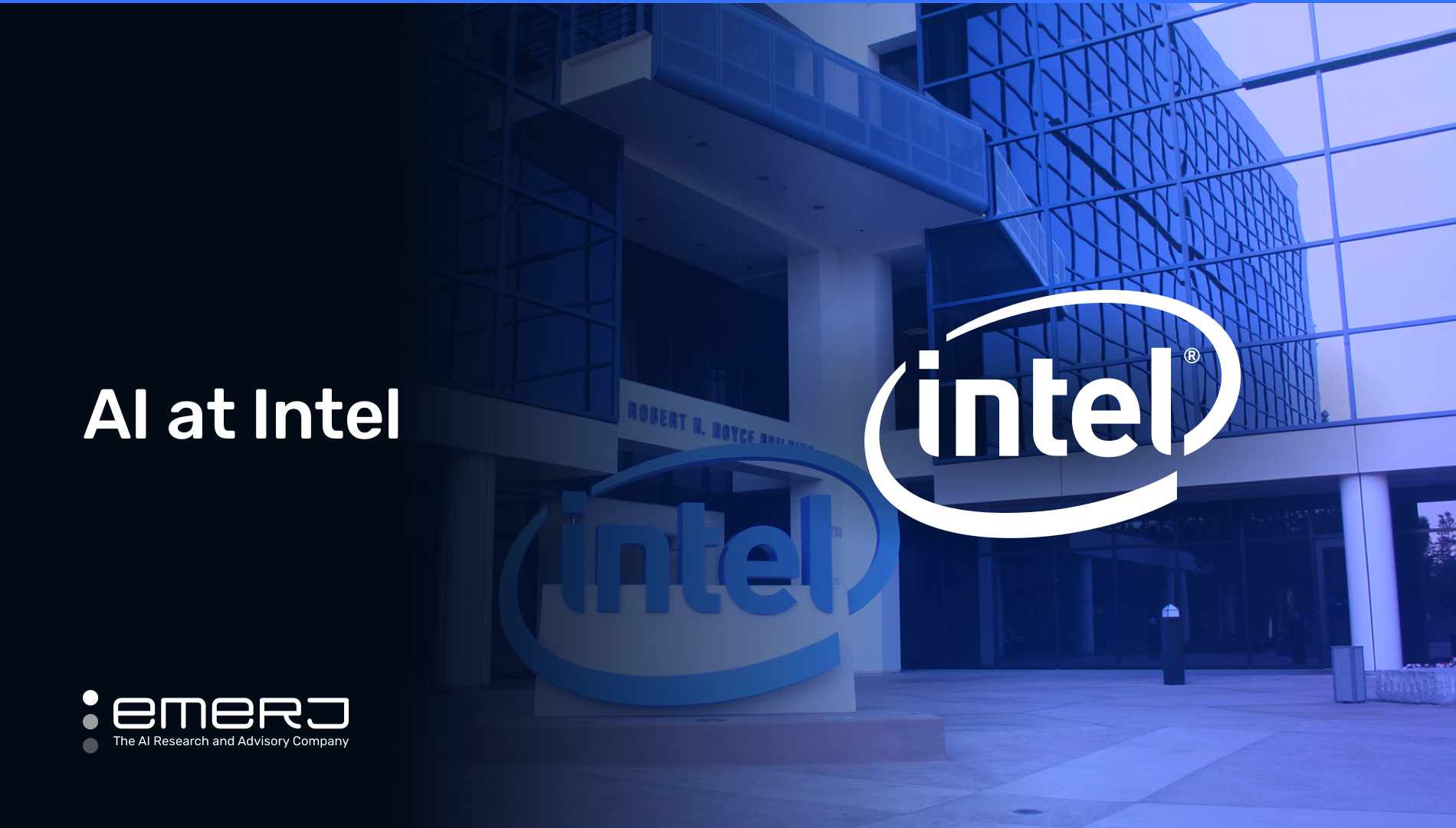
Intel was founded in 1968 by Robert Noyce and Gordon Moore, who had previously been among the founders of Fairchild Semiconductors. Today, Intel employs over 121,000 people worldwide. In its 2021 annual report, the company reported revenues of $79 billion. As of 2022, Intel trades on the Nasdaq (Symbol: INTC) with a market cap that exceeds $178 billion.
Intel has acquired multiple companies across various AI disciplines. Some of the AI-focused companies that Intel has acquired in recent years include DeepMind in 2014 for $500 million, Habana Labs (2019, $2 billion), and Granulate (2022, $650 million.) Regarding investments, the company announced in 2020 that it was committing $132 million to 11 AI startups. Intel’s CEO, Pat Gelsinger, lists AI among what he calls the “4 superpowers” of the “digital renaissance,” emphasizing the criticality of actionable insights.
This article will examine how Intel has applied AI technology to its business and industry through three applications:
- Building Deep Learning Applications – Intel developed oneDNN to help companies optimize computer hardware such as CPUs and streamline the development of deep learning applications.
- Identifying Sales and Marketing Opportunities – Intel integrates AI segmentation with an NLP model to help the company identify and understand new markets and acquire clients.
- AI for Inventory Optimization – Intel uses basic AI algorithms to optimize its inventory of spare parts at its factories.
Building Deep Learning Applications
Building deep learning applications require a vast quantity of training data, a layer of the algorithm, and significant computing power. To help businesses overcome these difficulties, it offers a cross-platform software called Intel oneDNN.
Intel claims that it is the function of this product to help neural networks run as fast as possible. To achieve this, the company states that the software uses highly optimized versions of user input data. The company states that oneDNN is used for two purposes: to build deep learning models or improve its performance.
