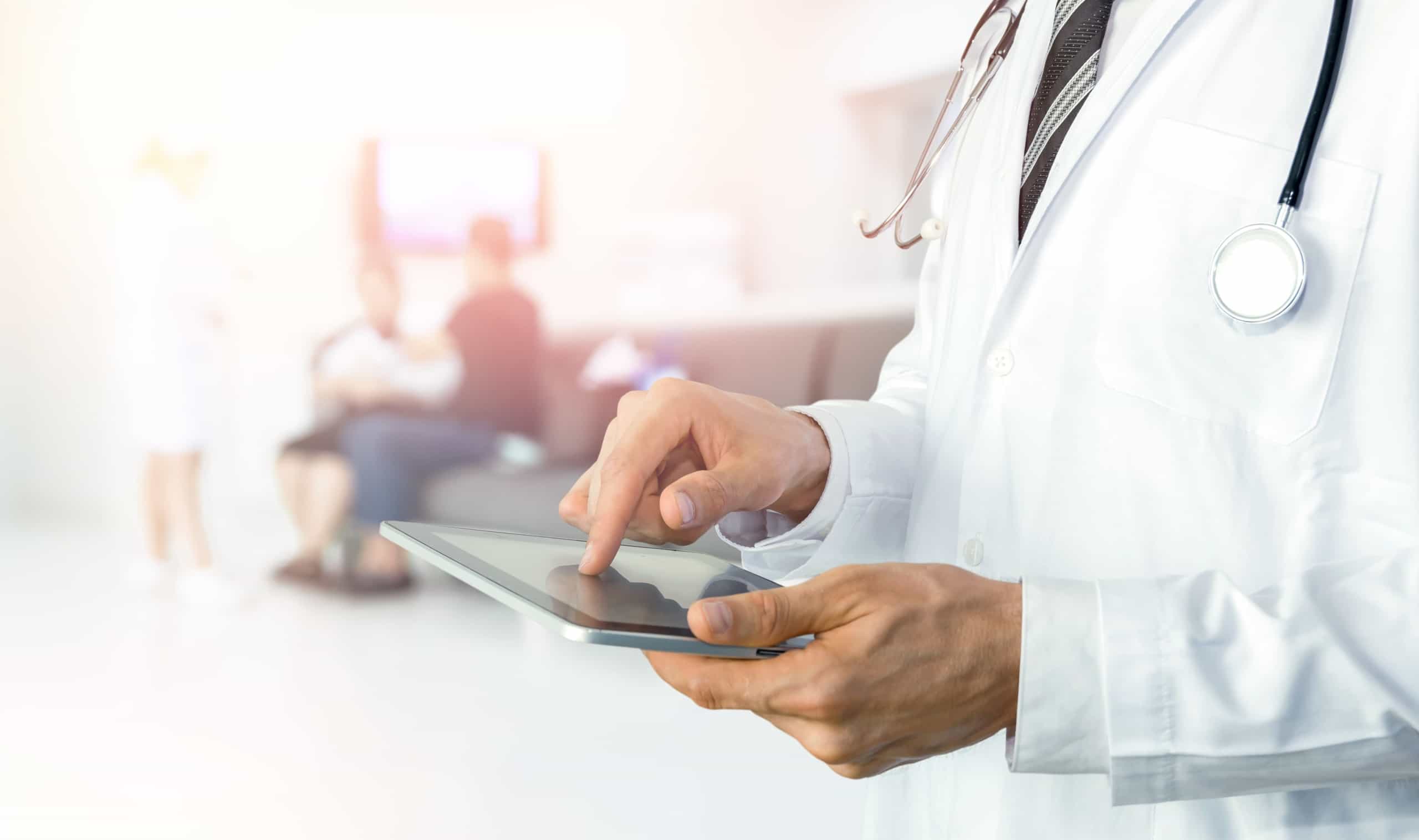
Episode summary: In this episode of “AI in Industry” podcast, we speak with Manoj Saxena, the Executive Chairman of CognitiveScale, about how AI and automation are being applied to white-collar processes in the healthcare sector.
In simple business language, Manoj summarizes key healthcare applications such as invoicing handling, bad debt reduction, claims combat, and the patient experience, and explains how AI and automation can make these processes more efficient to improve the patient experience in healthcare organizations.
