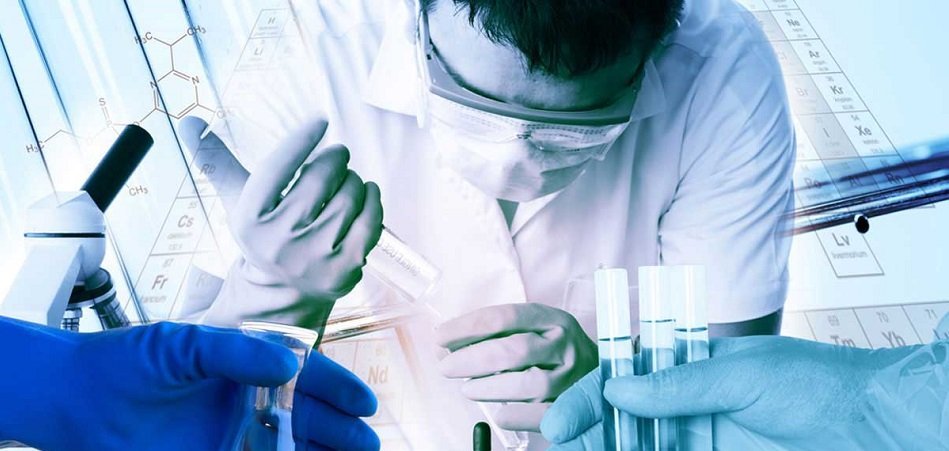
Life sciences companies are likely to begin experimenting further with AI in their workflows in the coming years, but they face challenges in AI adoption due to strict regulations. Machine learning has a “black box” problem, meaning that it’s in many cases impossible to know how a machine learning algorithm comes to its conclusions.
An AI application that detects cancer, for example, may not be able to show an oncologist how it determined the presence of cancer in a patient’s body. As a result, if the oncologist used the application to diagnose a patient, they wouldn’t be able to explain to the patient what makes them sure they have cancer.
This issue relegates AI applications in life sciences to experiments and pilots, and widespread adoption, although likely inevitable, may not come for a while as public opinion shifts toward accepting that its diagnoses are informed by decision-making artificial intelligence and regulations evolve to match. For now, life sciences companies are mostly using AI for research and development, drug discovery, and sales and marketing.
The AI vendors in this report all claim to help research centers and laboratories and pharmaceutical companies with at least one of the following:
- Assisting scientists in handling and measuring liquids with greater accuracy and consistent repeatability, as well as helping them find data about antibody usage.
- Providing sales and marketing teams with information and recommendations on how to engage with healthcare professionals and organizations.
We start our analysis of AI in life sciences with its research and development applications.
Scientific Research
Andrew Alliance
Andrew Alliance offers the Andrew robot, which it claims can help molecular biology, immunology, cell biology, microscopy, and other life science research laboratories assist scientists in handling and measuring liquids with greater accuracy and consistent repeatability in their experiments using machine vision.
Andrew Alliance claims that the robot works with the AndrewLab software, which helps scientists create pipette protocols or predefined written procedures to follow in experiments, through the interface.
The robot may be able to identify liquid chemicals, called consumables, their source location, and their transfer location. The company also claims that Andrew is able to grip a pipette, set the volume, insert and eject the pipette tips, draw from the source containers, and dispense the liquid into new containers without human intervention. The company adds that the software can be programmed to repeatedly dispense the liquids precisely during lab experiments.
The company states that the machine learning model behind the software was trained on light-absorbing liquid samples mixed with Ponceau S, a dye used in electrophoresis studies, that was diluted with analytical grade water. The company also added chemical detergents and other additives to modify the viscosity of liquids.
The samples produced by the pipetting are then measured either by a photometer, an instrument that measures the intensity of light or by a reference balance.
These samples would have been labeled as the concentration of chemicals or compounds. These labeled images would then be run through the software’s machine learning algorithm. This would have trained the algorithm to discern the sequences and patterns of 1’s and 0’s that, to the human eye, form the image of a formula or chemical element as displayed on the application’s interface.
The scientist using the interface could drag and drop the chemical elements and their amounts that are not labeled into AndrewLab, and choose the location where the pipette would source and dispense the liquid.
