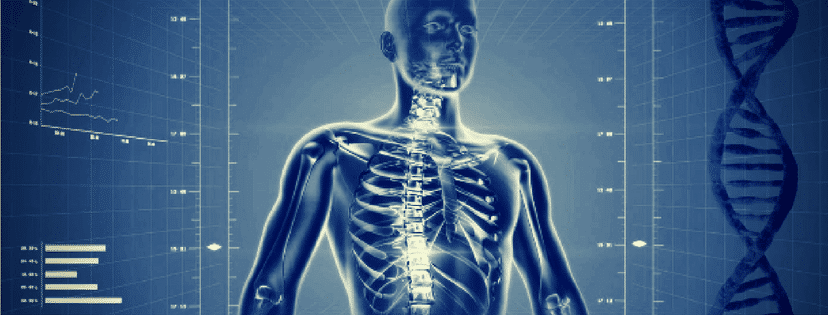
While there have been and continue to be innovative and significant machine learning applications in healthcare, the industry has been slower to come to and embrace the big data movement than other industries. But a snail’s pace hasn’t kept the data from mounting, and the underlying value in the data now available to health care providers and related service providers is a veritable goldmine. In this editorial, we provide an overview of where healthcare’s big data actually comes from, and why providing robust data analytics services in this sector matters.
Doctors’ notes, electronic medical records, prescriptions and similar information are more tangible, but other less concrete sources of information – such as digital data from wearable devices and other trackers – may be poised to help transform healthcare from a prescriptive practice into a more holistic and preventative approach to medicine.
In 2011 alone, the U.S. healthcare system reached 150 exabytes of data. Calculating rate of growth, the referenced paper’s authors predict that U.S. healthcare will soon reach the zettabyte (1021 gigabytes) scale and, thereafter, the yottabyte (1024 gigabytes). To shed some perspective on this level of mass, it (may) be helpful to know that one exabyte of data is equal to one billion gigabytes. Our minds can’t fully grasp anything at that scale, but we can still marvel at this mountain range of information.
Big data in healthcare may be chaotic and distributed, but it’s also much less expensive to own and operate than data stored in relational databases; it’s also more difficult to use. But big data is not size alone; two often overlooked features of big data are its potential to yield valuable insights from complex, noisy (unstructured), longitudinal, and voluminous data, and help guide us toward answers to questions that could not be answered prior.
Therein lies the golden possibility of big data in healthcare – to provide a more holistic picture of individuals and of populations that is not dependent on just one or a few variables, but that makes positive correlations based on a much broader set of variables, and which in turn can help uncover new insights. President Obama’s 2015-announced Precision Medicine Initiative is one vision of a new healthcare infrastructure built on diverse and broad data sources. As Kristen Wilson-Jones, vice president of data and online services for Sutter Health, has stated,
“Big Data will allow traditional claims and procedure data to be integrated with data created outside of healthcare to break down artificial barriers between healthcare settings. For example, data from grocery store purchases, social media, and personal preferences can be integrated to better understand what impacts individual and population health.”
Johnson mentions some unconventional examples of where data may be collected i.e. grocery store purchases and social media. The next section provides an overview of some of the major present (and future) sources of big data in healthcare.
Big Data Sources for Healthcare
1 – The Internet of Things (IoT) – The IoT has already made waves in the energy and utilities, home monitoring, and transportation industries, and the number of connected things in healthcare is growing. IoT platforms are also relatively cheap and a cost-effective option for building and marketing apps at scale. Data sources at present and in the future include (but are not limited to):
- Wearables now allow people to track their heart rate, blood pressure, weight, activity levels, stress levels (Examples: FitBit, PIP, Muse headband, etc.)
- Apps are available on smart phones that track a user’s exercise regimen and intensity, amount and quality of sleep, (Examples: Pebble Time, AliveCor Heart Monitor, MyFitnessPal, etc.). Company Medtronic recently partnered with IBM to crowdsource medical data from apps and other devices.
