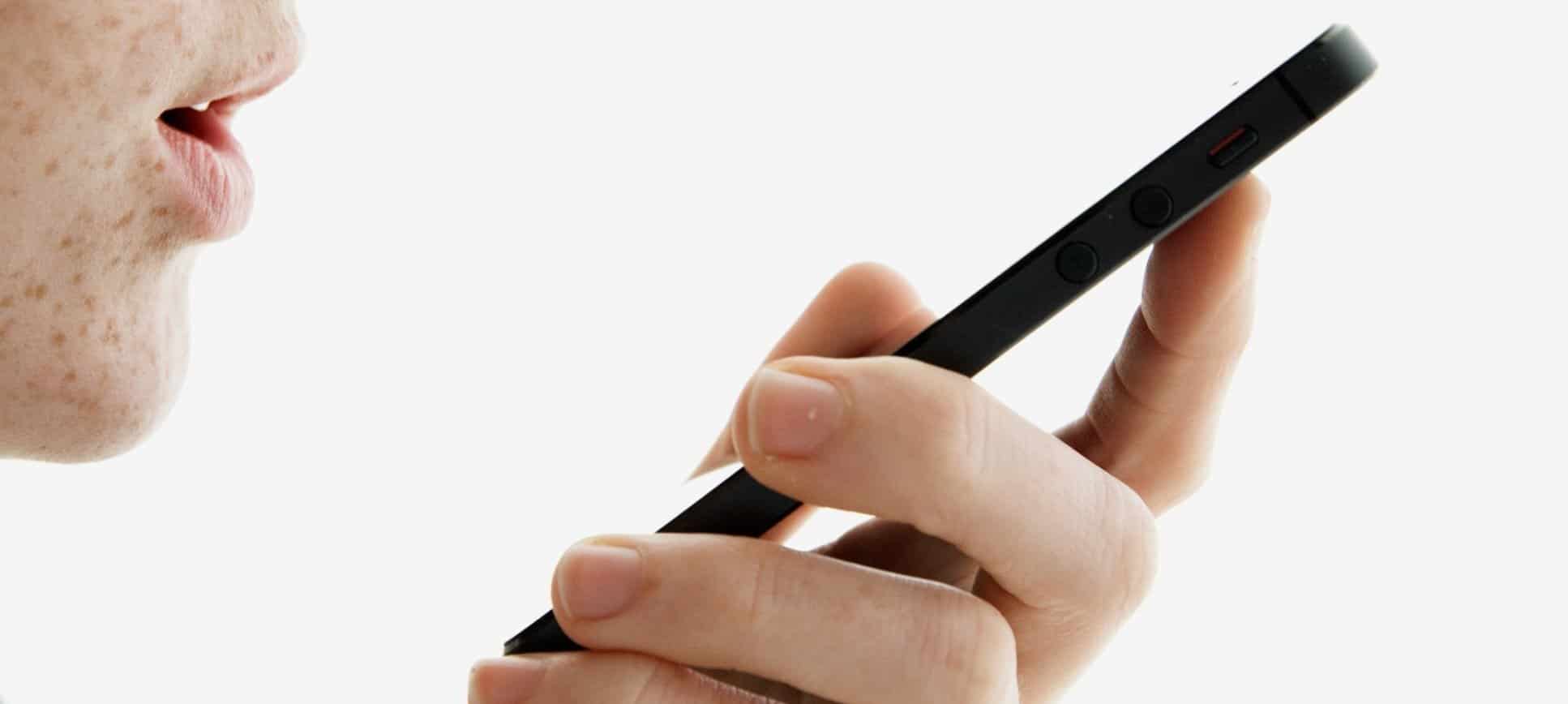
Episode Summary: In this episode of AI in industry, we speak with Michael Johnson, the director of research and innovation for Interactions llc, in Boston MA. Michael explores the inbound (human to machine) and outbound (machine to human) applications of voice based natural language processing (NLP) and also talks about attaching a time-frame to how soon small and medium enterprises (SMEs) would have access to this technology in a financially sensible manner.
Although NLP is often associated with chat or text interfaces, voice is important for applications in call centers, mobile phones, smart home devices, and more. In addition, Michael explains that voice involves unique challenges that text does not have to deal with – including background noise and accents, which need to be overcome to deliver a good user experience.