Subscribe to our AI in Industry Podcast with your favorite podcast service:
Guest: Manoj Saxena, Chairman, CognitiveScale
Expertise: Artificial intelligence, cognitive computing
Brief recognition: Manoj Saxena is currently a venture capitalist focused on his role as a Founding General Partner at The Entrepreneurs’ Fund IV, a seed fund with eight active investments.
Today, he is Chairman of CognitiveScale while serving on the London Stock Exchange Tech Advisory Board, and the Saxena Family Foundation. He was the CEO and founder of two successful venture-funded software startups which were acquired by IBM (2006) and by CommerceOne (2001).
From 2011 to 2014, he led IBM Watson Software Division as its first General Manager, helping form Watson Business Group in January 2014 with a $1B investment from IBM. Prior to this, he founded, scaled, and sold two venture-backed software companies. He holds nine software patents.
Big idea
Manoj believes AI has tremendous possibilities in the application of intelligent process automation. In healthcare, he lists the following four white-collar automation use cases:
- Invoice handling and invoice exception management
- Bad debt reduction – being able to predict and manage bad debt
- Claims combat – making sure that healthcare organizations process and combat claims exception in a much more efficient way
- Patient experience – (jestingly referred to as “air traffic control”) for patients as it involves monitoring and directing their movement as they move from system to system or department to department
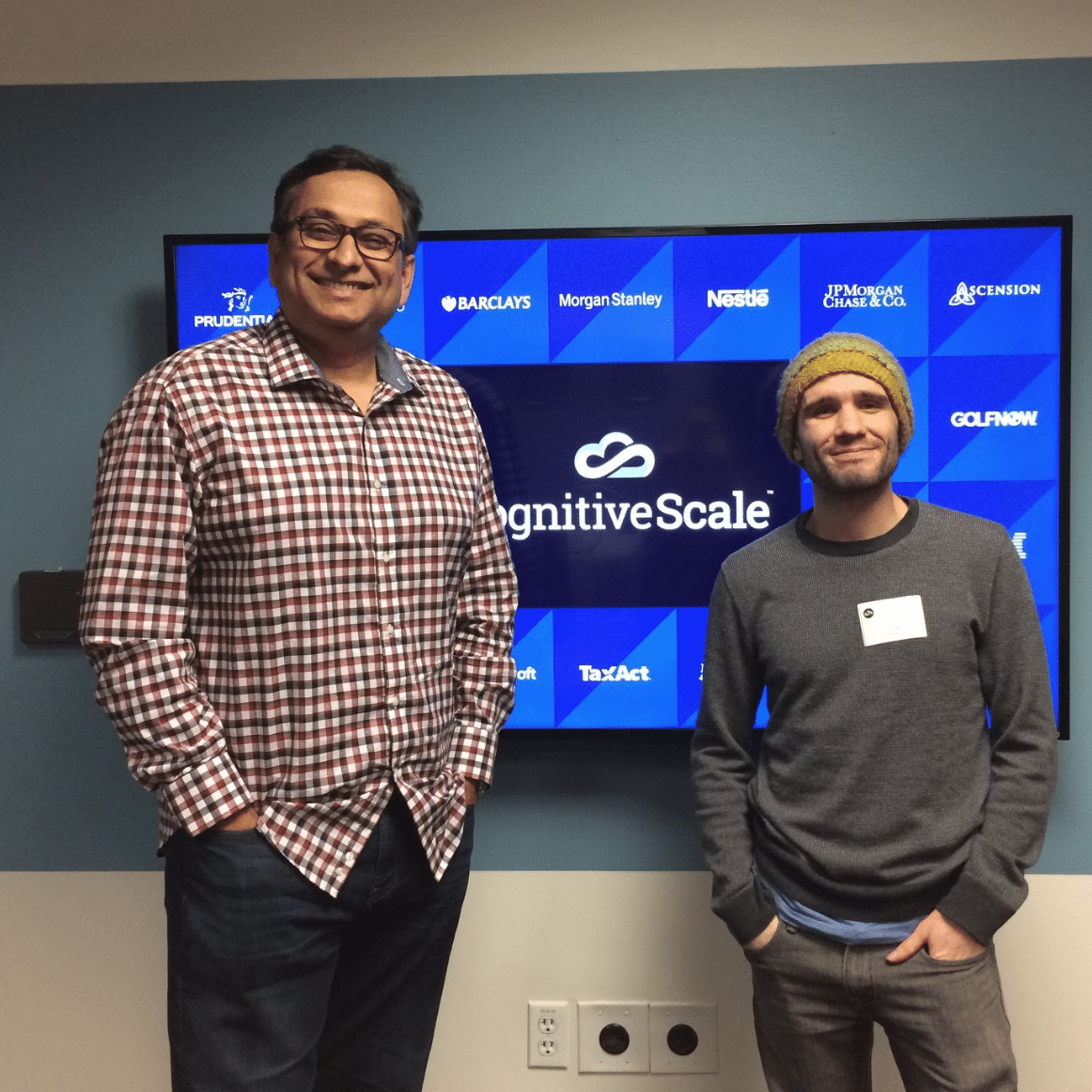
These processes require an application that can discern or make judgments based on details and historical data, making it potentially low-hanging fruit for machine learning applications.
