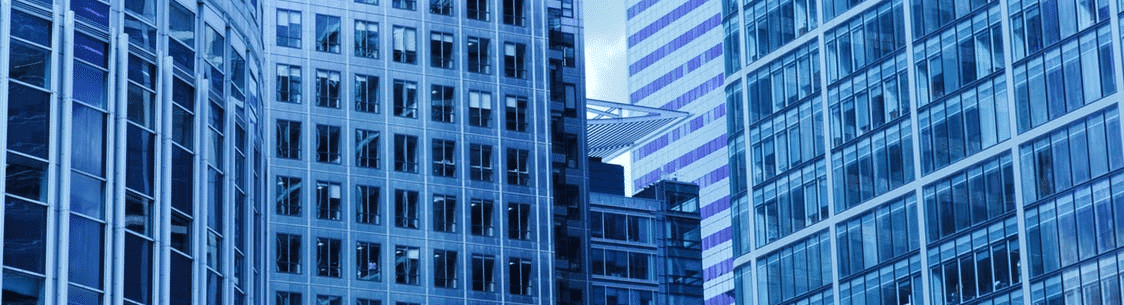
Busy and multitasking are understatements for today’s executives and entrepreneurs. Machine learning has the potential to help make businesses more efficient, competitive, and profitable, but learning how it works and finding the resources to implement this technology takes time. Where to apply machine learning when first getting started is dependent on a number of factors – industry, structure, current problems – but having an idea of which solutions have proved most efficient for others and derived maximum return on investment is a helpful jumping off point.
This is exactly why Emerj set out to ask the question:
“Which useful machine learning applications (industry-specific tasks such as: sales forecasting, fraud detection, etc…) do you consider to be the “low hanging fruit” for medium and large companies to derive value?”
We leveraged our hundreds of interviewees (on our podcast and otherwise), and reached out to other experts in the field, in order to gain perspectives from researchers and executives across the globe. We reached out to 30 experts and asked them to share their thoughts on this trending topic. Thirty participants responded to this particular consensus question.
You can see an abbreviated version of the answers to our “Low-Hanging Fruit for Machine Learning in Business” question below in our full infographic.
Note: The data for this graphic is no longer available publicly and are only available for Emerj research members. This change was enacted in November 2018.
Survey the machine learning landscape for business on the web, and you’ll see that the three top domains referenced by our pool of experts – advertising and marketing; big data/analytics; and sales applications – are not too surprising. Emerj has had a number of executives and entrepreneurs, from big companies like IBM and eBay to startups like Crowdflower and Humanyze, who have shared their experiences and expertise in these areas on our bi-weekly podcast.
Dr. Patrick Ehlen, chief scientist at Loop AI Labs and a participant in this consensus commented, “With newer unsupervised learning capabilities, most organizations have access to a large amount of data in the form of language or text that can now be plumbed for insights that were not accessible only a couple years ago.”
Customer targeting strategies and service insights, business forecasting and risk modeling, and predictive analytics and data mining have been made possible in large part due to increasing volumes of data available to businesses of all sizes, allowing them to draw intelligent insights on customer behavior and marketing opportunities. The last five years have churned out more machine learning platforms and open-source software, and important lessons – such as the necessity of using the “right” structured data sets (i.e. clean and uniform), though progress continues to be made in applying ML technologies toward analyzing diverse unstructured data (such as health records or email text).
Predictive analytics provides a window into the future by using data collected in the past, allowing companies to take informed actions. Examples of application include:
- Identifying customer flight risks
- Decreasing attrition rate of employees
- Developing a customer lifetime value measure
- Assessing major customer service issues
- Developing targeted audience campaigns
A close fourth ML application that offers significant value for companies across the board is in fraud detection and security solutions. Consensus participant Dr. Alexander D. Wissner-Gross, a scientist, entrepreneur, and Harvard fellow explained, “As classification is now a reasonably well-understood problem, the lowest-hanging fruit for machine learning applications today in medium and large companies tends to involve classifying transactions.” Classification is one type of machine learning algorithm that can help organize transactions and automate accounting duties. Detecting transaction anomalies on credit cards or authentication sessions has particularly ramped up in the domains of finance and consumer retail, with ML systems able to detect cases of fraud in real-time and triggering programmed action sequences that help protect consumer information.
It seems probable that within the next five to 10 years, a majority of businesses in the U.S. (more than half) will be using machine learning algorithms to help guide important marketing and strategic decisions, as well as protect the virtual systems upon which so many companies and their customers are dependent. As technology advances and costs decrease, robotics and drone technology may also come up in the game as an implementable technology for many businesses who would benefit from physical automation capabilities.
Related Emerj Interviews/Articles:
-
Machine Learning Misconceptions – Infographic from our Expert Consensus
-
Network Intrusion Detection Using Machine Learning (Sal Stolfo, Founder, Allure Security)
Fill out this form and receive access to the entire data set from the Machine Learning in Industry Consensus, as well as notifications of future interview series and graphics.
Image credit: Todd Kale