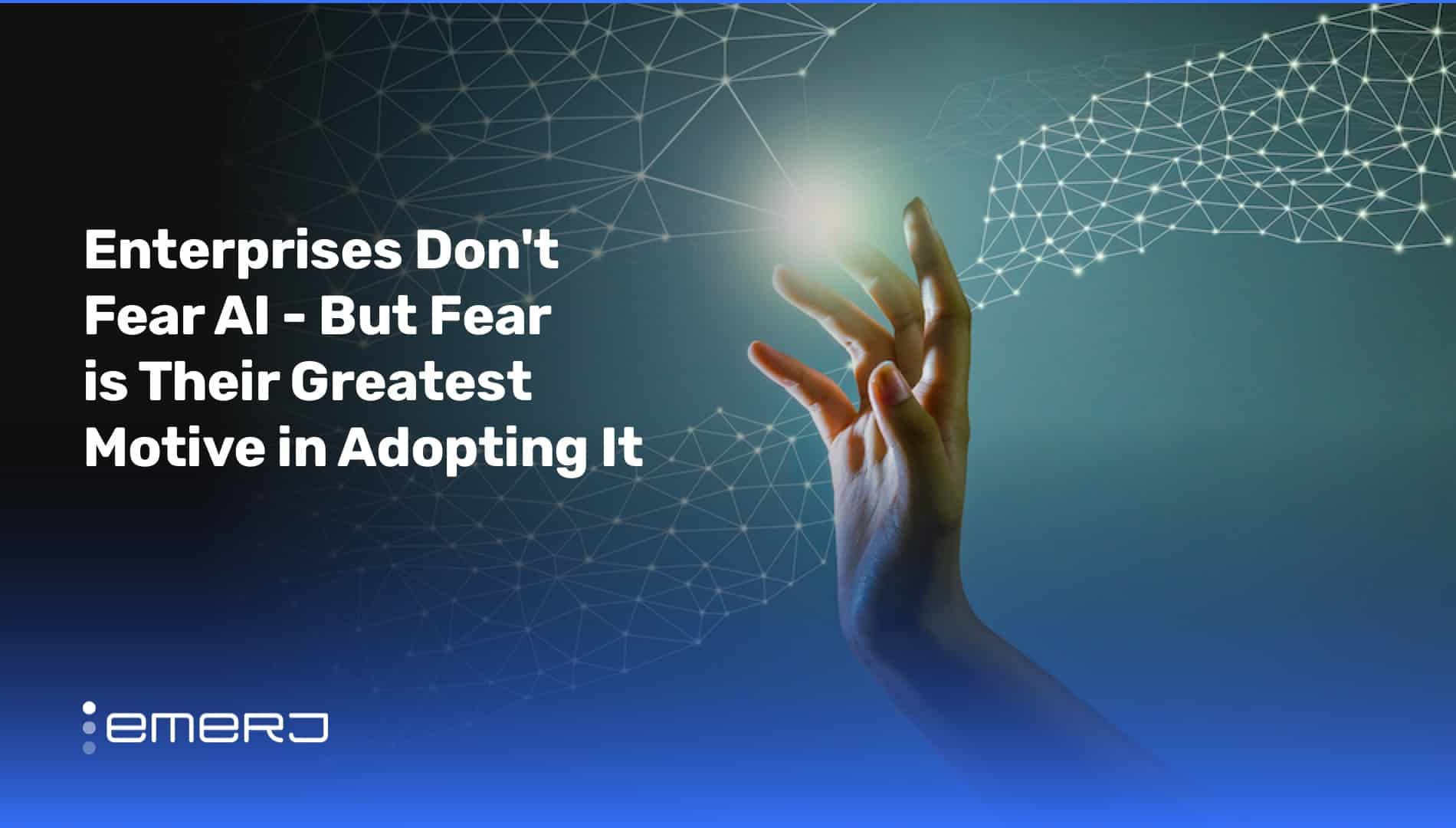
You might not know it when reading AI vendor websites or press releases from enterprises, but when you dig deep enough into why enterprises actual adopt AI, the pattern is clear:
It’s fear.
While it isn’t the only buying motive – it is among the most powerful.
