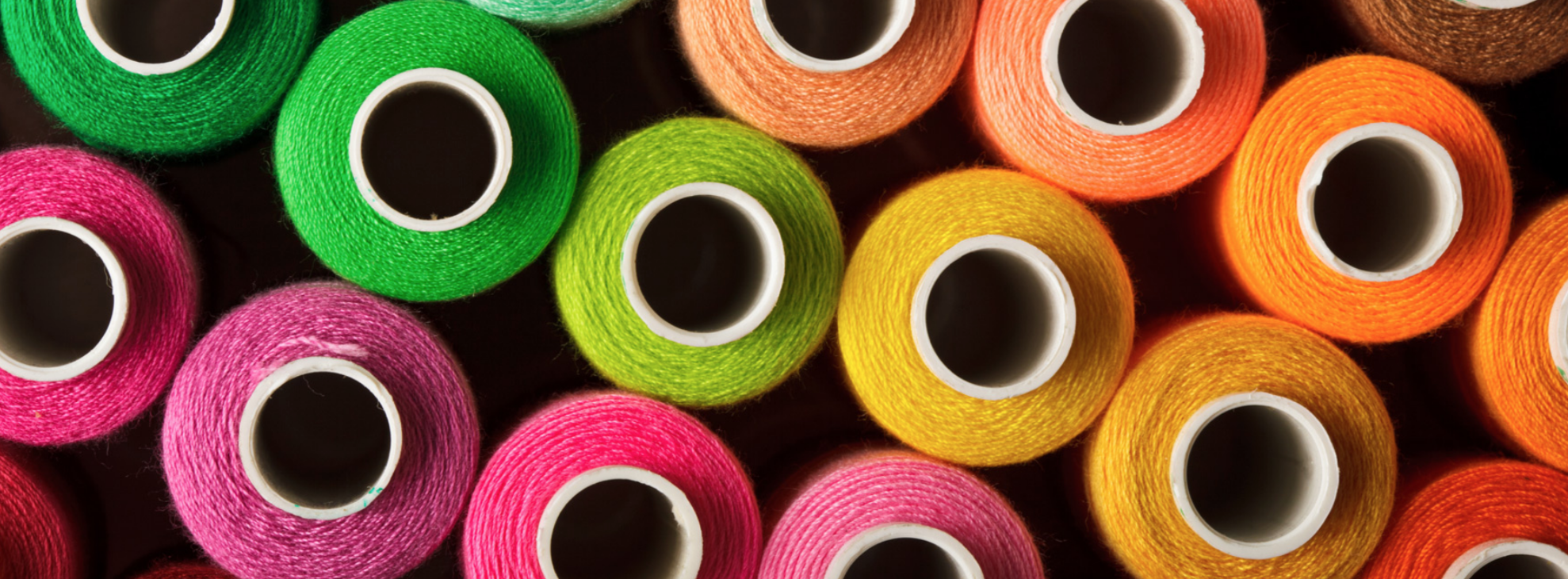
Garment and textile manufacturing have historically been labor-intensive industries, as seen in how many of world’s largest fashion, clothing and apparel brands seem to have a significant portion of their products manufactured in Asian countries such as China, India, Bangladesh, Vietnam, etc.
Much of the migration in textile manufacturing to the East happened over the past couple of decades when labor costs began rising in Asian countries (notably China). With increasing penetration of industrial automation in the industry, textile manufacturing businesses with access to historical and real-time operational data can leverage AI to improve efficiency and augment the capabilities of their human employees.
Readers should know that the adoption of AI applications in the textiles manufacturing industry is still very early, and although there are a few use cases (many of which we’ve explored in the sections below), there doesn’t appear to be widespread adoption of AI – even in developed countries.
Instead, we explore the possibility of applying artificial intelligence in the textiles industry today and what AI might be able to do for industry businesses two to five years in the future.
We’ve broken out the article below into current and future applications, examining companies and use cases individually to explore their business value.
(This article will only be covering textile and fabric applications, and will not discuss fashion. Readers with an interest in fashion can read our full article AI Applications in Fashion.)
