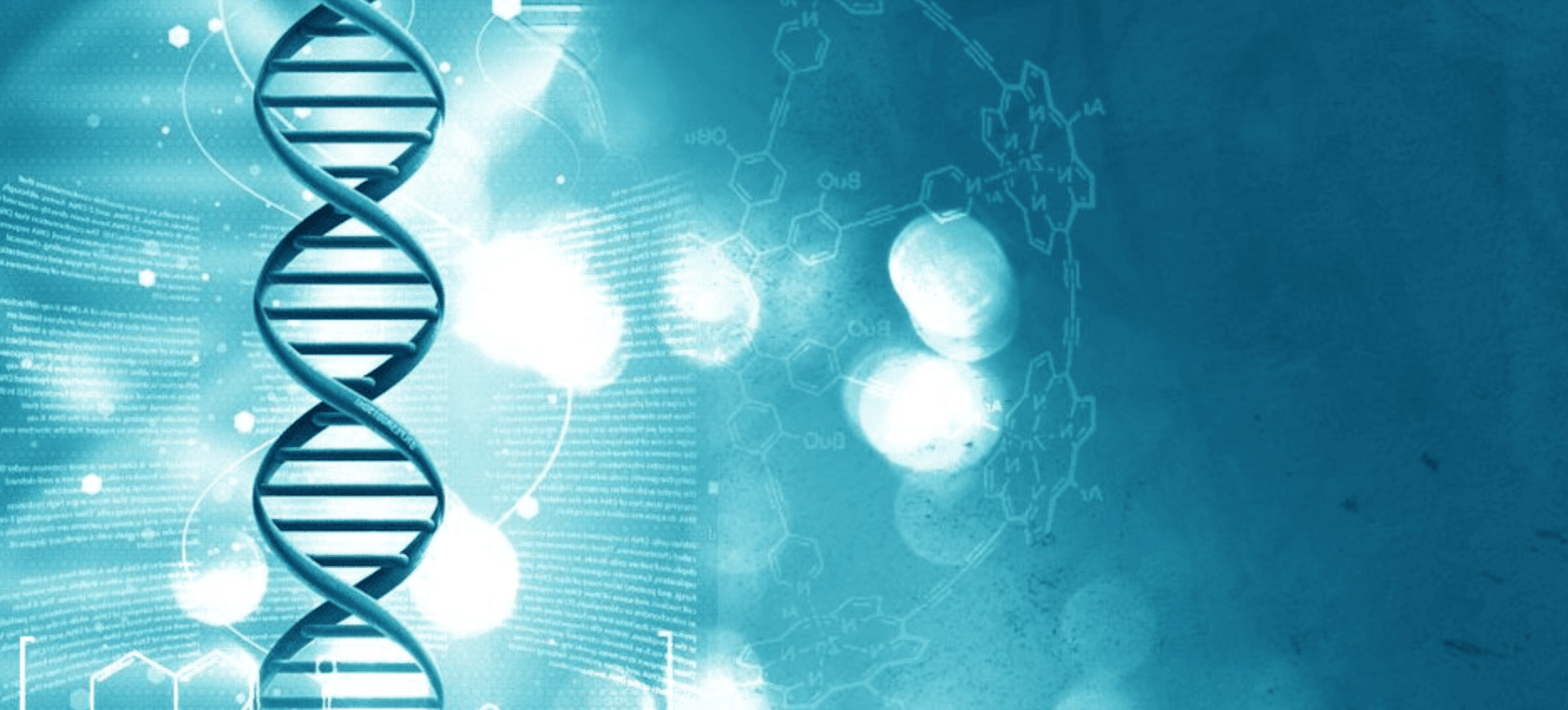
The recent renaissance of machine learning promises to transform the data-rich world of healthcare, and there are hardly any healthcare segments more excited than the pharmaceutical industry.
