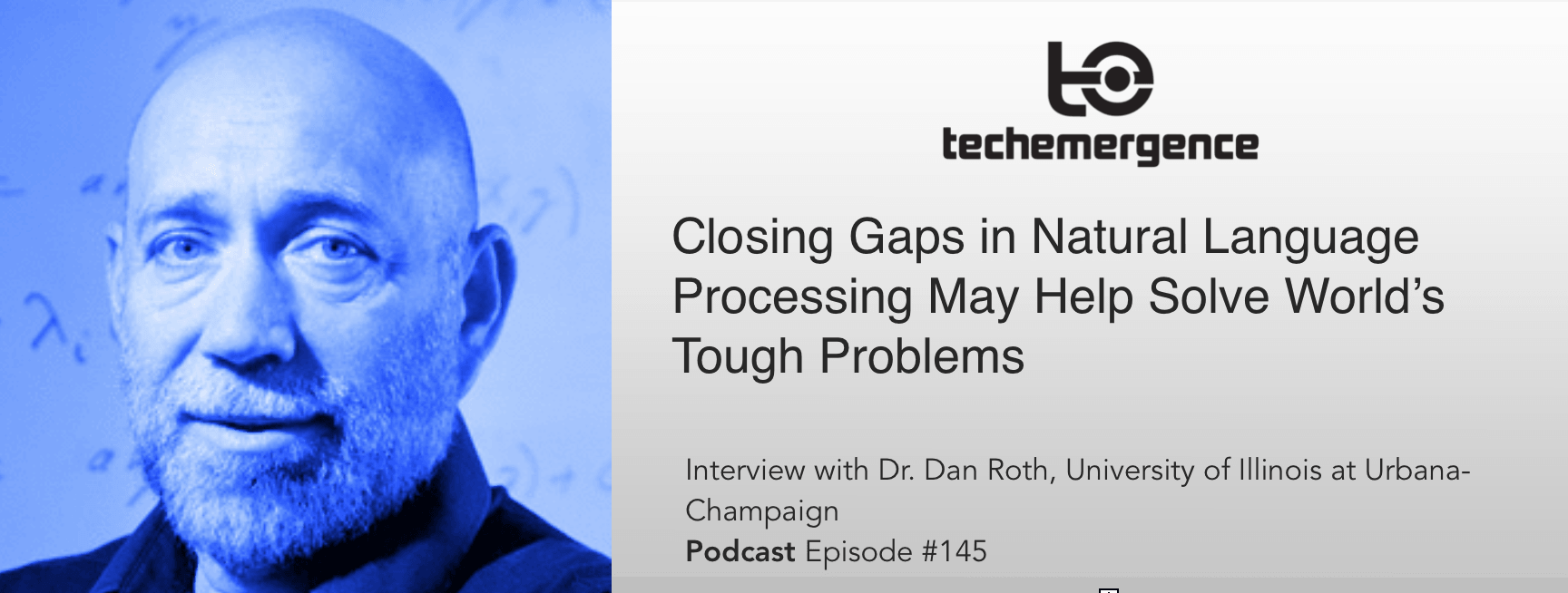
Episode Summary: People often mark progress by what they see, but there’s often much more going on behind the scenes, the up and coming, that marks actual current progress in any particular field. The same can said to be true for natural language processing, and Dr. Dan Roth’s research in this field makes him privy to the advancements that most of us are bound to miss.
In this episode, Dr. Dan Roth explains what the last 10 years of progress in natural language processing (NLP) have brought us, what’s happening with approaches in developing this technology today, and what the next steps might be in a computer capable of real conversational speech and understanding language in context.
Guest: Dan Roth
Expertise: Machine learning and natural language processing
Recognition in Brief: Dan Roth is a Founder Professor of Engineering at the University of Illinois at Urbana-Champaign. He is a Professor in the Department of Computer Science and the Beckman Institute and holds faculty positions also at the Statistics, Linguistics and ECE Departments and at the graduate School of Library and Information Science. Prof. Roth got his B.A Summa cum laude in Mathematics from the Technion, Israel and his Ph.D in Computer Science from Harvard University. He has published broadly in machine learning, natural language processing, knowledge representation and reasoning and learning theory, and has developed advanced machine learning based tools for natural language applications that are being used widely by the research community.