Current Applications
Cognex – Fabric Pattern Inspection
Cognex Corp., founded in Boston in 1981 and with over a 1000 employees today is an American manufacturer of machine vision systems, software, and sensors. The company offers its purportedly machine vision-based Cognex ViDi platform tailored for fabric pattern recognition in the textiles industry.
Cognex claims that the Cognex ViDi platform can automatically inspect aspects of fabric patterns such as weaving, knitting, braiding, finishing, and printing. The company also suggests its platform requires no development period for integrating it into a manufacturing system, and it can be trained using predefined images of what a good fabric sample looks like.
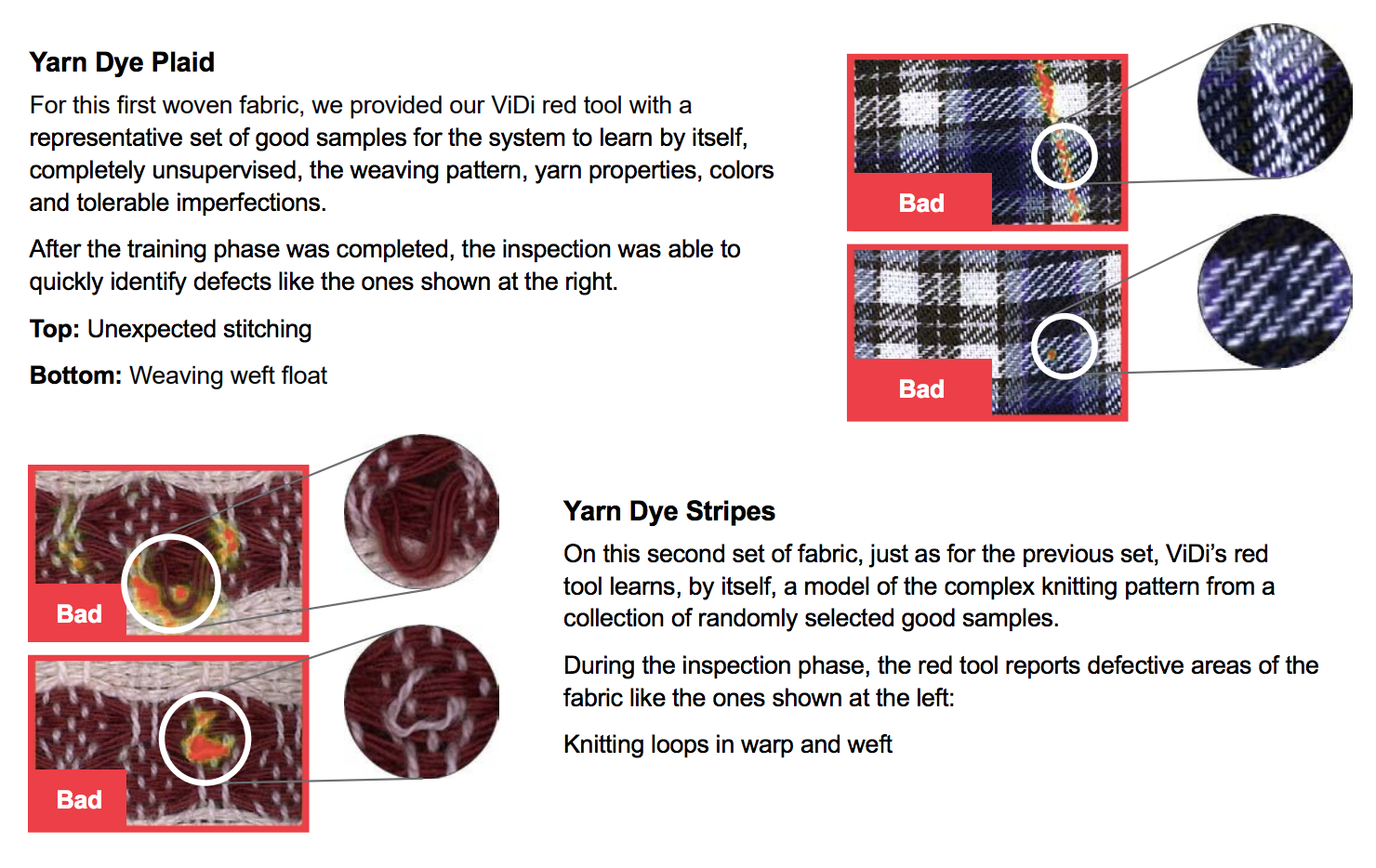
We could not find any list of successful use cases of Cognex’s ViDi technology at the time of writing. It is possible that the technology is in research and development or pilot phase, or that current customers have not given permission to be identified by name.
