speed and quality of services and production. Businesses using SaaS and similar technologies can now implement this competitive advantage by paying SaaS vendors for access to insights and best practices gleaned from vendors’ aggregate data sets across an industry. Using these services and paying for access to this type of data may soon become industry standard in staying ahead or keeping pace with competitors. If you’re on the vendor side, providing these data-based services at scale becomes an invaluable asset that can’t be easily matched.
Interview Highlights:
The following is a condensed version of the full audio interview, which is available in the above links on Emerj’s SoundCloud and iTunes stations.
(4:11) I can imagine that there might be a sort of common thread of where AI is actually driving value in the business in which you’re investing…is there a way to put that into a nutshell?
Gary Swart: Generally, data scientists fundamentally changing the future of development application and consumption; historically it was about aggregating, scrubbing, deduping, etc. and everybody claimed to be doing AI, but I think now what we’re seeing is the data is made actionable, and companies are developing effective methods for unsupervised learning. I think that’s what’s interesting now from an investment standpoint.
(5:17) I’ve heard of concepts of a proprietary data plume that often times is necessary for a company leveraging AI to be interesting, because otherwise the actions you can take based on your data are not that unique to what other people could do—does that plume idea resonate with what you’re talking about?
GS: It does indeed, we invest in SaaS companies with what I call predictive data in motion, and there’s not a SaaS investment we’ve made over the last couple of years that don’t have both a unique data set but also prescriptive elements to its core value.
…here’s a good example from our portfolio, a company called Inside Sales…they take the learnings from all of their customers, and those learnings benefit all of their other customers. So they have more than 100 thousand users on their platform, and they’re passively observing the behavior of sales results, so it’s a dialer that enables you to more effectively and efficiently contact your customers, and every time you contact a customer, the platform learns from that behavior and those best practices, and then that in turn benefits everybody else when making a call…
(9:05) My supposition is that in addition to how many calls were made, what were the roles of the people who were called, etc…all those different data points are maybe stacked on top of weather data, etc., all these things are getting pulled into this contextual data lake…so that these patterns can be coaxed out by ML.
GS: That’s exactly right. So when you can take the dialer information, you can marry it with what happens in the CRM system—Salesforce, etc.—and you know the outcome. So what happened? Did the sale get advanced as a result of that call? Take all the situational data, and then you marry it with all the other data that you can pull into the system, and now you can start to get prescriptive and predictive about what a rep should do…with a platform like this you can prioritize those calls, so they (sales reps) call them in the right priority order.
(11:21) It sounds like there’s a lot of contextual factors going into what’s sorted at the top; is this something that people can parse out? I know there’s perennial consideration with AI around this black-box concern…i.e. if it can’t say why, we’re just not going to do it…is it possible to figure out why these three are at the top as opposed to the ones I thought would be closer to the top?
GS: I think there is; reps can override it, which in turn creates more learning for the engine. A big thesis for us is the future of work, and it’s where is the world of work going. You think about automation, and the majority of manufacturing jobs in the U.S.—we didn’t lose them to offshoring or outsourcing, we lost them to automation…there’s also atomization, taking things and breaking them into smaller pieces, and then there’s augmentation, taking the resources that you have and making them more efficient and more effective. So in the case of Inside Sales, ML for predictability in sales forecasting predictability has an 80 percent accuracy rate, so the engine is getting good because they have so much data and so much usage over time, they’re seeing unprecedented capabilities here that sales leadership, and all the way down to the rep level, just haven’t had before…
(18:52) How do you see the dynamics we’ve talked about thus far altering in the decade ahead as these technologies become mainstream?
GS: I think if you look at the evolution, there’s sort of been major forces shaping the opportunities, starting with big compute, and then big data, and then smart algorithms, and now we’re even seeing smart voice. So big compute is by no means over, we’re very much on our journey, but the growth as the business there has been huge and this has enabled first IT transformation, then development transformation, and now cognitive business transformation, with AI and cloud, and I don’t see that going away.
(20:03) Is it possible that this data plume’s growing advantage, widening the moat so to speak, will almost be ubiquitous across industries? That it might be a little more “winner take all” across the board where technology is used—is this reasonable to think about?
GS: I think there’s going to be continued verticalization, but Bank of America estimates that $14 trillion will be the economic impact of AI (in 2020), and AI bots will power 85 percent of customer service by 2020, according to Gartner…so you start to think about automation and when will the robot take my job. Well for certain jobs, for the highly cognitive jobs, it’ll be faster than you think. The most known and repetitive task will be automated, then discrete and predictable tasks can be atomized, then the complex and ambiguous tasks at the top of the stack will be augmented with AI, but will soon enough move up that value chain.