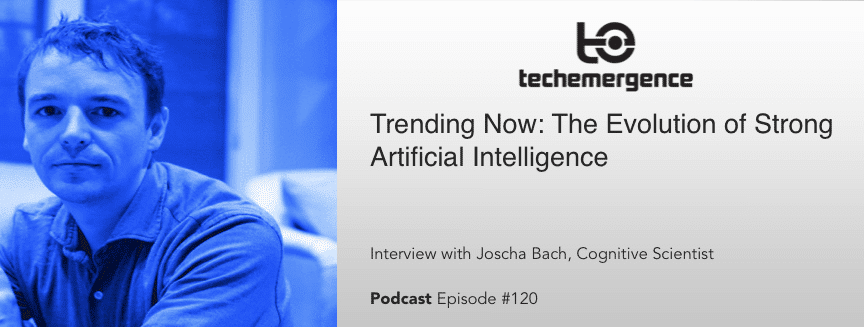
Episode Summary: Dr. Joscha Bach is a software developer and researcher, who is currently developing a cognitive AI framework at MIT Media Lab and the Harvard Program for Evolutionary Dynamics. In this episode, he speaks about the troubles in projecting when strong AI may be developed, and sheds light on the trends taking us there, including deep and reinforcement learning.
Guest: Joscha Bach
Expertise: Cognitive Science and Artificial Intelligence
Recognition in Brief: Joscha is a lead researcher on the MicroPsi project, agent architecture that is helping scientists to better understand how the mind works at the level of consciousness. His book, Principles of Synthetic Intelligence, published by Oxford University Press in 2009, discusses the foundational ideas for MicroPsi. In addition to AI research, Joscha has taught AI and Programming at the university level, and was also the founder of the eBook company Bookpac GbR, as well as co-founder of IT services company Wizpac Ltd.
Current Affiliations: Research Scientist at MIT Media Lab/Harvard Program for Evolutionary Dynamics
Strong AI Grows in Popularity
Strong artificial intelligence is yet another trending term in today’s AI-covered media. What is it and how far have we really come in achieving the concept? Strong AI, in its most basic sense, encompasses a mindset of artificial intelligence development. Strong AI proponents’ ultimate goal is to develop AI cognitive capabilities to the point of an even playing field with the intellect of a human being. According to Dr. Joscha Bach, the areas in which we’ve made the most progress are largely due to developments in the hardware.
“We obviously have technology that was unthinkable five years ago, systems that are close to approaching human proponents in some vision and other tasks like automatic categorization”, says Bach. In comparison, we’ve made small progress in terms of understanding the overall architecture of mind. The ideas in deep learning are those that people already had back in the 90s, and were developing back in the 70s, says Joscha. Those ideas couldn’t be implemented because the hardware didn’t exist at the time.
In other words, we’ve made greater leaps over the past decade due to better technology in capacity, not because we have better theories; however, “one of the big differences is in our conception of AI”, says Bach. “I was almost on the way out (of AI)…this has changed.” AI works well now, and people have gotten wealthy in the last few years building AI technology. According to Joscha, there has been more funding made available to AI researchers in the last five years, particularly by private companies like Google, than in the entire history of AI. This major boost in investment is helping AI on its upward trajectory.
Deep Learning Meets Reinforcement Learning
This trend speaks volumes for the importance of cultural shifts and changes. Bach also recognizes that certain technologies seem to have limitations before they can accomplish full potential. For example, deep learning only has so much power in recreating the neural network layers of the human brain; it’s rather limited, actually, in comparison to the real thing. But reinforcement learning may speed up in the next few years, says Bach.
“In some sense, we can view our mind as bands of regularities implemented in our blueprints in the genome, and only a small subset of the genome encodes for our nervous systems, which for perspective fits on a CD ROM. The complexity of getting the mind to work once we have the known principles is not so big, but the reverse engineering is what is so difficult,” explains Josacha. With deep learning, we may have captured only a dozen of these ?, and it’s very unclear as to when we’ll make more progress in this area.
“It’s very tempting to say it will be done in 20 or 50 years…but as a software developer I know its a pipe dream – if I don’t have a specification, I don’t know when it will be done,” suggests Bach. He contends that there could well be a silver bullet of unifying principles that are yet to be discovered, which will make the process of building a human-like mind much more possible.
Certain deep learning and reinforcement learning have overlaps. But while deep learning is mainly about building neural networks with many layers, Bach defines reinforcement learning as “related to taking in signals from the environment and using it to build the “right” kinds of representation, to anticipate behavior or the world, and coordinate actions to come up with the right policy to maximize the signal.”
In much the same way, humans receive signals constantly, rapidly forming neural networks and learning pleasure from pain, for example. We’re also taking in these signals in many dimensions, time, space, color, etc., and organizing these into features, object permanence, mental states of people, and coming up with explanations of concepts.
“One of the big questions”, says Joscha, “is how much do we have to put into the machine in the first place?” Furthermore, how is this input related to how much is hardwired in our brains? The key to unlocking cognitive science lies in understanding just what “mind” is, and how to engineer and get it to work through our own creating.