Manoj cites the example of invoice management as part of his company’s work for clients. He relates that prior to applying AI, only about 64 percent of the invoices are processed. But within a five-month period that the process was augmented with an AI that could read and understand the image and content of an invoice, the client saw invoice processing rise to the high 90 percent.
For context, Manoj explains that invoices could be an image or a document containing multiple fields such as customer number, member ID and user ID. Depending on the company, these fields are represented in different ways. As well, individuals accomplishing the invoice could fill the fields differently, for instance using IBM Co. or IBM Corp.
Because AI is capable of learning and processing structured and unstructured data, it will be able to read and understand the key concepts within an invoice and see patterns. Applied to healthcare invoice processing, the information patterns established by the AI determines what and when the patient needs to pay.
Revenue cycle management is another area in healthcare that benefits from AI, specifically claims combat. Manoj says that in a midsize hospital, millions of rejected claims submitted by patients are resubmitted. Taking an example from one client, CognitiveScale’s AI solution took a sampling of and compared successful and unsuccessful claims. Upon learning patterns, the AI solution was able to determine the chances of a claim being denied, a step that greatly helps the knowledge worker.
AI can also be applied in the prediction and avoidance of bad debt in healthcare, according to Manoj. For instance, as a hospital is admitting or treating patients, the AI can look at data related to the patient’s capacity to pay and compare it with patients with similar profiles that the hospital has served. The AI application can then alert or guide the hospital if such patient had previously struggled with and recommend sources of funding. This helps both patients and healthcare organizations have a better experience and lower bad debt, he said.
Manoj explained that this is done by training the AI around three types of behavior:
- Declare – What has the patient declared what do we already know?
- Observe – What have we observed about similar patients in the past?
- Infer – What can we infer from cases this?
Taking the information or data gathered from asking these questions, the AI application will find patterns and come to a conclusion.
One challenge for AI companies is to ensure that the application needs to be explainable to the non-AI individuals in the healthcare organization. This is particularly vital in finance, and more so people’s lives are at stake. At CongitiveScale, we have an AI application development platform called Cortex that allows the developer to build the framework, determine the data types needed, the models to be trained, the tests to be conducted to ensure that AI performs accurately and is explainable.
This democratization of AI, Manoj explains, combines software, data and methods. He added that 10-10-10 method that CognitiveScale uses (10 hours to select the use case, 10 days to build the reference AI using the client’s data, and 10 weeks to production) has reduced deployment from eight months to a matter of weeks because the software has evolved, while AI companies are becoming better at identifying what type of data is needed to power the AI processes.
In the next five years, Manoj clearly sees AI will become standard in revenue cycle management as healthcare organizations prioritize helping people streamline in the pre-authorization, claim submissions, claim settlement and payments processes. Another area where Manoj sees the standard adaption of AI is care delivery, as organizations manage patient outcomes at the lowest possible cost while being compliant to the protocols.
Interview Highlights with Manoj Saxena from CognitiveScale
The main questions Manoj answered during our interview are listed below. Listeners can use the embedded podcast player (at the top of this post) to jump ahead to sections they might be interested in:
- (5:13): What are some of the fruitful AI applications for automating white-collar healthcare work?
- (6:08): What these have in common is work that normally involves judgment. What is a good representative process that would involve judgment work in AI?
- (7:15): For the context of people working in the back end, in terms of processing invoices, what could go wrong? What are the elements that make these complex?
- (12:10) What kind of training does the AI need to determine a patient’s ability to pay?
- (16:03) How do we take a skill and mold it into something that is easy? Can we get to the point where someone behind a desk at a healthcare organization can do this?
- (18:30) In the next half-decade, which of the AI processes you outline will become the norm? Which ones are going to see their way into an average suburban hospital?
Subscribe to our AI in Industry Podcast with your favorite podcast service:
Header image credit: Adobe Stock