Based on the description provided by Cognex, the product seems to work as follows:
- Textile manufacturers might save on costs and time taken for inspecting the quality of the final fabric end-product by replacing visual inspection with use the Cognex Vidi platform. Typically the manufacturer might install the camera-based inspection system in their factories and input a few hundred images of “good” final samples, and “bad” samples (see image above).
- The platform learns the weaving pattern, yarn properties, colors and tolerable imperfections from these images and after a training period of a couple of weeks and might potentially be able to detect defects (like wrong knitting patterns) in the textile end-product, saving humans from the manual task of assessing hundreds of yards of material manually.
Below are a few snapshots from Cognex’s brochure illustrating its features, and what kinds of textile defects can potentially be detected by the machine vision system:
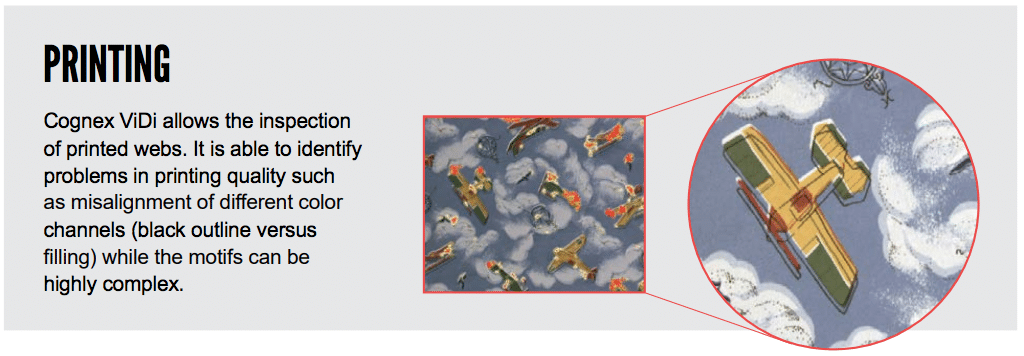
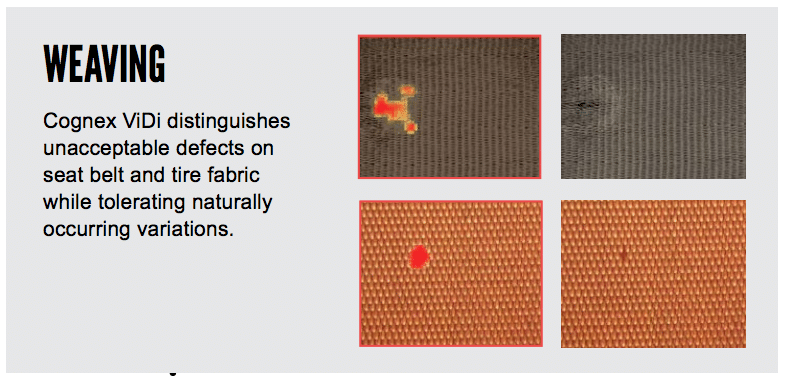
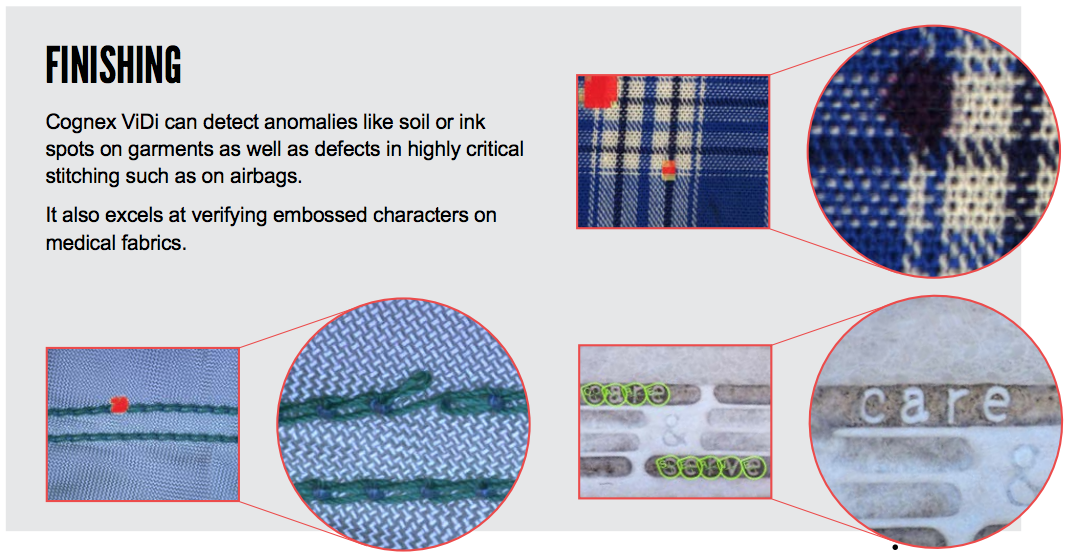
In the short 2-minute video below, a user demonstrates uploading “good” images of fabric samples in order to train the ViDi system to identify fabric errors:
From our preliminary research we found case-studies of Cognex vision systems being used in many industry sectors like automotive and pharmaceutical, yet there seems to be no such resource for the textiles industry.
According to Cognex, several challenges are inherent in inspecting fabric patterns, namely their complexity, variability and the sheer numbers of fabric types. Reto Wyss, Computer Science PhD and the CTO the Director of Software at Cognex was CTO at ViDi for 5 years before the first was acquired by Cognex.
Datacolor – AI Tolerancing for Fabric Color Matching
Datacolor, founded in Lucerne, Switzerland in 1970 with over 380 employees offers color management instruments and software.
