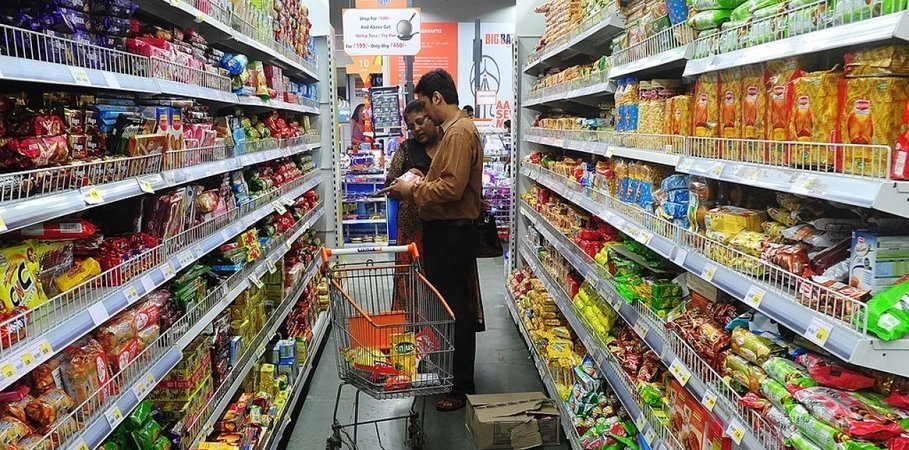
Episode Summary: In this episode of the AI in Industry podcast, we interview Sumit Borar, Senior Director of Data Sciences and Engineering at Myntra, an eCommerce site for fashion, about the current and future state of eCommerce personalization and how the way customers in India purchase products online affects that personalization. Myntra talks about the challenges of bringing dialed-in personalized recommendations to the physical world and the challenges of bringing eCommerce into the developing world.
In addition, he discusses with us the different ways that eCommerce is being experienced in rural parts of India and some of the unique hurdles that they’ve had to overcome. Business leaders looking to apply machine learning and data science to the eCommerce world in developing markets and business leaders aiming to bring data science to the physical retail world should tune into this episode.
Readers interested in the state of AI in India might be interested in our extensive report on the topic.
Guest: Sumit Borar, Senior Director of Data Sciences and Engineering – Myntra
Expertise: machine learning for recommendation engines, spam, search, and large-scale classification
Brief Recognition: Borar holds an MS in computer science from the University of Southern California. Previously, he was a research engineer at eBay and a senior software engineer at Oversee.net.
Subscribe to our AI in Industry Podcast with your favorite podcast service:
Interview Highlights
(02:50) Where does personalization today play its most important roles at your company?
Sumit Borar: When shopping for apparel, apparel doesn’t have a name for a product. All we are looking for is usually either a brand or article type. In an offline retail store, you have maybe 20 t-shirts to look through or select. On an eCommerce site, the limitation in terms of rack space is not there, so we can provide a large amount of inventory for customers to choose from. The downside is that if someone is looking for a specific brand name T-shirt, they could get shown thousands of result which matching their search. The critical part here is that a customer usually loses interest after looking through 2 or 3 T-shirts, saying “I can’t find what I’m looking for,” or “there’s too much information at this point.” This is where personalization comes in.
Let’s say a user is looking for a black T-shirt. What kind of brands does he usually shop? What kind of price points does he usually look at? Does he usually prefer round-neck or collared T-shirts? All of these preferences are usually way clearer [based on] past behavior. That’s what personalization means to us. Can we use those preferences and show him the correct black T-shirts for him?
(05:00) Is this mostly based on their behavior or do we in part get to collect some of this information about who they are: gender, age, geographic location, etc? What’s getting factored in?
SB: We classify these signals into two things: the signals we can get from the user by asking them questions (explicit) and the signals that are implicit: clicks, views, and what they purchased in the past. Typically, explicit signals are where we ask questions or have some kind of interview with the user. That model typically has a large drop-off in terms of how users will respond. They don’t’ want to answer ten different questions. Implicit signals are more valuable because they capture the patterns that people follow in their day-to-day lives. If I typically look at base colors while shopping, and not bright colors, that might not come out when asking an explicit question, but implicit information would tell us that. Also, there are only so many questions one can ask explicitly, but with implicit information, we can drive more for the user. These can be things the user does not consciously understand.
(08:01) Where does implicit and explicit information come in? Do you tend to lean away from explicit information altogether at your company?
SB: While we ask for genders, we have an explicit gender and an implicit gender. Implicit gender involves who someone is shopping for. For example, I might be male, but I might be shopping for my wife. So showing recommendations for just men might not be the right way to go. We start with explicit gender and we slowly move more and more toward implicit gender [based on purchase history].
(12:48) Multiple people can sometimes use the same account to make purchases. Do you have to buyers if that’s the case?
SB: In India, the younger generation is adopting eCommerce quickly. The older generation is still getting onboarded slowly. In those cases, what happens is those in the younger and older generations in the same family share an account. That has lead to us asking, “Who are you buying for?” [as an explicit question]. We assumed most of the time a customer buys for himself, but this was inefficient. When we offered the feature for people to say who they were buying for, we ended up with a million new profiles of customers because buyers were using the feature.
(16:02) When you think about what is going to be possible with personalization two or three years from now that will become a norm, where do you see personalization going as an evolution of the technology? Where will people see this stuff in their everyday lives?
SB: Google is pushing personalization like crazy right now. It started with things that are niche, but now it’s becoming a habit because it knows what you’re going to look for, and it shows the right things. The next stage of the journey is that you will be able to carry your shopping experience from online to offline.
Right now, offline is not really organized in terms of what customers want and how stores can target them or what stores should order. It sits in the minds of the store managers, and it’s not the most efficient because a machine could do a much better job at figuring this all out. All of that stuff will start getting more meshed together to provide a more seamless experience between online and offline.
The other thing is ads. More and more personalized ads will cross the online world to the offline world.
(20:18) What’s an example of how the physical world could integrate personalization?
SB: Think about credit cards and rewards programs. More and more rewards program you see are across multiple stores or multiple domains. In the background though, they would all be the same. What this reward program would be is, essentially, when it scans that someone purchased things in the physical world, it would link that purchase to that person’s unique ID. That information can be collected into the digital world.
Subscribe to our AI in Industry Podcast with your favorite podcast service:
Header Image Credit: Swarajya