While hospitals and healthcare facilities have complex sets of stakeholders for AI adoption (e.g. Hospital CEOs may want AI tech, but doctors may resist it – or – A hospital division may be ready to use the latest in AI tech, but patients may not recognize the benefit), it’s believed that the pharma industry may be able to move faster in bringing machine learning immediately to one of healthcare’s biggest data problems: Developing drugs.
Here at Emerj, we’ve seen interest in pharma-related AI articles go up by over ten times in the last year, indicating that machine learning has made it squarely “on the radar” for pharma leaders.
Partnering with NextLevel Life Sciences, we set out to ask life sciences industry experts two questions that matter the life sciences business leaders today:
- What are the main challenges for pharma companies to bring on and integrate AI talent and tech?
- What are successful pharma companies doing today to better prepare for a data-fueled, machine learning future?
The Challenges of Bringing AI Expertise into Life Science Companies
Heading into our interviews, we expected that messy data, talent access, and siloed business units would contribute to AI adoption issues in large pharma companies. But which issues seem most pressing? What should business leaders me most focused on overcoming? That’s exist what we decided to ask our interviewees.
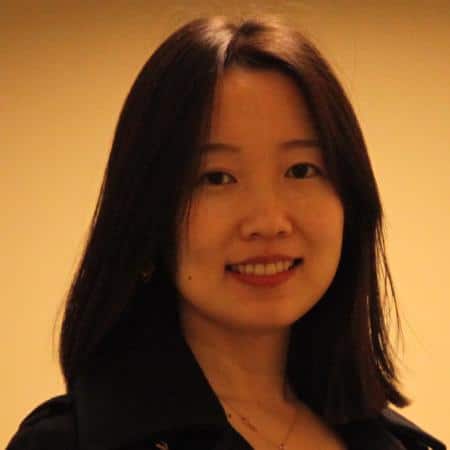
Shelley Zhuang, Founder and Managing Partner at 11.2 Capital:
Interest in AI-driven solutions for life sciences is definitely growing steadily among biopharma leaders, healthcare providers, payers. The biggest challenge is talent. Relatively few people have deep experience with artificial intelligence (probably fewer than 10,000 in the entire world), and even fewer also have biopharma or healthcare experience, and you really need interdisciplinary innovation and collaboration to apply AI to solve meaningful problems in life sciences.
Jan Sagal, Senior Conference Producer, NextLevel Life Sciences
There are a handful of main challenges:
Mindset of adoption: For many years, pharma companies have been watching other industries discovering, designing and implementing advanced technology-driven solutions without taking action themselves. For example,‘big data analytics’ has only been on the radar of some big pharma companies in 2013 (later than may have been seen in many other industries). The life science industry has been a late adopter of most innovative technologies, despite the fact that they do have huge R&D budgets at their disposal.
The nature and availability of data: Each company generates terabytes of data and keeps it hidden behind a firewall. Partly, this is due to strict regulatory and compliance standards and partly due to an extremely competitive environment in which these companies operate. Most of this information sits in silos and for a long time companies did not actually consider this suitable for retrospective analysis. Management often doesn’t see sufficient value in something that requires such a major investment.
Talent and capabilities: Live sciences companies have had to build their new generation of IT teams from scratch and recruit experts from other industries.
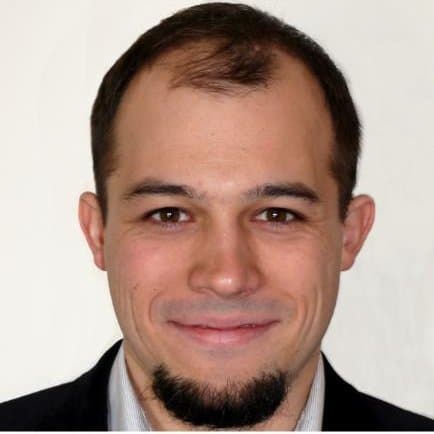
Dr. Kristóf Zsolt Szalay, CTO at Turbine Ltd
There’s a big gap in how biology experts and computational experts think. Even computational biologists usually have very little, if any, wet-lab experience.