To ensure that the original design colors match the colors in a finished textile product businesses usually assign a “color tolerance” – a limit to how big the difference in color between a sample and the requirements of the customer can be, before the sample is considered acceptable. These tolerance values are generally agreed upon internally by the manufacturer or between supplier and customer to determine if the sample passes or fails inspection.
While traditional color tolerancing was done based on numeric descriptions of color through ”instrumental tolerancing systems”, that method generally had a lot of false positives compared with visual inspections, causing delays in the approval process because of the need for careful human intervention.
Datacolor claims it has developed an artificial intelligence Pass/Fail (P/F) feature to help improve the accuracy and efficiency of instrumental tolerance.
Datacolor suggests that its AI feature can take into account historical data of visual inspection results from human operators while creating the tolerances that in turn result in instrumental inspections matching more closely the samples of visual inspections.
Datacolor’s AI P/F procedure purportedly works as follows:
- The textile expert first visually reviews all the individual batches that had been manufactured
- The operators enter the color measurements and tolerances for all the batches in the Datacolor software to help train the AI P/F system
- The AI P/F system can then be tested for new batches to automatically set AI tolerances, training the system to determine which samples pass and fail
The snapshot below shows how textile manufacturers might use the platform to set tolerances for a number of manufactured batches for one customer. The green circle around the center of the graph represent the batches with “ideal” color values, thus passing the test and the yellow circle represent the acceptable tolerance limits:
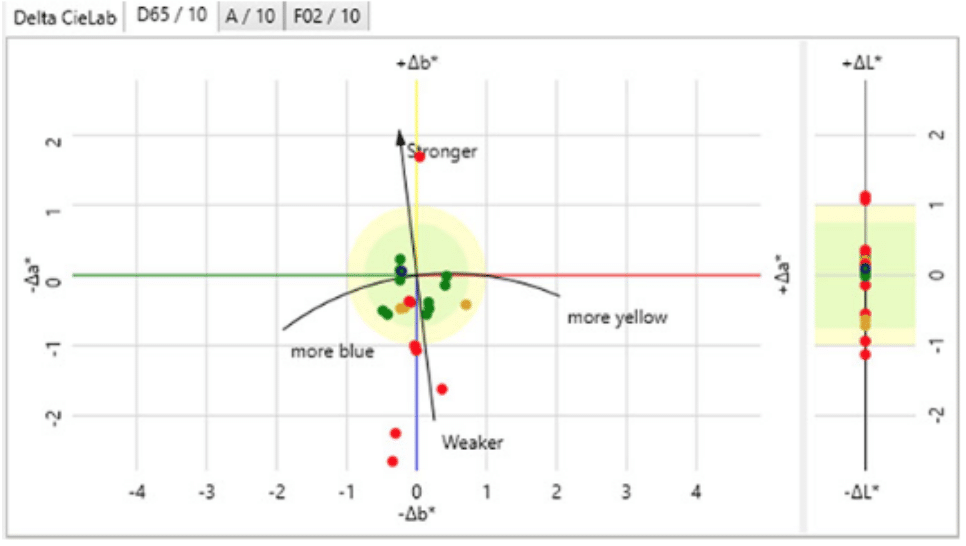
In the real-world, this application might benefit both textile manufacturers and their customers to improve the speed and accuracy of the inspection processes for color matching.