Vendors across industries from financial services to pharmaceuticals will tout many different kinds of benefits on their homepages, but at Emerj, we have noticed that risk reduction is often most important to the actual enterprise buyers we speak to (and to the AI vendor salespeople who are getting deals done).
There are three main reasons that fear rules as an AI adoption motive – some of which are counterintuitive – and we explore them in-depth in this article.
We also explore what enterprise AI buyers and smart artificial intelligence vendors should do about this reality of AI adoption.
But first – why is fear driving AI adoption in the enterprise?
Why Fear Drives AI Adoption in the Enterprise
ROI Numbers Are Difficult to Get, But They Aren’t Even Needed
The first reason is that ROI numbers are both difficult to get and, in some cases, they are not even needed to sell AI into the enterprise. If a vendor is going to sell AI into an enterprise, it has to have some sort of reason why the company should adopt it.
Usually, this reason is for saving money, increasing revenue, or finding new business opportunities. But the fact of the matter is that there is a number of reasons why finding actual ROI numbers is very challenging.
One reason is that artificial intelligence use-cases are very nascent. In other words, most use-cases are very new, and these vendors do not have strong before and after numbers from the companies that they have worked with.
Often, a vendor’s proof of concept project with one client will change into a slightly different application than its proof of concept with another client because clients have different needs. Also, the vendors themselves are trying to figure out how AI can drive value; it is not always self-evident to them.
In addition, a lot of proof of concept projects fail. As a result, even if a vendor did manage to get before and after numbers, they are not always going to be very flattering for them. Many vendors have had a lot of proof of concept projects, but the majority of them were frustrated failures.
This is because AI is very hard to adopt in the enterprise. For more on these challenges and how companies can set themselves up for successful AI adoption well into the future, read our executive guide: Critical Capabilities – The Prerequisites to AI Deployment in Business.
But vendors do not even need to point to ROI numbers to get enterprises to buy. They do not need to say, “You are going to make more money if you work with us,” or, “You are going to lose money if you don’t implement this AI application.” All they have to do is say:
“Isn’t it plausible that if you could reduce the instances of ‘X’ by just a little, then that would help your company?”
This is because it can be difficult for client companies to set up the ability to generate ROI numbers from pilot projects in the first place. These client companies need to track a lot of data they likely are not tracking in order to get these numbers, and oftentimes it is not something they can easily do.
For example, a vendor company might sell enterprise search software that could help call center representatives find contracts and documents related to the customer they are speaking with. It is unlikely that any client company that wants to purchase this software is tracking the time it takes call center representatives to pull up contracts and documents without the software.
It is also going to be very hard to ask the client company to rigorously track the amount of time it takes call center representatives after they’ve started using the software.
As a result, the enterprise search vendor can forego providing ROI numbers to the client before they buy. Instead, it can focus on motivating potential customers to buy by discussing their fears.
For example, the vendor could reference GDPR and discuss how its enterprise search software could help customer service agents find and delete all of the documents a customer asks when they make a “right to be forgotten” request. The vendor could say, “Wouldn’t a regulatory threat be worth any sum of money that you could pay?” And that is often a compelling argument because companies risk being fined 2 to 4% of their annual global revenue.