Subscribe to our AI in Industry Podcast with your favorite podcast service:
Guest: Michael Johnston, Director of Research and Innovation for Interactions LLC
Expertise: Linguistics, Natural Language Processing, Natural Language Understanding, Semantic tagging, Spoken and Multimodal Dialog Systems, Virtual Assistants, Human Computer Interaction
Brief recognition: Before going on to work with Interactions, Michael acquired a Ph.D in linguistics from UC Santa Cruz . Michael went on to work with AT&T as a principal inventive scientist from 1999 to 2014 where he was responsible for AT&T’s research and development program in interactive multimodal virtual assistants.
Current Affiliations: Michael is the director of research and innovation at Interactions LLC. He is also serving as the Chair of the W3C Multimodal Extensible Multimodal Annotation group since 2003.
This interview is part of a month-long series of podcasts about natural language processing, sponsored by Nuance Communications. Learn more about our content and promotional services on our partnerships page.
Big Idea
Michael explores a few commercialized business use-cases and also delves into the challenges facing any business looking to apply spoken voice based NLP in customer service or customer-facing tasks. We start by looking at the currently commercialized applications, explore an NLP business use case, identify the challenges in inbound and outbound voice based NLP, and lastly move on to what we can expect in terms of a technology disruption in the near future.
Businesses looking to apply voice-based NLP need to take into account the data-intensiveness of their applications. In cases where there exists a massive set of well structured data, NLP systems can often perform well with making sense of language. Without a certain volume and uniformity of data it is often challenging to get these systems to work well.
Current Applications
One of the primary applications for voice based NLP currently is in telephony for enterprise applications. A similar growth in usage of voice based NLP in home and mobile settings has also aided the growth of this technology into a mainstream AI communication application. The telecom sector, finance sector and the hospitality industry are among the first adopters of voice based NLP in applications – such as routing incoming telephone calls.
One such example for current NLP platforms in business use cases (telephony in this case) is Interactions’ solution for global hospitality group Hyatt which was accomplished by way of the following steps:
- Hyatt determined the “low hanging fruit” common questions and situations handled by their employees, including simple, common tasks such as setting up a reservation or finding information about reward points.
- An AI solution was modelled based off of these fundamental question types, building a wide and representative set of examples of voice requests related to this core set of simple tasks.
- The system was put in place and trained to understand simple commands and route calls directly to humans capable of handling those requests.
In the next decade, we can also expect smaller enterprises without access to large amounts of data to license pre-trained AI platforms and tweak them to fit their needs. For example, if an NLP company working with dozens of hotel chains can determine common patterns among all of them, these “patterns” could be used to jump-start call routing technologies for smaller firms who lack the data to train their own algorithms.
Challenges in Voice (as Opposed to Text)-Based NLP
Spoken voice based NLP inherently brings with it challenges on two fronts, the inbound side (hearing users) and the outbound side (“speaking” back to users). On the inbound side, spoken voice inputs are made more complex by accents, background noise, talking at the same time, etc – and the need to communicate in a manner which is familiar with the audience.
The additional layer of spoken voice outputs in voice based NLP as compared to text based NLP, creates challenges in the outbound side; for example, speaking with correct intonations, correctly conveying words with emotion, and more. Overcoming these challenges would involve an additional layer of training for voice-based NLPs; one way to configure voice-NLP agents would be to add human assistance to the data-based training process. This would enable platforms to start off with a much higher level of accuracy than agents trained purely by feeding in datasets.
A Look at What’s Coming in the Future
In terms of social factors, the mainstream acceptance of talking to home devices (such as Amazon Echo or Google Home) is making speech and voice based communication more common. Yet, simultaneously, this is also leading us to a future environment which favors devices with multimodal capabilities such as home devices and wearable electronics where audiences interact through multiple channels (like speech recognition integrated with touchscreens/gesture recognition) of input. Such integration of technologies is inherently challenging currently, but with improved processing capabilities we can expect them to come to fruition in the near future
Interview Highlights
The most important questions that Michael answered during this interview have been listed below. Listeners can use the embedded podcast player (at the top of this post) to jump ahead to sections they might be interested in:
- (2.57) While applications like sales support or customer service are pretty commonplace for chatbot interfaces, can you tell us a common business use-case for spoken voice based NLP application and how its implementation would look like?
- (4.01) What are the voice-specific challenges for businesses looking to deploy voice based NLP platforms? (as opposed to text-based NLP)
- (8.01) In terms of training, how critical is the volume of input data for the NLP platform to accurately respond? In cases where there exists a lack of sufficient data, (read SMEs) what additions to the voice NL process are required to address this?
- (13.53) What can we expect in terms of disruptive technological changes in the voice based NLP space in the near future? Can you give us a technology roadmap of sorts?
Subscribe to our AI in Industry Podcast with your favorite podcast service:
Headline image credit: TheUnlockr