For example:
- A tablecloth business might buy textile raw materials from a fabric manufacturer and place an order for a particular type of fabric in a certain specific color – the color is usually specified in digital terms as a specific number.
- The fabric is the manufactured in several batches with each batch varying slightly in terms of actual color obtained due to variables in the dying process like the amount of color added or the differences in the color bonding (to the fabric) levels in each batch.
- Samples from each batch might be quality checked using Datacolor’s platform to set a tolerance limit. Any samples lying outside the tolerance limit are rejected (see red dots in the figure above)
- The manufacturer’s human inspection officers are first tasked with entering visual tolerance readings for each batch (a few hundreds of samples) into the AI Pass/Fail feature to help identify what samples can be considered good and what samples need to be rejected.
- The AI P/F feature is then fed with images of the fabric from other batches where it can potentially assign the tolerance limits automatically by “learning” from the human inspectors – potentially saving significant time and human effort for the manufacturers by automating tedious color matching tasks.
At the time of this writing, Datacolor doesn’t seem to openly list it’s existing customers, and we were unable to find any case studies of their AI tool in use with any client company.
Datacolor’s software technology is currently led by VP R&D and Chief Technology Officer, Tae Park and Director of Research Michael Brill. Although we must add here that neither seem to have an explicit background in AI, though Michael Brill does hold a PhD in Physics from Syracuse University.
Future Applications
In the last five years, academic research papers have been published on using image-recognition technology in the textile industry in a number of applications, such as grading yarn appearance from the Textile Department, Amirkabir University of Technology, Iran or fabric-defect inspection using sensors. As machine vision continues to make its way into manufacturing and industrial applications, we can expect to see more textile examination use cases in the future.
Yet, commercial use of AI in pre-production textile processing seems limited to only a few applications today, particularly in identifying and grading textile fibers and yarn. Fiber identification and grading in terms of color, length, uniformity ratio, tenacity, etc., may see AI use cases develop in the years ahead.
We suspect that only larger and more tech-savvy textile manufacturers are likely to adopt this technology in the near-term, given the setup, integration, and the potential need for data science talent that would be required to successfully scale such an application across a company.
The coming few years may possibly see the emergence of various vendors offering AI services for applications such as virtual modeling of yarn from fiber properties (Cornell), prediction of yarn tensile properties and yarn unevenness (Fraunhofer Institute).
A few examples of applications that businesses might see becoming commercialized in the future include:
- On the production side, AI might be applied to detecting visual defects in shirts or collars, or it may be applied to automatically detecting and measuring wrinkles on fabric.
- Measuring wrinkles in fabric material is vital as it influences and decides the visual aesthetics of a garment. AATCC (American Association of Textile Chemists and Colourists) methods are commonly used in measuring fabric wrinkle performance but the process is tedious – and trained experts still disagree frequently about the results. Machine vision-based wrinkle measurement might help textile manufacturers cut costs and time required for this process.