- Medical devices and sensors that can also send data into the cloud: pulse oximeters, glucose monitors, electronic scales, blood pressure monitors, SpO2 sensors, proximity sensors (like iBeacon), as well as future sensors that will provide data from millions of patients on a continual basis (a suggested read is our interview with Tracy Ingram, founder of BioscanR, a personal health monitor that collects a range of vital signs and that competed for the Qualcomm X-Prize)
2 – Electronic Medical Records/Electronic Health Records (EMR/EHR) – Though they sound identical, the two are different. EMRs contain data from a particular physician’s office, while EHRs are designed to provide a more holistic picture of a patient’s health records over time (birth to death); EHRs can be used as collaborative tools among different medical practitioners and move with a patient from one location to another.
3 – Insurance Providers – Including private payer and plan claims, government health plan claims, and pharmacy claims.
4 – Other Clinical Data – Data from computerized physician order entry (CPOE) and clinical decision support systems (physician’s written notes and prescriptions, medical imaging, laboratory, pharmacy, insurance, and other administrative data).
5 – Opt-in Genome and Research Registries – GenomeConnect, ClinicalTrials.gov, and NIH Clinical Research Studies are just a handful of organizations and institutions that run genomic research studies and collect participant data.
6 – Social Media – Social media posts, including Twitter feeds, blogs, status updates on Facebook and other platforms, and web pages can reflect and provide evidence of a person’s health, mood, state of mind, etc.
7 – Web Knowledge – Less patient-specific information, such as emergency care data, news feeds, and articles in medical journals
Putting Data to Good Use
Companies and organizations who want to put data to good use will need a comprehensive plan for health data collection, optimization and analysis. Applying predictive analytics, modeling and gleaning pattern-based and intelligent insights has the potential to benefit all ‘players’ in the system, including individuals, health care practitioners, public health facilities, life science organizations, health insurance companies, and medical and pharmaceutical manufacturers. Reduced aggregate medical costs seem likely to be one of the major benefits associated with all of the following advantages:
1 – Preventative Healthcare and Patient Empowerment
- Using health data and other variables like socioeconomics can help organizations predict missed appointments, noncompliance with medications, and also predict patient trajectory over time
- The potential to yield optimal outcomes exists across many scenarios, for example: analyzing patient characteristics and the cost and outcomes of care in order to present best-fit and cost-effective treatments, which will also impact provider behavior
- Population-level disease profiling will allow researchers to help identify predictive events and develop more effective prevention initiatives
- Integrating mental healthcare into the traditional clinical setting will help provide more holistic services, and connect patients with the necessary resources and support
- Improved monitoring of patient activities outside the traditional care setting (medication adherence management, home-based monitoring, etc.) will help individuals take increased personal responsibility and help providers determine best treatment plans
- Improved patient care coordination across healthcare providers
2 – Combatting Fraud
- Implementing advanced analytic systems for fraud detection and checking the accuracy and consistency of claims will help minimize fraud, as will nearer to real-time claim authorization
3- Increased Sources of Revenue
- Creating new revenue streams may be available in companies that are able to provide aggregated and synthesized data – patient clinical records and claims data, for example – to third parties who are looking to make advances in the field of medicine and pharmaceuticals (for example, licensing data to assist pharmaceutical companies in identifying patients who have opted-in for participation in clinical trials), which in turn will help drive the creation of new medical devices and pharmaceuticals
Bridging Gaps
As we move into a new era of big data-driven healthcare services, there are two significant challenges that face big data analytics companies and healthcare providers – lack of context and outdated data. Pioneering a big data analytics infrastructure that relies on common data standards and that provides users with access to analytics generated from real-time data sources will be key to creating a flexible and profitable healthcare system. The potential advantages to be gained at a national level seem to outweigh the investments already being made in finding ways to more quickly field and stitch disparate pieces of data into a holistic tapestry of health and well-being at the individual and aggregate population levels.
Sources:
- https://www.healthcatalyst.com/big-data-in-healthcare-made-simple
- http://healthitanalytics.com/features/the-difference-between-big-data-and-smart-data-in-healthcare
- http://library.ahima.org/doc?oid=105683#.WCFNVeErLdQ
- http://hissjournal.biomedcentral.com/articles/10.1186/2047-2501-2-3
- https://www.siam.org/meetings/sdm13/sun.pdf
- http://www.verizon.com/about/our-company/state-of-the-market-internet-of-things
- http://www.imshealth.com/
Image credit: Big Data Made Simple