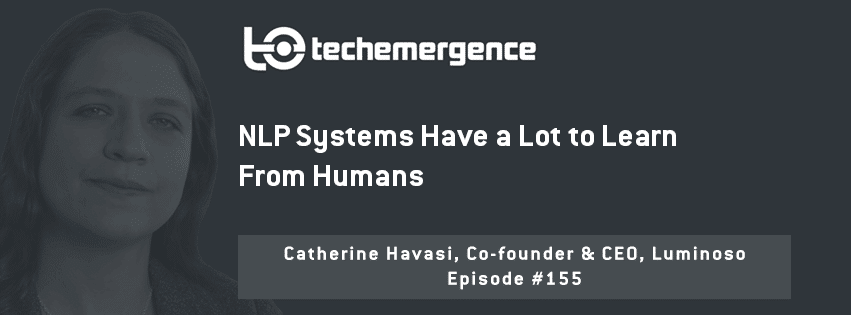
Episode Summary: Not more than 10 years ago, it would have been difficult to talk into your phone and have anything meaningful happen. AI and natural language processing (NLP) have made large leaps in the last decade, and in this episode Dr. Catherine Havasi articulates why and how. Havasi talks about how NLP used to work, and how a focus on deep learning has helped transform the prevalence and capabilities of NLP in the industry. For the last 17 years, Havasi has been working on a project through the MIT Media Lab called ConceptNet, a common sense lexicon for machines. She is also Founder of Luminoso, which helps businesses make sense of text data and improve processes, and one of a handful of female leaders in the AI field who we’ve had the privilege of interviewing in the past year.
Expertise: Common sense reasoning and natural language processing
Recognition in Brief: Dr. Catherine Havasi completed her undergrad degree at MIT and received a PhD in Computer Science from Brandesi University. In 1999, Catherine began working on a project at the MIT Media Lab to collect common sense from volunteers on the internet. Since then, the Open Mind Common Sense project (OMCS) has expanded, with sites in Korean, Japanese, Portuguese, and Dutch. With others in the Common Sense Computing Initiative. She also maintains the semantic network ConceptNet and works extensively on AnalogySpace, which makes rough conclusions about new common sense knowledge. Catherine has contributed numerous scientific articles to various publications, and won the 2007 Xerox award for best PhD student paper on semantic processing. She is also co-founder and CEO of the AI company Luminoso.
Current Affiliations: Research Scientist at MIT, CEO of Luminoso
Interview Highlights
(1:34) NLP has been around for quite some time, having machines make sense of text probably going on before our births – what are some of the traditional approaches to NLP?
(3:36) Where was older NLP used with what we might call coarse methods of rules-based systems and taxonomies?
(5:33) You mentioned search engines, Korean languages that don’t involves spaces, where are some other areas where this kind of NLP (is being used), where right now, somewhere, someone in a company is cranking away?
(7:05) Where is the (recent) shift (in NLP) towards i.e. taxonomies, rules-based systems are now shifting into what?
(10:30) What does it look like to train a machine learning program around NLP? Can you give me a tangible, step-by-step example where this could be used or you’ve seen it used yourself?
(15:41) What you were just getting into, I think was the unsupervised side of things…we’re having a machine that’s making sense of the machine by itself in the NLP context, how is that even possible for us laypersons to understand?
(17:30) In the context of language, what does that look like – we’re sending enough sentences, sound bites through a series of neural networks, how are those connections then being made without the instructions being put in, in the first place, how does that work in real time?
(22:08) What are some tangible yields here from getting NLP right? How can this kind of machine learning approach be leveraged fruitfully in an industrial environment?