Sinequa, a prominent enterprise search vendor, has an entire page dedicated to explaining how its software could help with GDPR compliance, as displayed below:
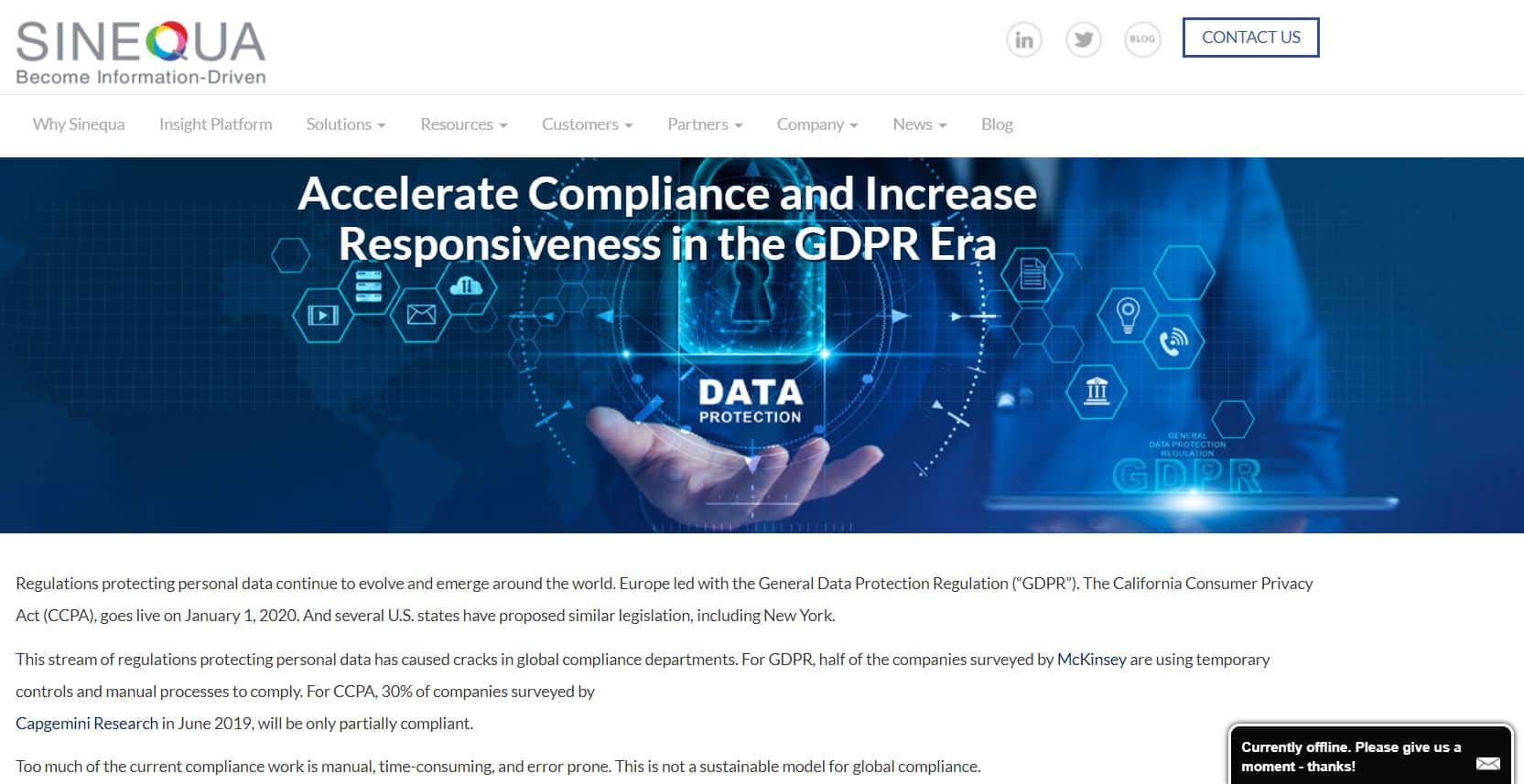
This is not to say that all vendors who appeal to risk don’t have other ROI numbers to lean on. Established firms like Sinequa have a variety of value propositions to appeal to – but risk remains paramount.
Sometimes they do – sometimes they don’t – but for almost all AI vendors, quantifiable “before and after” stories of clients are much harder to put together than plausible stories about reducing risk.
People Want to Avoid Pain
Companies want to avoid losses, but ultimately it’s people who are behind these companies. In large enterprise industries, there are a lot of people simply focused on risk. Companies do not reach enterprise sizes if everybody “move[s] fast and break[s] things,” as Mark Zuckerberg might have felt worked for Facebook.
Many people at large companies are concerned with maintaining the status quo and not necessarily in disrupting themselves. It is not necessarily in the interest of every middle manager or every director or VP to want to disrupt themselves.
The thing everybody has in common is that they do not want to lose, and so people want to avoid pain. As such, the motive for avoiding risk is ubiquitously appealing.
Simple AI Use-Cases in Risk-Related Functions
A lot of risk reduction ties to relatively simple AI use cases. For example, in financial services, anti-money laundering is a very important use-case for artificial intelligence. It is also a very important function within financial services because money laundering can cost a bank a lot of money and result in large fines and punishments. Anomaly detection, a type of machine learning software, is a proven technology in this domain, and so it is easier for vendors to sell products for this use-case.
