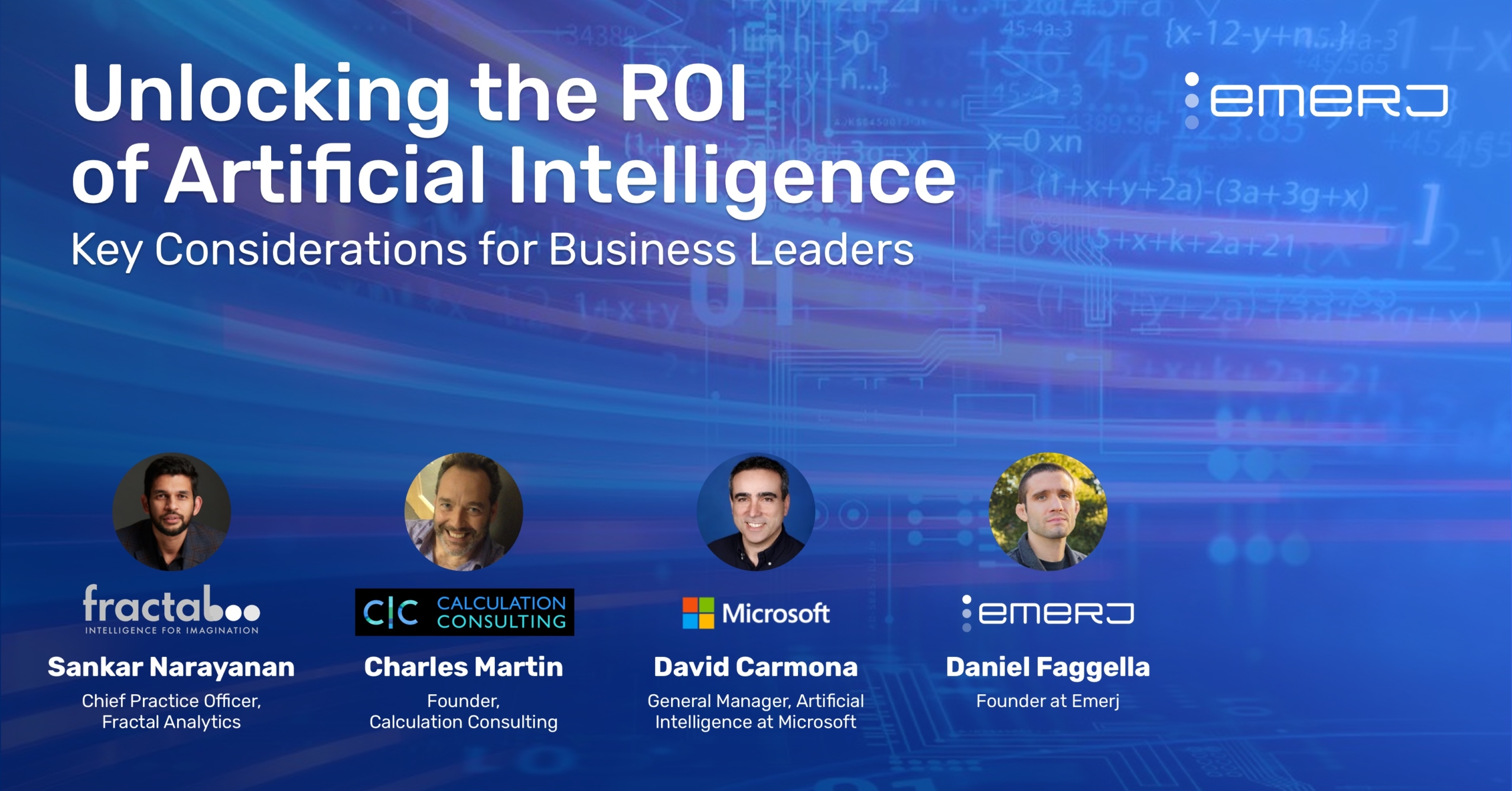
Businesses still don’t have a clear understanding of what to expect when it comes to the ROI of AI. Many believe that AI is just like any other software solution: the returns should, in theory, be immediate. But this is not the case. In addition, business leaders are often duped into thinking the path to ROI is a lot smoother than it is when it comes to AI because AI vendors tend to exaggerate the results their software generates.