Current Affiliations: Fellow of the American Association for the Advancement of Science (AAAS), the Association of Computing Machinery (ACM), the Association for the Advancement of Artificial Intelligence (AAAI), and the Association of Computational Linguistics (ACL); Editor-in-Chief of the Journal of Artificial Intelligence Research (JAIR)
The Wild Frontier of Natural Language Processing
Most people see applications like Siri, Google translation, and Google search, and know what it’s like to interact with these softwares using text and voice commands. But there are also a great many burgeoning technologies, including text mining and text analytics tools, that allow us to extract information from free text and use as structured data for database technology. “We can look at collection of millions of media and text documents and process them; there has been lots of progress in shallow natural language processing (NLP) technology which now is common, and there’s a lot more to come,” says Roth.
With all of the progress that AI and machine learning is making today, why are we still in the shallow end of the pool with NLP? “People do not realize how difficult the problem is; any word you want to take in English, take the word table for example…may mean different things and parts of speech in different contexts,” explains Dan. Machines face a complex set of cognitive tasks that require a lot of knowledge, something that we too often take for granted.
Roth suggests that conventional programming (the typical “if-then” statements and defining variables) is not good enough with NLP, which requires combining learning techniques with reading and listening to lots of data, all of which must be understood in context – a monumental task for a machine with no built-in background knowledge.
And much of the technology that we might assume is NLP is often barely scraping the surface of this technology. Google Translate, for example, can find a word similar to it in another language, but it does not understand text, it won’t answer a question, and it cannot help you beyond simple translations. Humans (more often Americans) can refer to the last Thursday of November or use the term Thanksgiving, and both are understood to represent a national holiday, but Google doesn’t understand this idea. What is trivial for people is still a huge gap that needs to be closed machines.
To solve the problem of context, the approach being taken today by many researchers is two-fold: the first prong is machine learning, which reads through a lot of text and tries to understand abstractions and learn from masses of data, and the second is acquisition of knowledge, a lot of which can be done automatically through well-organized, human-made sources like Wikipedia, for example.
Writing programs that can learn from both methods and better understand the ‘meaning’ of text is a line of work that many are pursuing, says Roth, and it’s called ‘indirect supervision‘. “We’re trying to catch signals of supervision wherever we can, minimizing human effort, using annotated information to develop algorithms to better understand data,” he explains.
When we think about computer vision and machine learning, there are programs able to sort through types of images – cars, for example – based on what it knows about cars: this is the front, this is the side, these are the wheels, etc. Humans still manually edit any images that machines identify falsely, and then feed it back to ‘teach’ the machine.
The cycle of labeling and training to identify is very similar to NLP, only there are many more stages and much more nuanced information to teach. Parts of speech, categories of phrases, inferences – these are just a few of the factors that a thinking machine must take into account. If we’re to achieve a computer that can listen to and understand the meaning of speech, even in simplified language, then there has to be real effort in trying to minimize human supervision.
Blazing the Trail Ahead
In the next 10 years, access to information may be elevated to a new level with significant progress in the field of NLP. “I can see us being able to communicate with computers in a real natural fluent way, much better than Siri,” says Roth. A middle schooler trying to solve a difficult problem in algebra may be able to consult the machine on strategies for solving an equation. Firms involved in a big legal case, where it often takes many lawyers and thousands of hours to look through millions of documents to think about a legal issue, may be able to much more quickly provide an intelligent summary with evidence pointing to specific documents and correspondents.
There are many more areas in which NLP may revolutionize the way we interact with each other and our machines, from customer service to smart toys. At present, some of the greatest challenges for NLP involve medical and compliance information, due to the sheer volume of documents that need to be accessed in an intelligent way. “Articles printed in biomedical last year were over a million, that means no one knows what’s happening,” says Roth. But the next decade may see advances that allow physicians to verbally request relevant research articles that pertain to a specific medical issue.
The potentials here are endless, but one example might be a medical practitioner trying to determine the influence of a certain drug on a particular gene, something that may require unnecessary experimentation in a lab if he or she is not aware of existing pieces of information that could help answer tough questions. If physicians and other experts have better, almost real-time access to the information that they need to know, then humans will be much better poised to figure out some of the most difficult questions that still plague our global society.
Image credit: University of Illinois