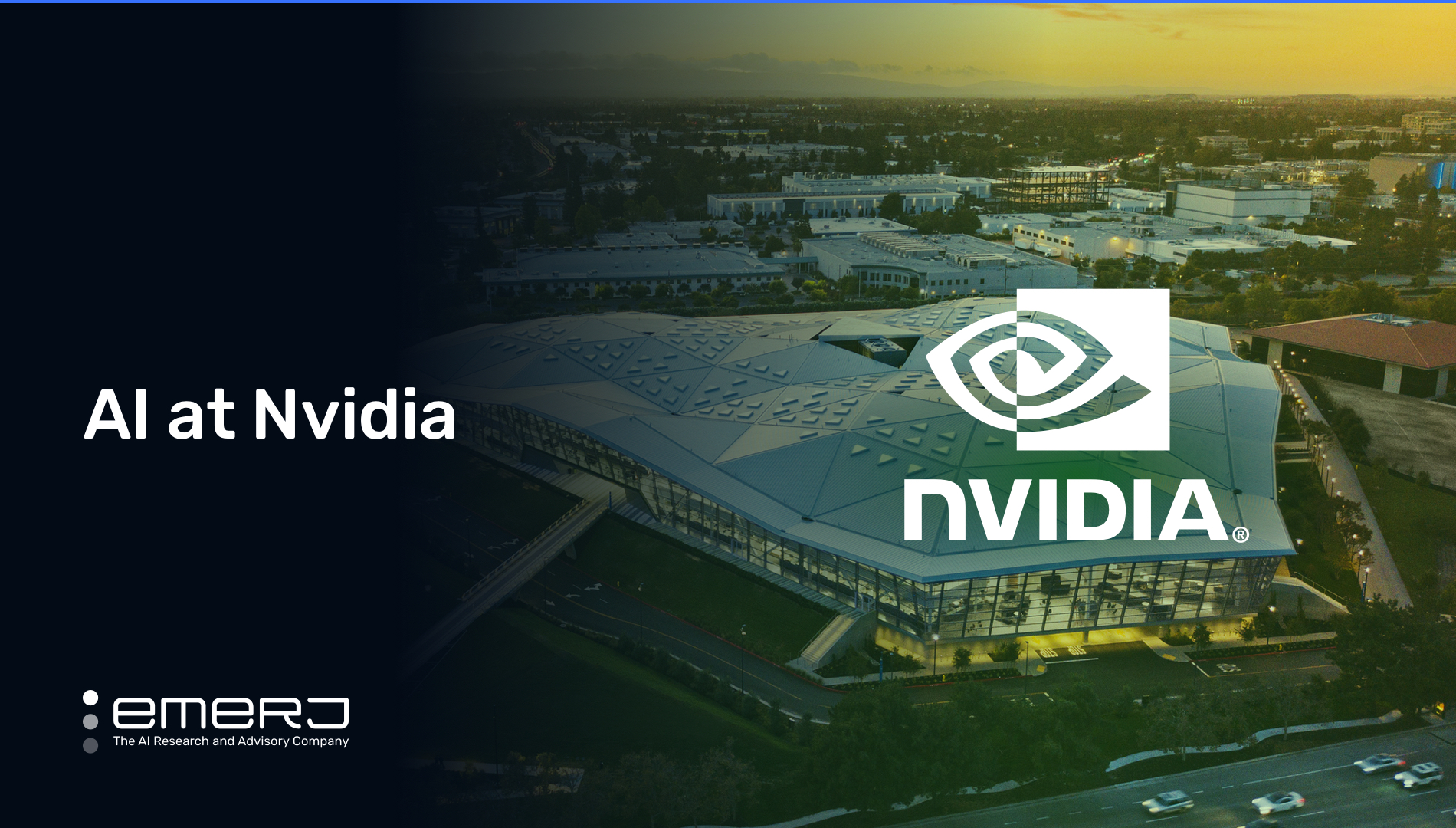
NVIDIA is a multinational company known for its computing hardware, especially its graphics processing units (GPUs) and systems on chip units (SoCs) for mobile devices. The company went public on January 22, 1999.