In order to train an anomaly detection algorithm, a bank might let the software run in the background as it picks up on what a normal transaction looks like. It would then flag anything that deviates from that norm as anti-money laundering or fraud, whichever the algorithm is being trained to detect.
This kind of use-case has evidence of ROI in business dating back to the early 2010s, and so one could call it “low hanging fruit” for machine learning in the enterprise. It is nowhere near as complicated as using AI for other use-cases with much less precedence.
In contrast, imagine a bank is trying to overhaul its wealth management processes with artificial intelligence. The AI software is intended to kick out emails to the bank’s wealth management clients and suggest trades to them. Over the course of several years, the bank is going to be able to determine if these kinds of suggestions to its wealth management clients helps to retain them and improve their customer lifetime value. It is a very complicated process that takes a very long time to be able to split test and determine if it is actually delivering some kind of result.
In the anti-money laundering use-case, the bank uses historical and real-time data to determine if a transaction is money laundering or not, and the software gives a 1% to 99% confidence ratio of whether a given transaction is, in fact, money laundering or not.
This is a much simpler use-case because it involves numeric data that the bank is already collecting in real-time and data the bank has already collected in the past.
It so happens that a lot of risk-related AI applications are relatively simple AI use-cases like this. As such, fear and risk aversion are able to drive adoption because it just so happens that artificial intelligence can much more easily help with reducing risk than in other use-cases.
What Enterprise Buyers Should Do About It
With all of this in mind, enterprise buyers should assess AI solutions through multiple lenses of return on investment. Instead of relying entirely on how much an application feels like it should reduce risk, buyers should think objectively with their procurement teams and with their relevant subject-matter experts in particular business units and departments. They should ask about what their actual needs are and what kind of ROI they should expect from the software they’re looking at. And there is a number of different kinds of ROI that buyers might strive for:
- Efficiency: This involves tangible evidence of being able to save money.
- Revenue: Tangible evidence of being able to drive up a company’s top line.
- Reducing Risk: Taking a potential for risk and gauging some degree of reduction to that risk.
- Business Transformation: Business transformation implies the benefit of disrupting oneself, so to speak—servicing customer needs in a new way and serving the market in a new way that will hopefully help one to win market share. In the longterm, it could improve a company’s prospects for growth and profitability.
Enterprise buyers should look across these ROI types and ask themselves which ROI types they are really looking for when it comes to procuring an AI solution.
If risk reduction is most of what the company cares about, it can adopt AI technology based on that motive alone. But if it turns out that there are other elements of ROI that are very, very important to the business, it should not write them off.
It will be natural to tend toward reducing risk, but if the business determines that increasing its revenue is actually most important, then it may start to look at a different set of applications altogether.
As such, it is important to think ahead of time as to what the business is looking for from an AI solution and then to get a sense of which applications seem most likely to deliver on the kind of ROI that it is looking for.
One of the dangers of relying on risk reduction is that risk is simply what the vendors are using to sell the technology because they have very little evidence of efficiencies and of revenues. Buyers can fall for vendor marketing tactics when these vendors do not otherwise have actual tangible proof of being able to improve business outcomes.
Enterprises that are looking for best-practices in generating ROI from AI projects may want to read our report: Generating AI ROI – Best Practices and Frameworks.
What AI Vendors Should Do About It
AI vendors that are selling these kinds of applications should find and address recognized pains. So, for example, an AI-enabled enterprise search vendor selling into financial services may want to focus on marketing their solution toward finding references to the London Inter-bank Offered Rate (LIBOR) within a company’s contracts. LIBOR will become irrelevant come 2021, leaving many contracts without a reference point for interest rates.
This will require contracts to be reviewed, amended, or rewritten entirely. Otherwise, the bank will open itself up to new potential risks. Banks will need to find a way to search through their contracts for references to LIBOR before 2021, and so this could be a potential pain point vendors could use to market their software. GDPR and other data privacy regulations are of similar concern to financial institutions, and vendors could capitalize on these new risk-related concerns.