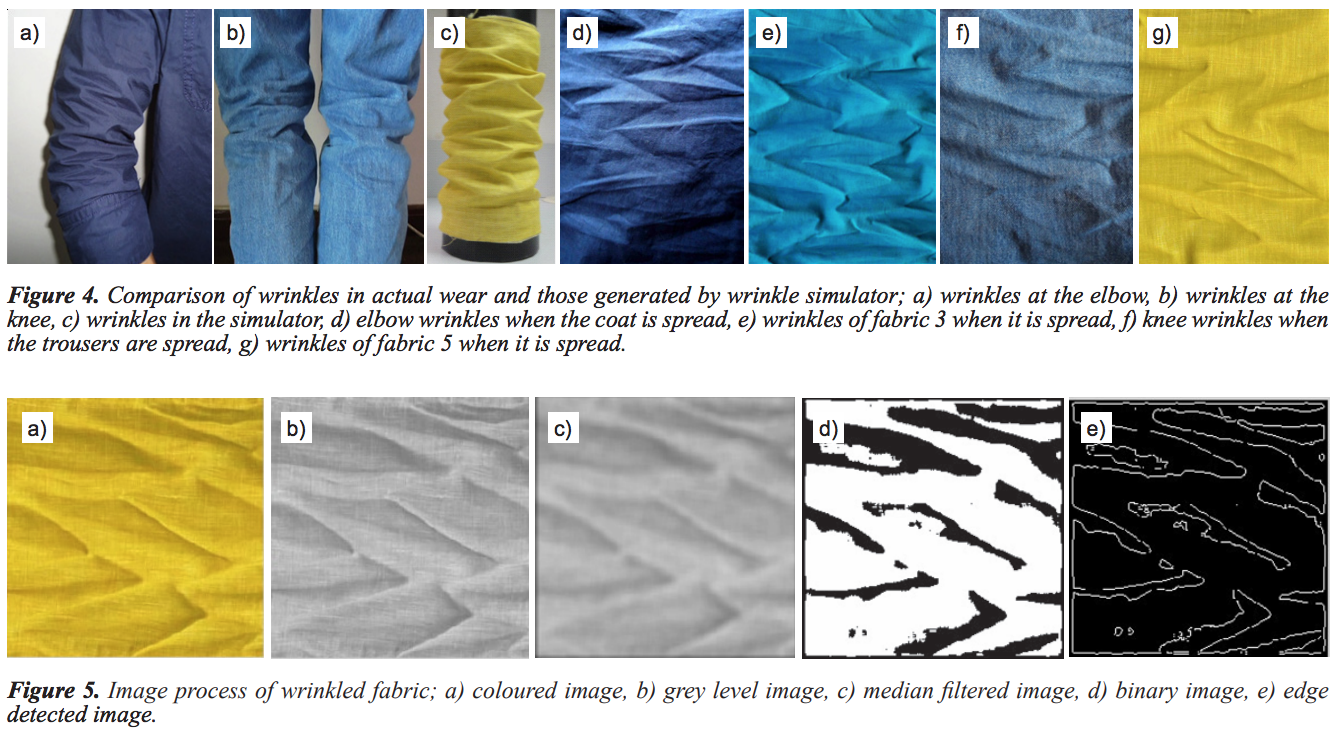
Based on Image Processing, linked above
- Another theme we might see in the future for AI in textiles includes using machine learning to identify previously hidden patterns from raw data. We might also see textile players taking a cue from finance or healthcare industry players and adopt “transferrable: data science and data-mining techniques as explained below:
- In the textile industry, the manufacturing of products (such as t-shirts, tablecloths, etc) generates a large amount of data regarding the raw materials used, machine settings for production, and quality parameters of the product. Machine learning can potentially enable business to find patterns and correlations between fiber properties, process parameters, and yarn properties or among yarn properties, machine settings, and fabric performance. This might help businesses of the future discovers relationships that were previously undiscovered thus aiding in improving efficiency and maintenance. This is very similar to AI applications we see elsewhere in manufacturing, where data about the production process can be collected to improve outcomes.
- Fabrics play an important role in design and prototyping in many industries, such as the design of upholstery in cars or T-shirt designs. Artificial intelligence might help design engineers in the textiles industry ‘3D-model’ yarn fibers in their designs and prototypes.