While the company remains focused on hardware production, it has implemented deep learning and AI into its GPUs and specific software, such as its autonomous driving platform.
The company trades on the NASDAQ (symbol: NVDA) with a market cap of just above $418 billion and employs approximately 23,000 globally. In its annual report, the company reports roughly $27 billion in revenue for its fiscal year ending 2021.
In this article, we’ll examine how NVIDIA has implemented AI applications for its business and industry through two discrete use cases:
- Enhanced Video Resolution – Nvidia uses machine learning to enhance video resolution and audio quality during real-time video calls.
- Analyzing the Impact of Marketing Campaigns on Sales – NVIDIA uses ML algorithms and data analysis to analyze marketing touchpoints and optimize the marketing campaigns for a new line of GPUs.
Use Case #1 – Using Machine Learning to Enhance Video Quality
NVIDIA claims its research scientists were experiencing a problem common to just about anyone working from home: audio and video breakups and freezing on video calls. The company claims that these videoconferencing problems were disrupting critical work processes.
Streaming video calls require a lot of bandwidth, often resulting in less-than-ideal communications: frozen video and/or audio, poor image resolution, audio breakups, and so on.
On the video side, one thing that increases bandwidth requirements is a software called a video codec, which most organizations use to compress and decompress video. On the audio side, extraneous background noise can be disruptive. Let’s examine what NVIDIA did to address both.
NVIDIA claims that traditional video transmission compression software like video codecs transmit a vast number of pixels, which requires a great deal of data and bandwidth to send and receive.
Instead of using this traditional software, NVIDIA claims it uses a specialized ML model in its Maxine product called a generative adversarial network, or GAN. The company claims that this reduces bandwidth by ten times over conventional video transmission methods.
The company claims its software works by first reducing the number of pixels transmitted. The model accomplishes this by extracting user image data limited to key facial points, specifically the user’s eyes, nose, and mouth, instead of the entire image.
The ML model then uses the critical facial area data and produces an output in the form of a reconstruction of the initial image and subsequent images. NVIDIA claims that much less data is sent over the network using this ML model, which results in better image quality.
In the following video of approximately 2.5 minutes, the company explains how its model works:
Besides improving image resolution, the company claims its ML model also reduces low-light camera noise and applies “AI-powered background removal, replacement, or blur. The company also claims that it works regardless if the user is wearing a mask or hat.
On the audio side, NVIDIA states that it uses AI to both filter out unwanted background noises (i.e., noise cancellation) and enhance audio quality – specifically speech. However, the company does not reveal how it does this aside from stating that the AI uses what appears to be neural networks to improve the quality of the user’s speech.
Regarding tangible business results, our research was unable to uncover any.
Use Case #2 – Predicting Customer Churn
NVIDIA wanted to determine how its marketing strategy impacted the sales of a new GPU line. Adobe determined that measuring NVIDIA’s marketing effectiveness was the best way to achieve its client’s goals. With this data, NVIDIA could potentially see the financial contribution of each marketing channel and, if desired, tweak its marketing campaign to achieve desired business outcomes.
It seems that Adobe’s method of quantifying NVIDIA’s marketing campaigns involved the analysis of multiple datasets to identify and analyze the most influential channels for acquiring customers, increasing conversions, and boosting incremental sales. Adobe states that it uses software called Attribution AI for this purpose.
Adobe first acquired the relevant market and sales datasets. The marketing and sales data was fed into Adobe’s Attribution AI platform, which used an AI algorithm based on a statistical technique called cooperative game theory. Adobe claims that it uses this algorithm because marketing touch points “are often misrepresented and undervalued or overvalued” with traditional rules-based models.
Below, an approximately 3-minute video explains how the software works:
The output of the algorithm is claimed to show the influence and incremental sales for each marketing for each channel via chart.
In NVIDIA’s case, the GPU marketing campaign’s channels (paid media versus email) and campaigns (bundled offers vs. brand refresh) were the most influential in driving product sales. It appears that the software also gives NVIDIA the ability to analyze the incremental sales of each marketing channel individually and in the aggregate.
