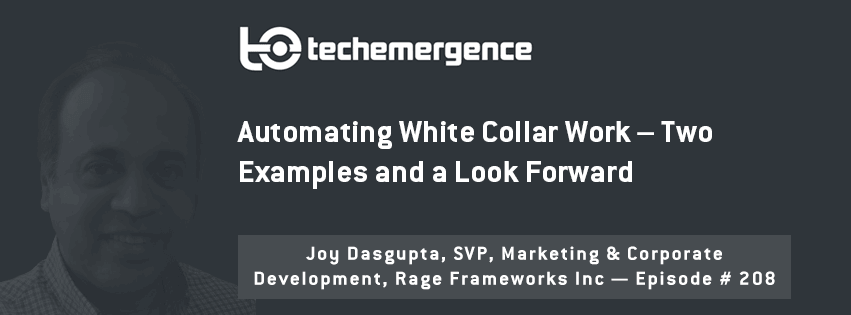
Episode Summary: Not all knowledge work can be crunched by a program, but there are some hard-to-automate business processes that a select few entities are making an attempt to automate now. Boston-based Rage Frameworks, Inc. is one such company, and in this episode we speak with Senior Vice President (SVP) Joy Dasgupta about specific applications of automation technologies applied to white collar environments. Rage Frameworks has developed intelligent machines that have been able to take over process that, prior to the emergence of AI and automation technologies, would have required thousands of people to accomplish. These developments are a microcosm of what is to come, and the process is not without its ethical considerations (as discussed in a previous interview with Yoshua Bengio). But Dasgupta’s insights provide a concrete glimpse into how these processes are being automated in the knowledge workplace today and what that might mean or look like decades from now.
Expertise: Business strategy and transformation
Brief Recognition: Joy Dasgupta heads up Marketing and Corporate Development at Rage Frameworks. Prior to joining Rage in 2012, Dasgupta served as Vice President of Global Business Transformation with American Express, was Founder and Managing Partner of Gestalt Partners, and was a Global Technology Leader for 13 years with Hewlett Packard.
Current Affiliations: SVP, Marketing and Corporate Development at Rage Frameworks Inc
Interview Highlights:
The following is a condensed version of the full audio interview, which is available in the above links on Emerj’s SoundCloud and iTunes stations.
(2:14) Talk us through some common examples of knowledge work that you’ve seen commonly solved by your solutions?
Joy Dasgupta: Today, for example, if you’re a commercial bank and you lend money to small business, you’re going to want to monitor them on a quarterly basis to check on their creditworthiness…and you expand that across the US, and you expand that across the globe, and you will have millions of millions of borrowers who need to submit financial statements to the banks they’re borrowing from for creditworthiness analysis and checks and credit modeling.
The state of the art today is that each and every one of those banks, based on the intelligence that we have and the primary research we’ve done, are entering this information manually…we have an application which we call Life’s Cred that essentially uses one patented component within the overall patented platform, which is the extract component, that can intelligently extract text, financial data, as well as meaning from the footnotes that then go and augment the data that’s there in the body of the statement…
(7:50) What are our inputs (in this scenario)?
JD: The world outside we have to assume is going to be stuck in 1975 for a while, but the world inside the banks has to be 2017…we take in whatever form and format we get; we get attachments to emails, we get Microsoft excel, we get PDFs, we get Quickbooks…an attempt to solve this problem, which has been int he making for years and now is in productions, is the whole idea of this XPRL standard, where financial statement tagging is standardized and therefore at least for public companies downloading their statement, is pre-tagged, so you’ve eliminated the manual work around it, but unfortunately that tagging doesn’t go deep enough..it doesn’t give you the tags within the tags that you need to be able to extract for the details on operating leases (for example)…
(13:40) What’s another good example of something highly repeatable or that you all are streamlining?
JD:…A second use case..in this specific bank for example, they’re sitting on contracts from let’s say the year 1980, millions, and over time these have been amended, amendments have been attached, some of the banks have gone ahead and tried to digitize some of this to have good solidi digital repositories, but they’re unreadable, they’re just scanned images that are kept there, a blackbox, so the challenge is two-fold: one is, can you go back and read all my contracts for me and extract the following 15 clauses, tell me what my indemnification clause looks like, how much in insurance exposure do I have…from legal and compliance perspective, help me understand what I have signed up for, so that’s 100 percent manual
…and then having done that…now that my contract is made into structured from unstructured – we actually think of contracts as somewhat of a semi-structured document – help me with my billing problem, make sure I’m complying…once you’ve got the contract extracted and you’re’ also able to extract invoices, and then match them all on the same platform, that creates a very compelling breakthrough on end-to-end knowledge process automation type solutions…
(19:53) In particular industries where you’ve done a lot of work, do you already have a lexicon built out…or odes it vary a good deal per company…I don’t know how much is transferrable but I’m interested in your insights.
JD: There’s a different use case where it’s 100 percent transferrable; for example, we have an application of what we call an intelligent machine; in this scenario, we’re tracking health of a company and that can be purposed for different reasons, it could track a supplier or a customer, or inform you of the equity research process…the task there is to track the financial viability of a company, so the ontology there is replicable across multiple companies in a given industry, but each company will have its own nuance…
(21:43) What do you see as the most important role for people as we start moving towards leveraging AI for knowledge processes?
JD: That’s of course a question we’re all grappling with as technologists and as watch dogs of society; let me answer that in this context – what happens to people in processes that we automate using our AI solution and our knowledge-base automation? Our task..is to really automate manual processes, and of course there will be displacement here. and the roles that remain is that of the exception management team…in the new model it’ s checkers, and a high-end checker who has a broader view and broader set of responsibility that’s managing their processes, that’s one of the areas
…there are use cases of AI that we can clearly see where there is no displacement but augmentation of existing roles, but there certainly are others where the purpose of the automation is to automate and it will displace manual roles into higher responsibility and accountability roles, and that displacement then is an open question as to how we deal with that overall…