AI experts rarely have an expertise in biology, yet working in life sciences requires the understanding of biological processes. Consequently, there is a great competition for the few experts who can bridge both domains.
Here are some of the ways that the approaches of life scientists and computer scientists differ:
- Life science is evidence-based and “only” trusts biological experiments, even though they know (and work around) their limitations (i.e. Time, cost, reliability issues, hard to control or replicate). Their entire reason for being is understanding what causes a certain response, observed in experiments.
- Computer scientists – especially those in the ‘black box’ world of machine learning – often accept that they can only attempt to predict what happens and does not necessarily understand (nor easily prove) why an experiment arrived at a certain result. This machine learning-oriented computer science requires large quantities of high quality data, and tends to celebrate agility, “moving fast”, and the hacker mentality. This contrasts sharply with the mentality often seen in life science, with its emphasis on slow and deliberate movement to ensure the safety and health of patients.
Other challenges that pharma leaders should prepare for:
- Some areas have lots of data (machine vision, molecular space), others – less so (e.g. Drugs in development, biological response to given drugs, etc).
- Also, there are magnitudes of difference between the cost and time taken on the two sides – it’s hard work to make the data needs of AI and the limitations of the laboratory meet. This has yet to be figured out, but there will be large benefits to the companies who move quickly and find a way to do so.
How Leading Firms are Integrating Computer Science and Life Science
The barriers to integrating AI in a life sciences environment are significant, but that hasn’t stopped firms from taking steps in the right direction. We asked our industry experts to share some of their thoughts on (a) what cutting-edge pharma companies are doing well to integrate AI, and (b) what life sciences business leaders should bear in mind moving forward.
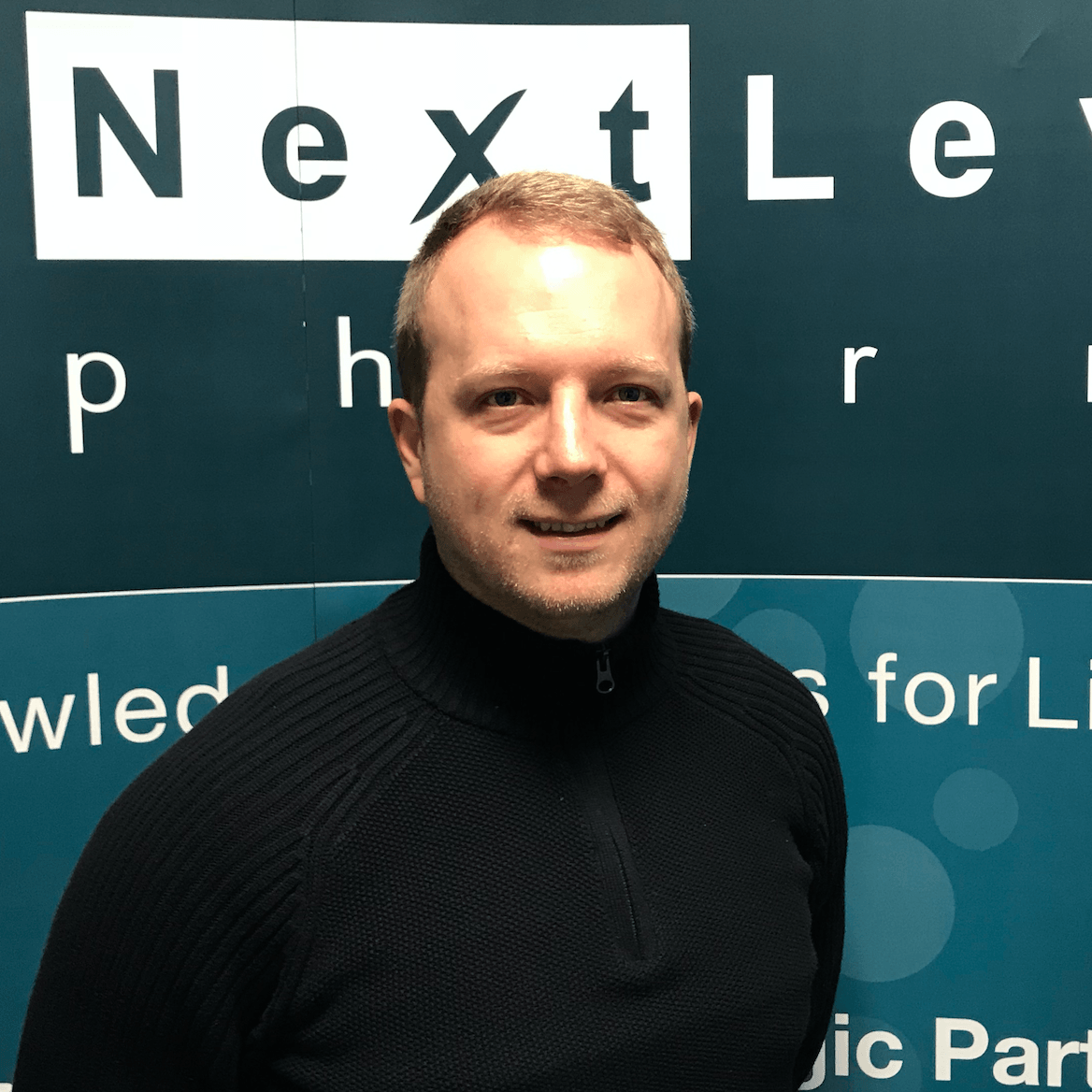
Jan Sagal, Senior Conference Producer, NextLevel Life Sciences:
There are two areas in which pharmaceutical companies are looking for solutions:
Externally, pharma companies are now out there looking for innovative companies that have successfully integrated data-driven and technology-driven approaches in their R&D. Pharma is also very keen to pay for ready-made solutions designed by technology (data, software) companies.
Pharma companies are also more keen to collaborate and partner with their competitors on a pre-competitive platform. Many big pharma companies sit on huge piles of data that is sealed off from the rest of the world and therefore is not used to its maximum potential. By opening up libraries they hope to get more relevant data to feed their models with. Public-private partnerships are also a collaborative model that is used by many pharma companies in EU.
Another emerging model we frequently see forming, is a partnership between pharma companies and technology companies (e.g. Alcon and Google).
Internally, the focus is primarily on building effective IT infrastructures that will enable full scale digital transformations. Companies are designing strategies to capture and store more data, to optimize internal processing and analysis of data and to effectively extract insights needed for strategic decision making. In order to do all this, they need to hire the right people, create guidelines and SOPs of course. Working closely with European universities is one way to approach it.
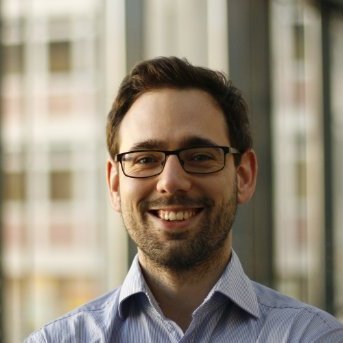
Szabolcs Nagy, CEO and co-founder at Turbine Ltd
I personally know an AI expert who’s been hired with an entire group for the sole purpose of having someone with AI expertise on hand. He’s basically been told “Do what you did before, just tell us the results first from now on”, which is practically impossible.
There’s now a strong movement of pharma trying to open to startups, effectively outsourcing a significant part of their innovation pipeline – a few examples are LEAPS from Bayer, the Quickfire Challenges of J&J and Bayer’s Accelerator Program Grants4Apps.
Here are some ideas that pharma companies are testing which seem promising:
- Building an internal team and an ecosystem of AI players within the company. Paraphrasing one big pharma company we’ve spoken with: “Why couldn’t we try working together first? Why couldn’t we work with as many as we can? Until it brings value, we’re happy to test and collaborate.”
