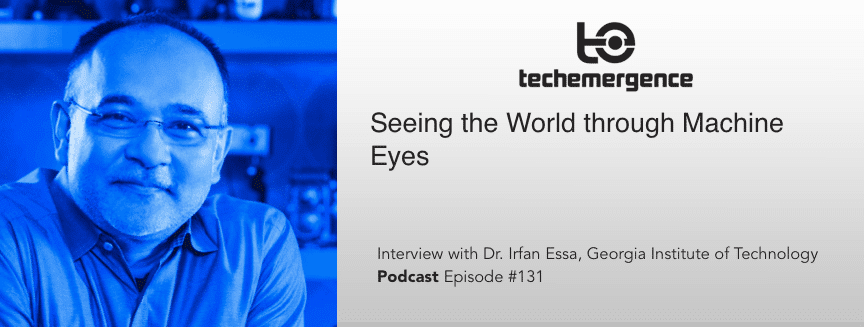
Episode Summary: Most of us forget that just about a decade ago, Facebook’s software was incapable of tagging people in a photo, but today can so without difficulty, sometimes without us even knowing. Machine vision has progressed to the point where it’s also common for computers to be able to pick out dogs from cats in images, another task that was not possible 10 years ago.
In this episode, we talk with Dr. Irfan Essa, an expert in Computer Vision at the Georgia Institute of Technology (GA Tech), about progress made in machine vision over the last 10 years, related projects in the works today, and where machine vision may be headed in the next decade.
Guest: Dr. Irfan Essa
Expertise: Computer Vision, Computational Perception, Robotics and Computer Animation, Machine Learning, Social Computing
Recognition in Brief: Professor Irfan Essa joined the GA Tech faculty after earning his MS, PhD, and teaching at MIT (Media Lab). In addition to teaching in the School of Interactive Computing, Dr. Essa is also associate dean in the College of Computing. He has published over 150 academic articles, several winning best paper awards, and presented at numerous conferences. Dr. Essa has been awarded the NSF Career and elected to the grade of IEEE Fellow. Since 2011, he has worked with Google Research as a research consultant.
Current Affiliations: Georgia Institute of Technology (GA Tech)
Building Machine Vision
Much of the progress made in computer vision over the past decade has been in the ability of algorithms to better “understand” the objects at which they’re looking in various images and scenes. We’ve gotten there in part, says Dr. Irfan Essa, by trying to mimic the human vision system, with the ultimate near-term goal of transplanting that technology into more intelligent machines that are aware of their environment and can interact and behave appropriately. He notes that one of the bigger tasks quickly coming down the pipeline is the ability to analyze the sheer number of images and videos available on the Internet.
Bigger strides have also been made in machines being able to identify objects in more dynamic scenes, helped by the auto and tech industries’ push to develop autonomous cars. Such vision systems require cameras and sensors to detect and identify the complex forms and movements of pedestrians, landmarks, and other real-world phenomena.
Irfan states that one of the biggest advances in the past decade involves the overlap of computer vision and machine learning. He notes that scientists spent a long time in an era of aggregation and collecting data, and have recently transitioned to an era of sense making i.e. using machine learning techniques – in particular deep convolutional neural networks – that are scalable with large amounts of data and able to more carefully disseminate the pieces of an image – to identify features in a face, for example, or to differentiate between species and within species. The next step, he says, is for machines to start asking questions and inferring information from the received data.
Machines that See the Road Ahead
Where might machine vision evolve in 10 years? “Places like GA Tech are thinking of taking a more diverse, multi-pronged approach,” says Essa. One prong is continuing to develop a theoretical, foundational framework that addresses how computational entities can be used to deal with large amounts of information. The second prong is applying machine learning to computer version in order to deal with more complex sets of features, and investigating how to use the technology to better understand images. The third prong, explains Irfan, is the availability of an application program interface (API), the tools that make it easier for anyone who has access to data in the Cloud to have the ability to use machine vision technology.
Another area of continued development is in prediction, assessment, and analytics to detect temporal aspects, says Irfan. “How do we start taking a unstructured, ad hoc data stream from the population to understand more about the signal itself and the content?”, asks Essa. The solution may only be found when we apply machine vision technologies in more dynamic instances, such as robots interacting with objects.
For example, if we want to develop a robot that can cook, the robot needs a sophisticated model of how to pick up an object in space and time. This requires more than taking pictures and showing those images to a machine, although the Internet of Things (IOT) could potentially help in this arena. “If the image of the object at which a machine is looking is available on the cloud, with a community seeing and saying something about (that object), and that information is then brought back to the machine, allowing it to infer…this provides more contextual intelligence in an environment,” says Irfan.
Behavioral imaging is yet another growing domain. The ability for machines to watch and analyze videos of people moving, and to then be able to predict the likelihood of what will happen next, could be of great use in many areas, including healthcare.
For example, a machine that could watch an elderly person get up from their chair in a video, then analyze and assess the types of necessary support that an individual likely needs in order to abstain from falling in the near-term future, would be a great leap, says Irfan. There are many relevant and pressing needs in healthcare – Kevin Hartnett from the Boston Globe writes about an app for the blind made possible through advancing computer vision technology.
A GA Tech project about which Essa sounds particularly enthusiastic is applying machine learning and computer visioning to observing children on the autism spectrum, with the goal of predicting underlying factors at an earlier age. Almost 20 years ago, Irfan’s PhD thesis was on building a system that would recognize human expression, and it’s an area that many have worked on since, he explains. “Can we actually observe a person in various types of dialogic situations, perhaps with a caregiver, and how do they react in home situations…can we predict how (the individual) is responding to certain signals?”, explains Irfan.
He and other researchers are interested in observing children with autism work with experts, who know the type of behavioral markers that trigger certain behaviors, information that could then be encoded into a machine used to detect how a particular response is likely to trigger a particular reaction. In turn, this information on early warning signs or triggers could then be provided to a caregiver for better support. “A bigger aha moment for me was…if a machine could actually hear the speech of a child at a certain age, would it be able to identify the types of support a child might need in the future?”, says Essa. Building such a tracking app is another project currently underway at GA Tech.
Is machine vision a necessary factor in better understanding artificial general intelligence (AGI)? “I believe both are connected to the extent that embodiment is part of the paradigm, though the pragmatic part of me says it’s required depending on the task at hand,” says Irfan.
If a robot requires a physical embodiment for its ultimate purpose, then its creators need to build a system that uses vision to react and interpret; but such a machine will likely also need to leverage more targeted abilities, like asking a question at the right time. Forms of embodiment are a practical issue, says Irfan. If an entity’s use is limited to information on the Internet, then such embodiment is probably not required. One uniform aspect Essa does see crossing all areas of future AGI development is experts working together to make advances across domains.