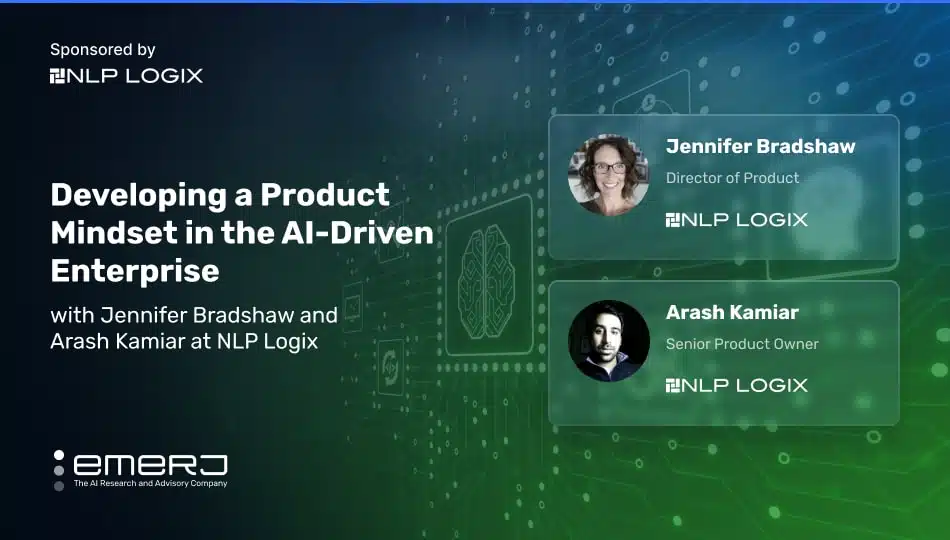
This interview analysis is sponsored by NLP Logix and was written, edited, and published in alignment with our Emerj sponsored content guidelines. Learn more about our thought leadership and content creation services on our Emerj Media Services page.
It is no mystery that AI and machine learning investments continue to increase across industries. Per the global market intelligence firm IDC, the top 2000 organizations globally will allocate over 40% of their IT spending toward AI in 2024. By 2027, the same sources anticipate AI spending will reach about half a trillion dollars.
While some may translate this surge in organizational AI spending as evidence of the AI’s efficacy, research is disproving this interpretation. In fact, many organizations need external perspective before achieving a return on their investments in AI initiatives. Many fail to realize the anticipated benefits, while others still do not even manage to recoup their initial investment.
How can executives ensure their technology investments are directly tied to strategic business outcomes? What criteria can they use to evaluate the potential economic impact of new tech projects? What role does education play in determining the relevance and effectiveness of AI projects?
In the following analysis of business insights from conversations on the ‘AI in Business’ podcast, we take a closer look at our recent conversation with Jennifer Bradshaw and Arash Kamiar of NLP Logix, two customer-focused product development professionals with wide-ranging experience helping organizations understand, evaluate, and leverage AI technologies. Our analysis hones in on the practical insights shared by Jennifer and Arash that can assist any executive in determining whether an AI project at their organization is viable and profitable.
We examine three actionable takeaways therein:
- Embracing the “Product Mindset”: Choosing AI technology based on its ability to solve specific business problems rather than novelty or sophistication ensures the investment yields practical, value-driven results.
- Assessing economic impact: Assessing economic impact first can provide a clear understanding of the potential ROI, helping organizations avoid mainly AI ventures that don’t offer tangible benefits.
- Making data-driven decisions: Familiarization with AI and machine learning capabilities can aid the organization in understanding if and how any potential solutions are relevant and effective for the specific challenges faced.
Listen to the full episode below:
Guest: Jennifer Bradshaw, Director of Product at NLP Logix
Expertise: Product management, business analysis, process improvement
Guest: Arash Kamiar, Senior Product Owner at NLP Logix
Expertise: Product ownership, agile project management
Embracing the “Product Mindset”
In Emerj’s discussion with Jennifer and Arash, the focus was primarily on the essence, importance, and consequence of what they describe as a product-centered mindset. The insights in such a framework are two-fold:
- Grounded in business acumen and a prescient understanding of industry pain points, as opposed to:
- Investing in technology for its own sake.
At the heart of the product mindset is the focus on investing in and integrating any technological solution must be based on its ability to solve problems.
As introduced by Emerj Senior Editor Matthew DeMello serving as podcast host, Jennifer invokes the famous exchange of Steve Jobs at the 1997 Worldwide Developers Conference. When then-returning Apple CEO Jobs was challenged on the decision to discontinue the software platform OpenDoc, he emphasizes that what matters is whether the technology solves the client’s problems, not its technological superiority.
Developers often hyperfocus on creating the best, most potent hammer (technology), while business leaders are more concerned with any solution to their nail (business problem). In simple terms, the variety or sophistication of the hammer used doesn’t matter so long as the nail is no longer a problem. Through the pair’s work at NLP Logix, Jennifer and Arash describe how they help design solutions that strive to maintain a sharp sense of where and when to direct technological efforts, starting from business problems and working toward the data.
