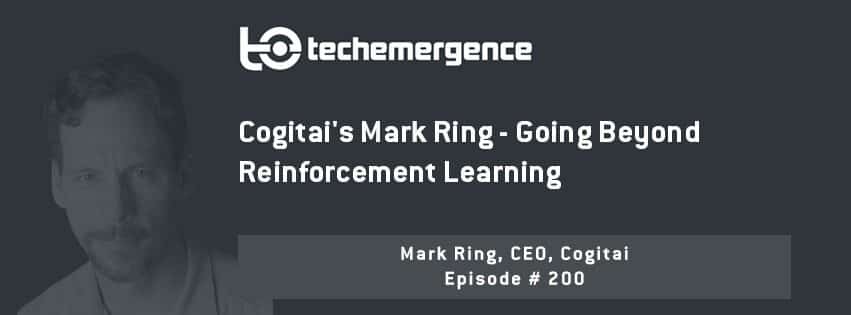
Episode Summary: Today’s episode is about continual learning, a focus of Cogitai, a company dedicated to building AI’s that interact and learn from the real world. Cogitai’s Cofound and CEO Mark Ring talks about the differences between supervised and reinforcement, and how Cogitai intends to take reinforcement learning in the direction of continual learning. Ring also touches on where he sees an opportunity for applying continual learning in domains like vehicles, consumer apps, etc., and improving abstract levels of understanding by machines.
Expertise: Artificial intelligence
Brief Recognition: Mark Ring, PhD, is Co-founder and CEO of Cogitai, Inc., an AI startup focused on continual learning. His research revolves around a single focus: Continual Learning in Artificial Intelligence, which tries to answer one question: If you can give an agent a single algorithm at its inception and then stand back and let it learn forever after that all on its own, what do you put into that algorithm to allow the agent to continue to learn, develop and improve forever? He has published numerous papers on the subject and presented at AAAI’s annual symposium and IEEE’s International Conference on Development and Learning, among others. He received a BA in Computer Science from Vassar College and his PhD in Computer Science from The University of Texas at Austin.
Current Affiliations: Co-founder and CEO of Cogitai, Inc
Interview Highlights:
(1:15) You had talked a little bit about the distinction between supervised and reinforcement learning…clarify that distinction for the people tuned in…
(6:30) How do you put the term continual learning in a nutshell – I know it’s a big part of what you’re doing.
(10:45) Hypothetically if we’re using the example of cars, I can see how reinforcement learning – I imagine – is already paying a good role…in terms of staying in the proper lane, etc…what would that look like if it went a step further, if cars could learn more from the environment itself…would it have a deeper understanding of roads themselves, about active driving…what might be knocked up a notch if we went further than reinforcement learning?
(15:38) There’s a lot of talk…in the bot space…that maybe at some point you have machines that calibrate to certain situations…help me think of where the chat-bot could go beyond current reinforcement learning…
Big Ideas:
1 – Continual learning is about developing a deeper understanding and formulating environmental context based on sequential, cause-and-effect experiences. An autonomous car that could ‘think’ on a more abstract level could potentially make better predictions about what is running across the road (a child versus a dog chasing a ball), and could in turn make better (albeit more difficult) decisions. The notion of learning from experience is an interesting concept that we explored in a previous interview with Dr. Vincent Müller, who believes that intelligent embodiment is a necessity for a genuine artificial intelligence.