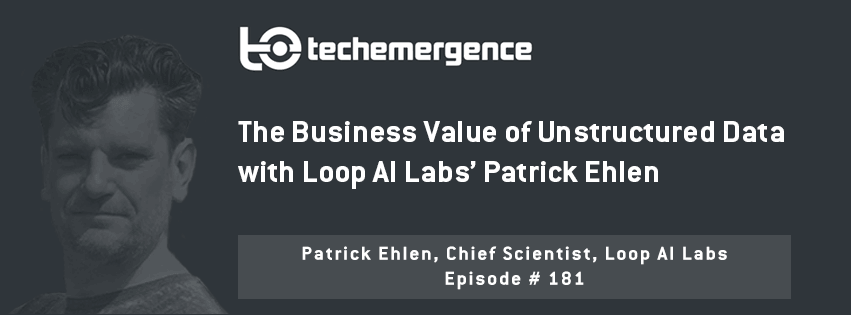
Episode Summary: Our guest in this episode has spent a large part of his life on figuring out how to make machines more intelligent. Loop AI Labs‘ Chief Scientist Patrick Ehlen has worked on a number of important projects, from DARPA projects to big-company AI solutions at places like AT&T. Loop AI works on getting AI to make sense and meaning of information the way that humans do, like making meaning of unstructured text. Ehlen talks about the potential business applications for this technology and where it’s making way its way into industry. Ehlen also touches on the implications for developers in the nascent AI field – like Loop AI – that are vying to implement its technology as an industry standard, and how such organizations will have to market themselves and deliver services to develop a thriving AI ecosystem.
Expertise: Machine learning and speech recognition
Brief Bio: Patrick Ehlen is Chief Scientist at Loop AI Labs. He earned a PhD in Cognitive Psychology from the New School for Social Research in 2005, where he was awarded the Dissertation Fellowship and was the first recipient of a Charles Cannell Fund award from the University of Michigan for his work on mathematical modeling of concept processing. While completing the PhD, he interned at speech recognition pioneer Dragon Systems (acquired by Nuance) and then at AT&T Labs-Research.
He then went to the Center for the Study of Language and Information (CSLI), an AI mecca at Stanford University. As a research scientist in Stanford’s Computational Semantics Lab, he worked on the $200M DARPA CALO AI project and devised machine learning methods to extract concepts and topics from ordinary, spontaneous conversations. In 2008, he returned to AT&T in San Francisco to join a fledgling R&D group at AT&T Interactive, where he continued to advance the state-of-the-art in natural language understanding technology, first by improving semantic similarity in the YellowPages search engine.
Patrick joined LoopAI in 2015 after seeing the potential applications in incorporating deep learning into their approach. He has been awarded four U.S. patents and produced 45 research publications in the areas of computational semantics, cognitive linguistics, psycholinguistics, word sense disambiguation, human concept learning, and artificial intelligence. His work is cited in over 430 scientific papers.
Current Affiliations: Chief Scientist at Loop AI Labs, Inc.
Interview Highlights:
(1:32) The first question I wanted to run off of you…is in dealing with gargantuan sets of unstructured text data…what are the business use cases?
(8:20) Clearly there’s a lot of business domains where such (dark) data exists…what are one or two other areas where there are patterns with real business value with this unstructured data if someone had a system to do so?
(14:08) You’ve been working with big companies, very interesting projects…clearly had to know your science deep enough…but also communicate this to people who haven’t gotten a post-doc…when you find yourself articulating what artificial intelligence is doing…what have you had to learn about conveying what’s happening and what the value is?
(19:16) What do you find are sometimes the gaps between what you know a business or institution would need to get something out of their data, and maybe what they think it takes?
(21:19) What are the areas that you find companies or executives maybe don’t understand how the process would work…we’re talking about something that at this point, the raw materials are so varied company to company…what do you think are some of the misconceptions around how this is going to work for us as executives?
(26:10) This world of leveraging machine learning and big data…it feels like there are a lot of companies that have machine learning approaches that could apply across the board early on…but it seems as though the field is not…to the point where you can really compare vendors in any way…how do you see the industry evolving in this world of big data?
(32:12) Do you see people sort of owning verticals or owning horizontals…things that work across industries…do you see niche-ing, for marketing or technology reasons, being something that helps this current globular world (of AI technologies) have some big building like the Google’s and IBM’s of the world?