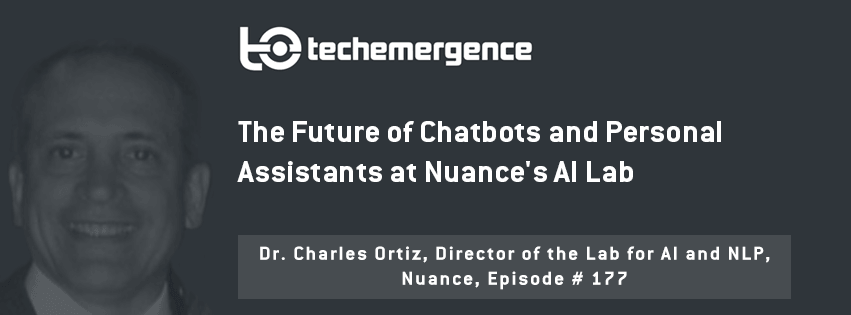
Episode Summary: This week’s interview was recorded live at Nuance’s Silicon Valley office with guest Charles Ortiz, director of the AI and Natural Language (NL) Processing Lab for Nuance Communications in Silicon Valley. In this episode, Ortiz speaks about what he sees as the most important developments in natural language processing (NLP) over the last few years, what advancements brought us to where we are today, and where progress might take NLP in the coming years ahead (both at Nuance and beyond).
Expertise: AI theory and applications, robotics, large scale information and multiagent systems, knowledge representation and reasoning. He spent over 13 years at SRI as the Director of the AI Center’s Teambotics Program before coming to Nuance, where he has been since 2012. In addition to serving as a postdoctoral research fellow (collaborative multi-agent systems) at Harvard University, Ortiz has received his PhD in Computer and Information Science from University of Pennsylvania, his MS in Computer Science from Columbia University, and his bachelor’s degree in Physics from MIT.
Brief Recognition: Charles Ortiz has over 20+ years of experience in AI research and technical management and over 50 peer-reviewed technical publications.
Current Affiliations: Director of Nuance Laboratory for AI and NLP
Interview Highlights:
(1:36) What are the industry technology meta-trends that are allowing speech and NLP to push forward as much as we’re seeing today?
Charles Ortiz: In the same way that robotics became more, there was more investment in robotics, because of things like form factor reduction and processors, the costs went down, and so the universities could purchase robots that they could do research on that didn’t cost a fortune; in a similar way, the work that’s being done in NLP and AI is actually has been facilitated first in this reduction in form factor as well…and also enabled by the advances in speech understanding
Because of that and proliferation of systems like Siri, it’s taken for granted that that’s possible to some extent; what we’re trying to do here is take it to the next step, the next generation of these personal systems
(3:30) Talk a little bit about Nuance’s history in bringing the ball forward to where speech recognition is now.
CO: My lab has been here about four years, we don’t really work in our lab about speech recognition…in the auto domain, the push to introduce personal assistants into the car, that’s a natural domain of speech and language because of the need to reduce risk and ensure that a driver’s cognitive load isn’t stretched to the point where they can’t pay attention to the traffic and so forth. People want to be able to have access to resources on the Internet, but you can’t be clicking apps with your hands on a cell phone and you can’t be pointing to things except in a very limited way; this means that speech is a natural medium for communication in that kind of domain, but what we need to do to take that further is think about how to model the tasks and solutions of the tasks the drivers or users are interested in, so this is not just cars, but I give that as one example…
Our systems have to become more proficient in being able to understand what the user wants done and not just in terms of an answer, like “what’s the temperature outside?” It’s more that the user wants to do something, say reserve a table somewhere for dinner or they want to find a store that has something in particular…you have to take the natural language processing in the front and carry it forth to the backend, which is responsible for doing the reasoning about the task.
(6:36) I imagine you have to go through a lot of the uses case in AI…and think through what are the common situations of intent….is it calibrated per user, is it calibrated across conversations…how do we get closer to the next step – understanding intent?
CO: There are a couple of thrusts in the work that we’re doing that’s driving what works. First, I should say that the focus is primarily on conversation and dialogue as opposed to one-shut utterances – this would be “what’s the capital of France” or “who owns the Golden State Warriors”, that’s a question you would get an answer and you’d be done with it; If I’m using a search engine and I I ask a question, the next question doesn’t have anything to do with it, that’s not a natural way to communicate with a human, language has some very useful properties and it’s very efficient, you can communicate a lot without saying much, and that’s by virtue of being able to carry around this context of a dialogue; if Im planning a trip and trying to reserve some flights to particular cities on particular days and then I switch to trying to get a hotel reservation, I don’t need do repeat where or what day, etc., and that’s a simple example
…in terms of domains, theres’ a big challenge here that we’re trying to address by making systems more transportable to new domains by virtue of having a lot of background common sense knowledge, this is a big challenge in AI, it’s not something that’s going to be solved tomorrow but we’re working towards incorporating more of this world knowledge so that systems can be easily engineered for particular domains
(12:49) Talk about common sense knowledge…some of that would be maybe if-then scenario, logic based, I Imagine some is teased out from failures and successes…how have you gone about approaching common sense knowledge for machines?
CO: What we’re calling it is big knowledge to distinguish it from big data because it focuses on more general information; it’s a very challenging problem…there are three basic ways you can do this, and we’re taking a sort of hybrid approach to building these knowledge bases.
One way is of course by hand,…another is to do crowdsourcing where you have systems that everyday people can contribute to….and then you can learn as well, try to learn or extract knowledge from a document on the Internet, for example, or some other source. What we’ve done in our approach is build the first framework where we could support these three forms of knowledge of acquisition and be able to do things like deconflict between one representation and one another, because you’re going to have some cases where some of that information in one conflicts with another, or the names aren’t exactly the same – they refer to the same things, but they don’t use the exact same names for whatever reason, so we have that framework in place and then we’re making use of all 3 types of knowledge…
We’re not claiming that we’re going to have in a couple of years the big knowledge database of all common sense knowledge, but what we’re trying to do i,s in the domains of interest to the business in which Nuance is involved, we have a sufficient backbone of world knowledge, so that we can then make our system more robust
(18:21) You’re thinking a lot about the future of this technology….in terms of where you see the possibility for transformative impact in that improvement of speech technology…where do you see 2 to 5 years where this could really take off and have a grip?
CO: In any case, the main advance is going to be conversational systems, dialogue-based systems that can engage with a user in a multi-utterance interaction, because that’s the way you and I talk, and we don’t want to try to teach people to talk in a different way; being able to support a dialogue is going to be one major advance that we see, and we’re focusing our efforts there, and the other is imbuing systems with more and more of this world knowledge, common sense knowledge, and that’s going to make systems slowly, but hopefully surely, more robust and it is one of the big challenges in AI…
I should mention…there’s been an effort in the last few years to come up with better measures of progress in AI, and these are in the form of alternatives to what’s called the Turing test, and one of those that we’re supporting has been promoted by the AI research organizations, the Winograd Skeema challenge (WSC) and it is meant to do two things…help measure progress in AI in this very challenging area and get more of academia involved, in terms of professors and students, in pushing the technology forward.
Related Chatbot and Speech Recognition Interviews:
- How Natural Language Processing Helps Mattermark Find Business Opps (Samiur Rahman, Data Engineering Lead with Mattermark)
- How Will the World Be Different When Machines Can Finally Listen? (Adam Coates, Director of Silicon Valley AI Lab for Baidu USA)
- Closing Gaps in Natural Language Processing May Help Solve World’s Tough Problems (Dan Roth, Professor at University of Illinois at Urbana-Champaign)