According to Intel, the business value of the software is tied to the streamlining of workloads across diverse system architecture that oneDNN afforded to programmers and, by extension, their employers. As an engineer from Intel explains:
“[For example], I want to be able to run object recognition on a 1 watt drone or process 1000 video channels in the cloud. But each … have their own libraries, tools, or unique programming model. This makes it challenging to be productive. The bold vision of oneAPI is to have cross-architecture, cross-vendors portability.”
In the video below, an engineer from Intel provides an overview of the oneAPI product:
(Source: Intel)
Intel uses oneDNN as the foundation for many other products. For example, the company uses oneDNN to provide training data for its analytics toolkit product, and its open-source OpenVINO toolkit uses the product for inference. As such, oneDNN is often used in conjunction with other software to power or optimize its software or hardware.
The company claims that oneDNN is the “library of choice for neural network” operations such as training data for inference for many leading platforms and supercomputers such as TensorFlow and Fugaku, respectively.
Concerning tangible business outcomes, Intel claims that one major tire manufacturer was able to use oneDNN in conjunction with OpenVINO to increase the detection accuracy of more than 20,000 times per day to 99.9 percent – up from 90 to 95 percent. Intel claims that it was also able to reduce the client’s labor costs by $42,000 per production line. In another case, Intel claims that it was able to reduce a client’s compute latency by 95% by implementing oneDNN into a multi-layered solution.
Identifying Sales and Marketing Opportunities
Similar to other enterprises, Intel must also identify sales and marketing opportunities to expand its business. The company claims that this is a “key challenge” in which “there is a need for intelligent automation.”
Prior to the implementation of a new sales and marketing system in 2020, Intel states that its salespeople had to rely on two methods to find business customers: manual search (e.g., Google, Bing) and vendor management tools (e.g., SAP Fieldglass, Genuity, etc.).
According to the company, such methods did not allow Intel’s salespeople to efficiently segment and identify relevant business customers. The main reason for this problem is the Intel salespeople’s requirement that their sales management system include nuanced, “Intel-only” language and concepts – a need that the aforementioned methods could not meet.
As a result of this, the company claims that sales staff was lacking the ability to (a) identify the most relevant and potentially profitable clients, and (b) tailor their sales approach to business customers, potentially losing out on opportunities.
To help solve this business problem, Intel and their analytics team and developed a system which they claim both “mines millions of public business web pages,” and “extracts actionable segmentation [data] for both current and potential customers.” Both are “two key customer aspects that are essential for finding relevant opportunities,” according to the company.
The first part of the process – mining the data – uses a web crawler with certain technologies to “ensure robustness.” The company claims that this capability includes the streaming platform Kafka, the ML tool TensorFlow, and the graph database platform Neo4j. In simple terms, Kafka enabled the voluminous data streaming requirements; TensorFlow provided the “muscle” to power the ML model, and Neo4j transformed the raw data into graphical representations.
The mined data includes information about a company’s brands, products, verticals, and other variables. Based on this data, the company claims that customers are then segmented according to industry segment (“ranging from broad” to specific verticals) and functional role (e.g., “manufacturer,” “retailer,” etc.) Both data types are “two key customer aspects that are essential for finding relevant opportunities,” according to the company.
The second part of the process involves the feeding of web page data into an NLP and neural network with a pre-trained BERT language model. The data is enriched by web crawling the company’s Wikipedia page.
The second process, the company claims, produces the robust customer classification using the nuanced “Intel-only” language and concepts required by its salespeople to discover new sales leads. The model apparently produced more specific and actionable customer data using this niche language compared with the more traditional, manual process.
Concerning tangible, quantifiable business results, our research was unable to uncover any. Intel claims they “were able to discover new leads in specific industries much faster and more accurately than using traditional methods.”
AI for Inventory Optimization
As a third bonus example, we are going to discuss how Intel optimizes inventory using AI with Gopalan Oppiliappan, head of the company’s AI Center of Excellence.
In this short podcast interview, Gopalan also shares a specific use case involving the successful implementation of AI to help the company with its inventory management problem
The business problem that Intel faced was a “classical supply chain problem,” says Gopalan. Some factories had an excess of parts while others faced a shortage. This had a bottom-line impact, of course. The company had to write off inventories with excess spare parts, as the items were not used in the acceptable timeframe.
The challenge was to see if the company could somehow use AI to help. The company asked itself a couple of questions: Can we help our factory managers predict when these spare parts will become obsolete? If so, what can be done to prevent the parts from becoming obsolete? Is there a better, more profitable business activity, such as selling the parts back to a vendor at salvage value?
Gopalan states that the company first needed to understand the historical consumption pattern of the parts. To accomplish this, Gopalan states that his team mapped the consumption patterns using traditional statistical methods. The statistical model enabled them to classify parts according to the risk of becoming obsolete. Items were classified as “high risk,” “medium risk,” and “low risk”. Here’s where AI comes in.
After classifying the inventory, the company used data labeling to tag the items with one of three risk categories. Having labeled the data, Gopalan and his team embarked on the “first phase” of machine learning: a decision tree algorithm to enhance prediction accuracy. Gopalan claims that this algorithm increased prediction accuracy from 68% to 81%.
In terms of tangible business results, Gopalan claims that the company was able to make its contracts with suppliers more favorable. More specifically, Gopalan indicates that Intel was able to implement more favorable salvage value terms and conditions. He gives the example of the “expected salvage value” of items within a 30- and 60-day period.