For vendors, compliance concerns are a great place to start because big sectors like financial services and life sciences are very fearful of regulatory risk. But all large companies in major sectors have very widely-recognized pain points that they are trying to avoid.
It makes sense for a startup to leverage these in their marketing efforts to marry its value proposition and the AI capability it offers to its specific ability to resolve known pain points. Expert System, an AI vendor offering search and discovery software, does this on their “Legal and Compliance” page displayed below:
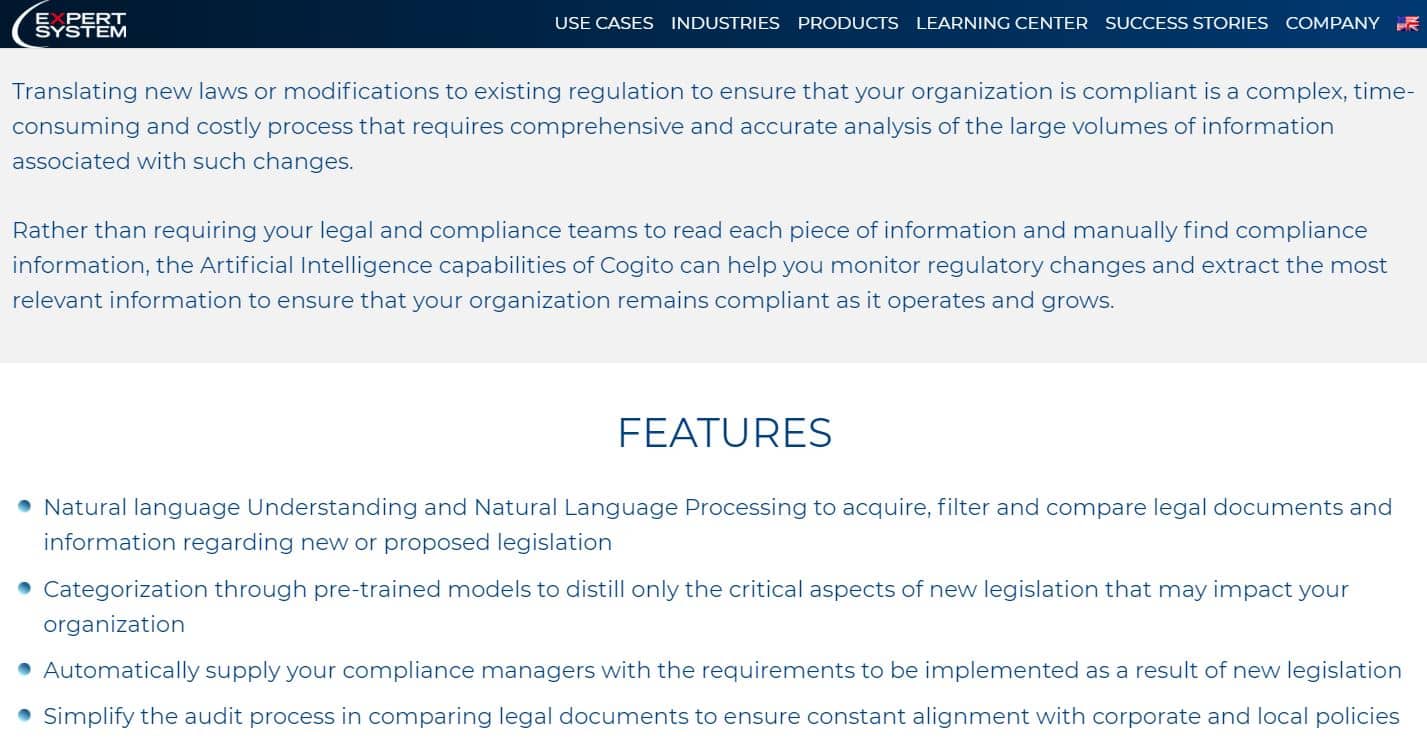
Pain seems to be what is driving dollars, what is helping to close proof of concept projects for AI. AI vendors need to do a better job of understanding the industry they are in and tieing their AI capabilities to a particular pain in that industry and making it clear that the specific capabilities they offer are the best solution to a known pain.
Header Image Credit: Frontend Consulting