- Removing silos from fragmented data inside the organization and create platforms where structured data is easily accessible to all parts of the organization and for various use cases.
- Make sure AI and computer science teams are cross-functional and divisional, not hindered by traditional barriers between research, development, medical affairs and other market related units. They can learn from all and work with all, they move outside the traditional limitations. This obviously requires smart restructuring, “creative destruction” of some long held barriers and creating new boundaries between units.
There are some other important points for evolving pharma companies to bear in mind:
- Understand that the best insight does not come from one specific data type, there is no silver bullet (e.g. genomics, electronic health records, etc), but a smart combination of various data types and learning from all at the same time (e.g. multi OMICS vs just genomics, gene expression, etc).
- Make a big bet and test these algorithmss in real use cases and on tough challenges, don’t push them to the fringes and “take it slow” – because other pharma players will cut in ahead of you.
- Understand that for AI talent, you’re competing against most the successful tech businesses in the world, so recruiting and retention must be extremely vigilant efforts.
Shelley Zhuang, Founder and Managing Partner at 11.2 Capital:
Given the talent shortage reality, not every life sciences organization will be able to justify the cost or have the time to build an AI practice in-house. One way to bridge this gap is to partner with other companies, for example, there has been a wave of new R&D collaborations between key biopharma players and AI-driven companies, primarily startups, just in the last few years (AstraZeneca with Berg Health, Sanofi and GSK with UK-based Exscientia, Takeda with Numerate, Sanofi with Recursion, Merck/Atomwise and so on).
AI Take-Aways for Pharma Business Leaders
One major lessons that seems to be an emphasis on partnerships and collaborations (with other pharma giants, with technology companies, and with AI startups) in order to quickly “outsource” some of the skills, expertise and infrastructure needed maximize the value of their data. In a world there AI talent is hard to come by, and internal restructuring around computer science is challenging, partnerships may be a way to get initial traction rolling while the underlying barriers within a company are worked on.
Of course, partnerships alone won’t solve the major challenges within pharma organizations, including:
- Isolated data silos, making information hard to access to work with for AI applications
- A lack of AI-specific talent – and particularly – a dire need for AI talent with life sciences experience (a rare find)
Our interviewees identified the breaking up of silos (of both data and functional units) as a critical factor in allowing for machine learning insight, and making room for AI talent within an organization. While there doesn’t seem to be a magic formula for this reorganization (or “creative destruction” as Szabolcs stated), innovative firms are aware that they should be finding a way.
One of our correspondents expressed that most of the efforts to bring AI into life sciences work is relegated specifically to the largest pharmaceutical giants – probably because they have the budget and influence to make the heavy, still somewhat speculative investments into cutting-edge machine learning applications. Given the associated costs and talent scarcity, it seems likely the best partnerships and talent will go to the firms who can afford it.
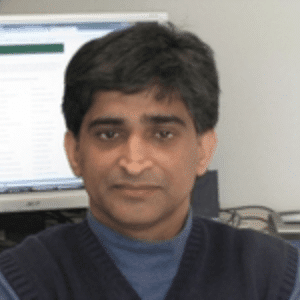
Murali Aravamudan, Founder and CEO at Qrativ:
This [topic of integrating computer science and life science seems most relevant to] to “big pharma”. Having said that, I do see some pharma and biotech companies explicitly forming new “data science” teams independent of their conventional IT efforts. Such “data science teams” tend to have a mix of people from a variety of disciplines (best of breed — bioinformatics, chemoinformatics, clinical analytics, commercial analytics, and of course machine learning/computer science / IT expertise).
Time will tell which pharma companies will pull ahead in the race to maximize their data with machine learning, but it’s at least safe to say that it’s an area of focus for many of the world’s top pharma companies.
This article was written in partnership with NextLevel Life Sciences. For more information about content and promotional partnerships with Emerj, visit the Emerj Partnerships page.
Header image credit: Live Science