In reality, identifying a metric to reliably measure the impact AI is having at a business is very hard.
In this article, we delve deeper into how business leaders should think about identifying ROI metrics that might help them understand the return they could generate from AI projects. To do this, we explore insights from interviews with three experts who were on our AI in Industry podcast this past month.
Special thanks to our three interviewees:
- Charles Martin, Founder, Calculation Consulting
- David Carmona, General Manager, Artificial Intelligence at Microsoft
- Sankar Narayanan, Chief Practice Officer, Fractal Analytics
You can listen to our full playlist of episodes in our “AI ROI” playlist from the AI in Industry podcast. This article is based in large part on all three of these interviews:
Subscribe to the AI in Industry podcast wherever you get your podcasts:
We begin our analysis with a discussion of how to measure the ROI of AI.
How to Measure AI ROI
AI projects inherently involve a level of uncertainty and experimentation before they can be deemed successful. In a small number of AI use-cases, identifying a measurable metric for projected returns may be relatively simple. For instance, in predictive maintenance applications for the manufacturing sector, businesses can link the returns directly to a reduction in maintenance costs or reduction in machinery downtimes.
But in other applications, such as improving customer experiences in banking, identifying a small number of reliable metrics to measure success is far more challenging.
Unless businesses have a clear understanding of the returns, they stand to risk losing on their AI investments. One way to ensure an AI project has a measurable metric is to choose a specific business problem where there already exists a non-AI solution and results are being measured and tracked.
Jan Kautz, VP of Learning and Perception Research at NVIDIA, who we interviewed for our previous podcast series on getting started with AI, seemed to agree that developing an AI solution for an existing business problem might be easier when it comes to measuring success rather than developing a completely new AI use-case with no precedent:
The danger of doing something completely new in AI is that you don’t actually know if what you are doing is actually correct because you have nothing to compare it to. I would suggest banks to pick an area where they already have an existing system in place so that you can compare what the results of the AI system are and know if you are at least getting better results than the existing system
Business leaders also need to understand that in order to deploy an AI project across an organization, they not only require data scientists, but also data engineers. Data scientists are those that develop machine learning algorithms for a particular capability.
Data engineers usually undertake the task of implementing the solution across the enterprise. This might involve identifying if the existing data infrastructure is set up in a sustainable way that will allow AI systems to function smoothly over time and across the organization or that the devops process is capable of sustaining AI projects.
Narayanan believes most successful AI projects that can show positive results will involve data scientists working in collaboration with data engineers. Input from these employees is critical to understanding what a measurable metric of return might because they have the deepest understanding of what the AI system can do.
But these employees usually lack the insight to connect technical benefits to the overall business gains, which needs to come from the subject-matter experts in the domain into which AI is being applied.
Business leaders need to take into account both these perspectives to truly understand what benefits they are likely to get from their AI projects today. This will also help them accurately analyze what they want these AI benefits to look like in the future and tweak their systems towards that eventuality.
Challenges to Overcome in Measuring AI ROI
Assessing ROI in Phases
According to Martin, in order to successfully realize returns from AI projects, businesses need to figure out how to test their initial assumptions, experiment with AI systems, and identify use-cases as quickly as possible.
Testing whether these initial pilot projects have been successful means measuring the performance of the AI system in the task that it is being applied to.
Measuring success in these initial projects can even go wrong in ways that are not related to the technical challenges involved with AI. For instance, if a business implements an AI customer service software and only a few users are introduced to it because of ineffective marketing campaigns, measuring the returns of the AI system become even more challenging.