It is also likely that the robot was trained on images and videos of the pipetting action of dispensing liquid samples at various angles and lighting.
The system then provides documentation of the protocol as a pdf file.
Below is a short 2-minute video demonstrating how AndrewLab works. The software allows scientists to specify pipetting actions such as aliquoting, concentration normalization. The company explains that scientists can Indicate their desired concentration, and the software automatically calculates the required reagent volumes:
Our research yielded no results when we tried to find case studies for the software, nor marquee company clients. The company has raised $14 million in funding from Omega Funds, Tecan Group, Waters Corp., Impeco, Rancilio Cube, and Sam Eletr Trust.
Giorgio Horak is the CTO at Andrew Alliance. He holds an MS in physical engineering, applied physics from Politecnico de Milano. Previously, Horak served as a research engineer at SpinX Technologies.
BenchSci
BenchSci offers a namesake software, which it claims can help biomedical researchers and scientists in research laboratories and centers find data about antibody usage using machine learning.
BenchSci claims that the machine learning model behind the software was trained on 5.1 million antibody products, more than 3 million vendor-supplied figures, and 45,000 figures from third-party validators. These were cross-referenced with over 9 million scientific publications, according to the website. It also claims to have extracted over 2,191,100 antibody usages and offers 3,415,146 images.
The company does not make it clear if the application is web-based or requires some integration with an organization’s system. However, it is clear that scientists can start using the application by entering a query in the application’s search bar. Researchers can search by protein, gene, or clone ID. The application then turns up general hyperlinks leading to information about antibodies that have been proven to work.
We were unable to find information on what the system does with the inputted data to yield an output, but we can infer that all the information has been labeled to enable the machine learning algorithms to search through the database to find data with similar labels and include these in the search results.
According to the website, the application offers 15 types of filters that researchers can use in conducting searches and narrowing down the results to find the correct antibody. One type of filter is figure data usage, which enables users to narrow results based on product specifications from the vendor. Another way to filter is to type the first letters of the antibody or the organism or species on which the experiment was performed. Filtering by vendor allows the researcher the option to either include preferred vendors or exclude vendors they want to avoid.
The company claims that the database is updated with information from new publications and about new products monthly. The application is free to researchers in academic and nonprofit institutions. For business users, the enterprise will need to purchase a license.
BenchSci claims to have content from both open- and subscription-based closed-access journals. Researchers are required to authenticate their subscription through their institution’s portal, such as through their institution’s library website.
Below is a short 2-minute video demonstrating how the BenchSci software works. Users can enter a protein or gene name on the search bar. The user may also search by protein, gene or clone ID. The user can then filter the data based on their experimental needs:
BenchSci claims to have helped Oregon State University (OSU) researchers investigate the molecular and cellular mechanisms of skeletal muscle maintenance, and how skeletal muscle regulates the body’s metabolism.
Myopathy is a muscular disease where the fibers become weak and do not function properly. Genetic therapies are one way to cure myopathy.
Vera Lattier, a researcher and PhD candidate at the university at the time the study was written, who works to discover the genetic markers in mice for myopathy therapy, reported that some challenges in the research included the volume and inconsistent quality of antibodies, the labor-intensive and time-consuming search through numerous publications, and incomplete information cited in papers.
Upon using BenchSci, Lattier reports that it took about 10 minutes to find the antibody that was being used in a current experiment, whereas it previously took a day. Lattier also reported that viewing published figures that were filtered according to the context of the research, was helpful in saving time.
The researcher also explained that using the wrong antibody would have cost losing samples, which are difficult to collect, and could result in a failed experiment. Having found the correct antibody through BenchSci enabled the researcher to save samples from not repeating a failed experiment.
BenchSci also lists the University of Guelph, University of Calgary, and the University of Toronto as some of its clients.
David Qixiang Chen is Co-founder & CTO at BenchSci. He holds a PhD in neuroscience from the University of Toronto. As CTO, he works with high-dimensional data, applying information about the brain into deep learning algorithms for automation and prediction in commercial software. Previously, Chen served as a neurosurgical research assistant at the University Health Network of the Toronto Western Hospital, and a researcher/developer at the same university’s Department of Psychology.