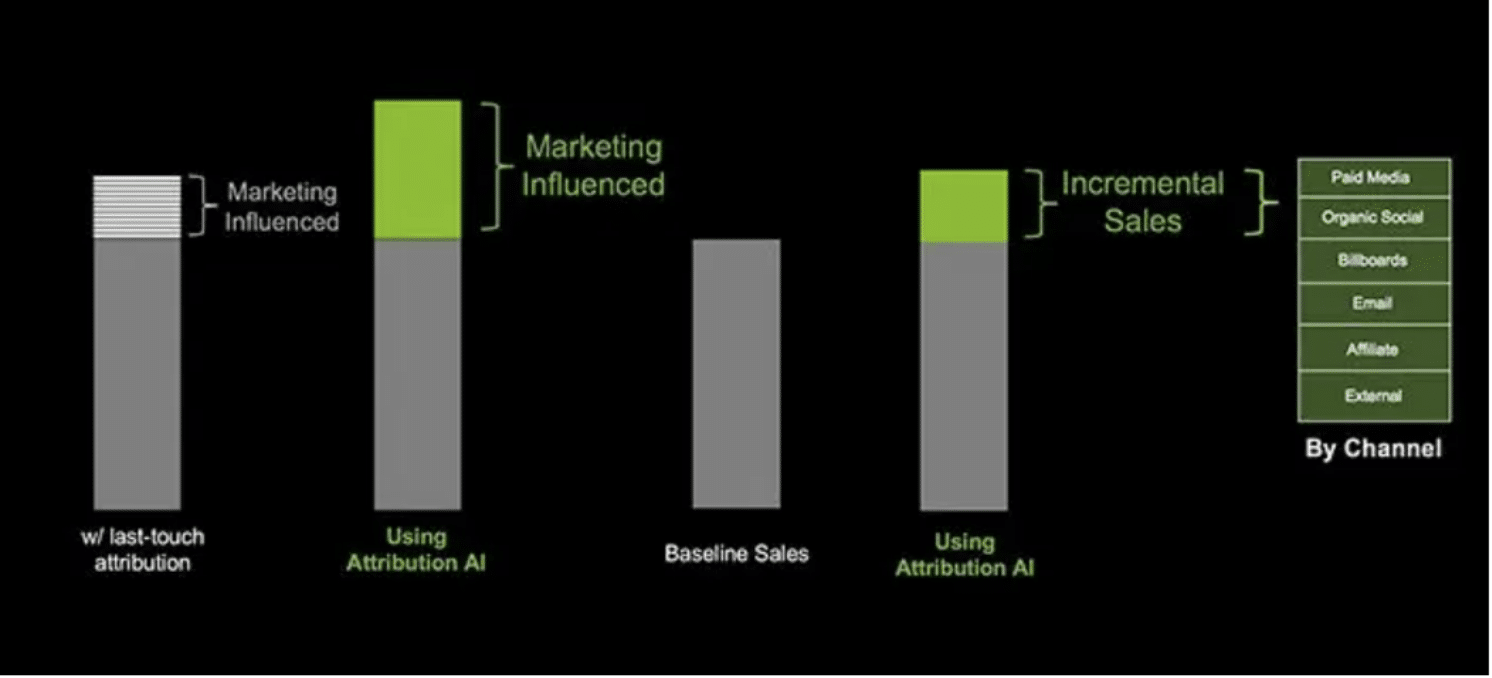
It appears that NVIDIA could select individual channels such as paid media, organic social, billboards, email, affiliate, and external and see their respective influence on both marketing and incremental sales.
Regarding tangible business results, Adobe claims that its solution gave NVIDIA the ability to quantify incremental sales and marketing effectiveness across channels. More generally, Adobe claims that its software-enabled NVIDIA to gain a more accurate picture of its marketing effectiveness by isolating last-touch attribution, which can skew the analysis.
Notably, no quantitative evidence is given to support Adobe’s claims of successful business outcomes for NVIDIA.