This is because the AI system might have been designed perfectly, but the pilot test might not have been accurately representative of whether any returns gained will actually lead to gains when deployed across the organization.
According to Martin, it’s critical for business leaders to understand that pilot test projects must not be run at scale across the enterprise. Enacting a large project, such as completely overhauling a fraud detection system at a bank, should only be done after careful analysis of the results from several experimental pilot projects. This is in line with Andrew Ng’s advice to shoot for first AI projects with 6-12 month timeframes, not massive multi-year roll-outs.
Leaders need to think about this in phases, where the first step is to identify which small AI projects can potentially help the business gain knowledge about working with data and AI capabilities.
This doesn’t mean that the smaller AI projects don’t need to result in any success metrics. Rather, it means that in some cases, the pilot that shows the most immediate returns may not be the ideal first step for enterprise-wide adoption given a company’s goals and long-term AI strategy. Leaders should focus on AI being a long-term skillset that is attained in incremental steps.
Budgeting For AI Projects
In order to measure a specific return, businesses also need to establish what kind of budgets they need for AI projects.
Unlike simple software automation, where costs are much easier to calculate, predicting the budgetary requirements for AI projects is more complex. Martin added that this was one of the more common AI-related questions that business leaders ask him. He said:
If a business leader is looking to answer questions like how much budget an AI project might require before starting the project, the best advice I can give businesses is to first ask how much budget can they realistically allocate to AI projects and then plan around that figure. AI projects are not easy to budget for because you don’t know what’s going to work and what is not; it involves a lot of experimentation. A business might not be able to ascertain how many such experiments they might need to run before finding a valuable use-case.
Martin stresses the fact that businesses need to think about AI from a long-term strategic perspective. They will have to make a decision on whether they are an “AI company” or not. Being an AI company means there will be a period of constant experimentation with uncertain results that could sometimes even take 6 months of experimentation to yield any noticeable results.
A recent article on MIT Sloan Business Review states that new ways of working and new management strategies (what might be called “change management”) are among the largest factors keeping most AI initiatives from generating ROI. Our own research arrives at the same conclusion.
There’s also no guarantee that an AI project will not go above budget, given the aforementioned uncertainty and experimentation involved. But this will give the data science team leaders an idea of how many experiments they might be able to conduct realistically and which ones they might need to prioritize.
Developing a Culture of Innovation
One big challenge that many businesses might be grappling with when it comes to AI likely lies in ensuring that every dollar that goes into AI projects sees a significant return. Getting a return as soon as possible is the ideal business scenario.
Narayanan spoke about what misconception business leaders might have about measuring returns from AI :
Most of our knowledge around what AI can do for business stems from well-marketed examples in the news media. We find that most of these use-cases have been business problems that are well defined in nature. For example, we have seen reports of AI software beating the best human chess players or Beating humans in Alpha Go – These are problems that have definitive end points. But when it comes to the most common business problems in fortune 500 companies. These do not have definite outcome.
What Narayanan seems to be articulating is that businesses might ask questions such as, “Is our next product launch going to succeed?” These questions are significantly more open-ended than a board game with definitive results. The term success might mean different things to different people or teams within an organization.
It might be hard to frame a clear question with a definitive answer for business problems. These questions at best might indicate that their problem statements can be extremely hazy and complex.
It might be impossible for any firm to look at a bucket of data and report how much business value might be gained from leveraging that data given the right kind of algorithms. This might be hard to digest for business leaders, but they need to expect uncertainty when it comes to AI.
This is not a traditional business mindset in many industries. According to Narayanan:
This is a cultural shift in the way of thinking of about how data might be critical for AI success. Leaders need to think about how AI can solve a business problem at scale for the enterprise, that is aligned with their business objectives as a whole while being highly sustainable.
Framework for Thinking Through AI ROI
In this section, we put forth a list of frameworks that business leaders can follow in order to maximize the possibility of gaining positive returns from their AI projects and effectively measuring it as such.