Arash states that a balance is required, “You have to stay focused on what the latest technology is, but in a way that aligns in a way with solving real-world issues.”
Jennifer continues to elaborate on their company’s approach by discussing the vital importance of understanding customers’ strategic objectives. She offers a relatable analogy: going to market with products not informed by data-based approaches to strategic customer objectives is like going grocery shopping while hungry without a list. You end up with too many things you don’t need. As the decision to invest in a technology approaches, Jennifer advises narrowing down product choice by asking a few value-defining questions:
- Does this technology solve a specific, industry-based business problem?
- How does the technology align with our organization’s strategic objectives?
- Is this technology essential for solving our problem, or is it just a nice-to-have?
The central lesson of the exchange with Jennifer and Arash is clear. When evaluating which technology to invest in, one must first and foremost clearly identify the business problem or strategic objective it addresses. Doing so ensures:
- The problem is addressed.
- The organization does not spend frivolously on a solution that does not meet business requirements or align with strategic objectives.
Assessing Economic Impact
Arash then brings the conversation to the critical importance of organizations evaluating the economic viability of a technological solution prior to investing. Moreover, Arash implies here that vendors don’t necessarily have the best interests of their clients in mind. To illustrate, he references an MIT study that suggests less than 25% of computer vision models currently being developed are economically beneficial for customers. It should also be noted that the study concludes that it could take “decades” for computer vision tasks to become financially viable for firms, even when taking into consideration rapid decreases in costs.
To properly evaluate the viability of a solution, organizations must first have a clear objective. Is it to reduce costs? Generate revenue? To align with strategic initiatives? To expand into new markets or demographics? Better operational efficiency? Failing to formulate such objectives can lead to what the pair calls “solutionism” – developing or investing in technology for the sake of having it rather than to meet an identified need – which may lead to frivolous and economically unsound investments.
However, organizations must go further than determining if a technology solves a business problem and aligns with core business objectives. Even when a technology meets these criteria, it may simply not be the right time to invest. A broader economic context must be considered before deciding whether to commit the necessary resources. AI investments are unlike investments in IT or any other operational area for many reasons, but high among them is that AI investments do not necessarily always lead to immediate cost savings. When considering total costs, leaders must take into consideration other expenditures such as:
- Change management and adoption costs: It is crucial to take into account costs associated with change management and adoption of new AI technologies, such as new hire salaries, training/re-training, process re-engineering, implementation disruptions, etc.
- Continuous monitoring and adjustment: Ongoing evaluation of the technology’s economic impact post-implementation is crucial to ensure that stated objectives are being realized. If said objectives are not realized, strategic adjustments – up to and including divestment – may be required to cut losses.
Understanding the economic implications of AI investments is just the beginning. As Arash’s insights and the referenced MIT study indicate, the decision to invest in technology must be grounded in a thorough evaluation of its economic viability. This includes considering not only the alignment with strategic objectives and the broader economic implications but also the organizational readiness to manage and adapt to the technology over time.
With such complexities in play, it’s evident that a deeper understanding of AI itself becomes indispensable. At such a point in adoption, organization-wide education on AI’s capabilities, strengths, limitations, and potential impact become foundational for a new kind of data-driven enterprise.
Leveraging Data and Making Data-Driven Decisions
Leveraging data is crucial for agile decision-making – a critical element of the “product mindset.” Moreover, to effectively scale AI with a product mindset, Jennifer and Arash both emphasize that organizations must leverage data at every stage, from evaluation to post-implementation.
In the early stages, Jennifer and Arash tell the Emerj executive podcast audience that developing in-house data analysis proceses helps decision-makers:
- Understand the specific needs and challenges the organization faces
- Help in creating a clear set of requirements for the AI solution
- Understand the feasibility and limitations of prospective AI solutions by simulating outcomes and analyzing results from test cases
- Anticipate potential implementation challenges through predictive analytics and lessons learned from past projects (if any)
Once the AI solution is implemented, the pair insist that ongoing data analysis is crucial for:
- Gaining insights into how an AI solution is performing in the real world by monitoring metrics and user feedback
- Iterating and refining the solution based on real-world performance data to meet organizational needs more effectively
- Refining the solution to better align with evolving corporate initiatives
Arash mentions that flexible decision-making is another benefit of the data analysis process, with the ability to pivot strategy based on insights in a critical organizational capability. Once an organization implements an AI solution, continuous data analysis becomes a cornerstone for sustaining such agility in decision-making. The ongoing process then enables organizations to:
- Respond swiftly to changes in user behavior or market dynamics
- Optimize AI models for accuracy and efficiency as more data becomes available
- Make informed adjustments to AI-driven processes and customer interactions
Ultimately, leveraging the process of continuous data analysis serves an organization well both before and long after a solution is ready to put into use. Both guests emphasize that data-driven decision-making is also agile decision-making, which allows organizations to align operations – both AI and non-AI related – to shifting dynamics. Finally, they assure the Emerj podcast audience that the process also helps to ensure that any future AI solution evolves in tandem with the organization’s strategic vision and operational needs.