- Traditional methods of modeling fibers are very tedious and simpler procedural models are too regular and not realistic enough in appearance. Researchers from Cornell have developed an AI algorithm which can model the yarn and fiber properties automatically and realistically without much human intervention.
- In the traditional process, human 3D modeling experts would have to create a yarn from individual ‘virtual fibers’ making it time-consuming and tedious. The research paper from Cornell claims to have developed a method where images of single strands of yarn are scanned using a CT (Computed Tomography) scanner. An AI algorithm is used to convert data from the micro CT scan into a 3D fiber model as shown in the figure below.
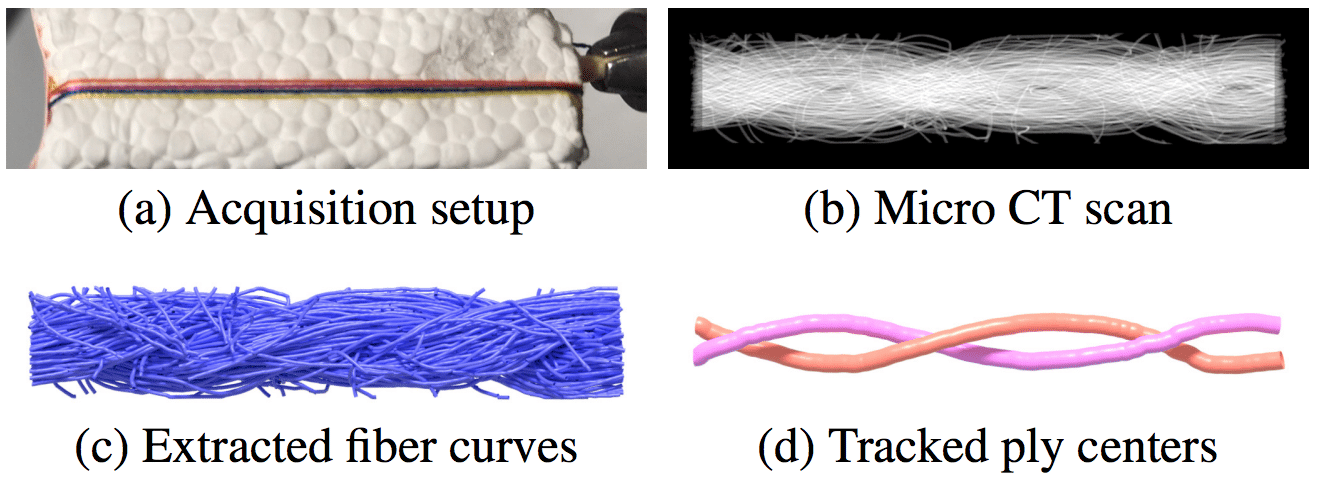
Concluding Thoughts on AI in Textiles
Although a few AI vendors cater to the textile sector today, the number of use-cases and vendors is relatively low compared with other manufacturing sectors, and heavy industry broadly. Most AI applications in textiles today seem to involve the use of machine vision to replace or augment human examination of textile samples – usually in order to detect errors and anomalies.
It is promising to see several research-and-development activities being carried out at universities and other institutions – and we consider this to be a leading indicator in more potential industry use-cases in the years ahead.
It seems clear that real-world AI applications in the textile sector are still at a nascent stage, and it is possible that cutting-edge AI manufacturing applications are more likely to arrive in larger and more modern sectors – including electronics and automotive. One challenge might be the current lack of many system integrators and AI consultants specifically focused on the textile industry (again, possibly due to it’s relatively smaller size when compared to other global manufacturing sectors).
As we look ahead five years, AI might be capable of helping businesses in the textiles industry enhance quality, production, and lower costs. We expect that machine vision for textile inspection will be a low-hanging fruit use case and that a strong ROI from machine vision applications might encourage more enthusiasm and adoption for AI in general.
Businesses looking to leverage AI would do well to note that any such image processing application requires a large trove of existing data for the platform to learn from and that successful integration often involves a significant amount of time, costs and domain expertise from employees working alongside AI engineers – a dynamic that we’ve covered in depth in our AI enterprise adoption article.
Header image credit: iFashion Network