Traditionally in business, the term “ROI” usually corresponds to short-term financial gain, often in terms of improved revenue. AI is a broad technology and sticking to this traditional method of defining ROI might not be the best place to start for businesses. For instance, AI might very well be used to potentially increase revenue in an application.
However, AI can also be used to reduce costs, improve customer experience, or increase the productivity of a specific team within the business. The first step to understanding AI ROI might be to associate the returns with any types of positive business outcome, not necessarily financial gains, including leveling up a team’s AI-related skillset.
Carmona said that in his experience, there have been several instances in which businesses have needed to invest funds in an AI project as it is being built due to budgetary constraints.
At the same time, business leaders might be looking for immediate returns on their AI investments. According to Carmona, balancing these two factors (uncertainty in AI projects and gaining returns fast) is something business leaders have to figure out before starting AI projects of any kind.
He spoke about a particular framework used by Microsoft (called the Agile AI framework) to find a balance between the two. We detail the steps involved in this framework below with insights from the interview:
- First, business leaders need to fully understand the capabilities of AI and what it can do in business and even more importantly what it cannot do
- Business leaders should then identify and position all the potential AI opportunities they see in their business. At this stage, business leaders should only focus on what areas of their business AI can be applied to. It may turn out that some of these applications are more suited to the existing data infrastructure in the organization and don’t require AI.
- The next step is to understand the size of the opportunity from each of these potential AI opportunities. Businesses need to break each opportunity into smaller pilot and test projects in which the outcomes for these initial projects are always listed in great detail, such as improving the call center operational efficiency or time spent in generating compliance reports.
- The last step is to align the outcomes of the smaller tactical projects on one side with the longer-term strategic AI capabilities that the organization wants to acquire
Narayanan stated that one of the critical things for business leaders to understand about measuring the returns of AI projects is to first frame the business question that AI is being applied to in a way that is specific.
For instance, leaving aside the technical concerns, businesses first need to ask questions such as “Is AI being used to solve a problem in the rate of growth of the organization, or is it being used to improve the efficiency of a business process or to improve customer experiences?”
He went on to give an example of a firm that he claimed worked with Fractal Analytics in the past to explain this concept better:
About 18 months before getting into AI projects the client we were working with brought in a visionary leader who said im not supporting any initiative that can’t show progress in 6 weeks.. He seemed to enforce this constraint even though he understood that there are a number of initiatives that are transformative long term engagements at enterprise level. This allowed the company to be more rapid defining areas to work and ruthless about what success and progress means and therefore establish a codified approach to measurement.
In a 12 month period they executed 30-40 different initiatives that they called Minimum Viable Proposition (MVPs). They identified 5-6 which had the potential to become transformative at enterprise level and this year they are taking these to deploy on an organizational scale.
According to Narayanan, the client gleaned the following three insights from this process:
- The team realized very quickly that multi-disciplinary teams are required for success is any of the smaller AI initiatives.
- They also realized that this more agile mode of working lead to better results and more clearly measurable success compared to the traditional waterfall model of working in software environments.
- The last and most important was that documenting and codifying the learnings from each of the MVPs helped the team to successfully ensure that the probability of success in the next pilot project was slightly higher
Related Articles
- Predicting the ROI of AI – Pitfalls to AI Adoption in the Enterprise: An extended analysis of how to project the ROI of an AI project and what business leaders need to consider to do this effectively. This article expounds upon some of the key points mentioned in the one you’re reading right now.
- The Financial ROI of AI Hardware – Top-Line and Bottom-Line Impact: This interview with Mike Henry, CEO of Mythic AI, explores the ROI that can be generated from upgrading a businesses’ computer hardware to better run AI algorithms.
- How to Apply Machine Learning to Business Problems: An overview of what business leaders should think through in order to determine if AI is right for the business problem they want to solve or not.