Grail
Grail offers software that can detect cancer early, which it claims could help academic medical centers conduct studies that could detect signs of cancer in the bloodstream, which in turn could increase cancer survival rates, and reduce cancer mortality and suffering using machine learning.
The company states the machine learning model behind the software was trained on clinical and sequencing data of people with cancer such as cell-free nucleic acids that are released into the blood by tumors. The data would then be run through the software’s machine learning algorithm. This would have trained the algorithm to discern which data points correlate to cancer signals.
The software would then be able to predict the presence of cancer cells in the early stages. This may or may not require the user to upload information about the physical symptoms of cancer into the software beforehand.
We could not find a demonstration video available for the software.
Having been established in 2016, Grail is only beginning to organize clinical studies. One of its studies, the Circulating Cell-free Genome Atlas (CCGA) Study aims to discover genomic cancer signals in the blood of people with cancer and compare it with cancer-free study participants. At the time of this writing in December 2018, the website reports that the study aims to enlist at least 15,000 participants in 142 sites in the United States and Canada. About 70% of these had been recently diagnosed with cancer but not yet treated, while the rest are known to be cancer-free.
The study is set to collect data from the participants over a minimum of 5 years. Among the partners in this study are Dana Farber Cancer Institute; the Mayo Clinic in Arizona, Florida, and Minnesota; Memorial Sloan Kettering; and US Oncology Network, among others.
Grail does not list marquee clients but has raised $1.6 billion in funding from Sequoia Capital China, Hillhouse Capital Group, China Merchants Securities, WuXi NextCode, Blue Pool Capital, 6 Dimensions Capital, Huangpu River Capital, ICBC International, and ARCH Venture Partners.
Alex Aravanis is the Chief Scientific Officer and Head of R&D at Grail. He holds a PhD in [electrical engineering and bioengineering from Stanford University and an MD from the same university. Previously, Aravanis served as VP of Engineering at Pria Diagnostics, VP of Development at Sapphire, and Senior Director for R&D at Illumina.
Sales and Marketing of Pharmaceuticals
Aktana
Aktana offers the Decision Support Engine, which it claims can help pharmaceutical companies provide their sales and marketing representatives with relevant information and suggested actions on how to engage with target healthcare professionals (HPC) and healthcare organizations (HCO) using machine learning.
Aktana claims that the machine learning model behind the software was trained on customer relationship management (CRM) data. The company explains that the system integrates with Salesforce, IQVIA CRM, Veeva, and Marketo to help marketing teams create multichannel campaigns to support the work of sales representatives.
It’s unclear how the user interacts with the software or what input is required from the user to activate the machine learning, but the company reports that the system is capable of segmenting customers. From here, we can infer that the algorithms have the capability to create segmented physician groups with similar attributes. This guides the marketing team to personalize the messaging and communication channel.
Examples of segmented physician groups could be those who prefer communicating via email or over phone calls, those who hold clinics in one location, those with the same specializations, and those who might want to consider new treatments for patients.
The system then gives sales representatives at the client company recommendations on how and when to communicate with their targets based on preferred channel, geographic proximity, availability and call history.
We could not find a demonstration video available for the software.
Aktana claims to have helped an unnamed pharmaceutical company’s sales representatives better prepare before offering products to physicians.
According to the case study, Aktana gave the pharma company’s 200 sales reps more relevant information about its customer segments and suggested talking points. Aktana alerted reps when HCPs responded to their communication and helped them identify HCPs who could with important competitive trends.
As a result of using the application, the case study reports that more sales reps were satisfied with the CRM and BI tools increased, from 58% to 81%. The reps also found it easier to prioritize the HCPs to visit from 52% to 80%. The case study also reports that 60% of sales reps’ visits to target HCPs were reduced by 3-5 days.
Aktana lists Sunovion Pharmaceuticals, Pfizer, and MSD as some of their past clients. The company has raised $49.2 million in funding from Leerink Transformation Partners, Safeguard Scientifics, and Starfish Ventures.
Marc Cohen is the Chief Science Officer at Aktana. He holds a PhD in Operations Research from the University of North Carolina at Chapel Hill. Previously, Cohen served as Chief Science Officer at Archimedes, VP of Research at Fair Isaac Corp., and Senior Director at SAS Institute.
Header Image Credit: VI SEEM