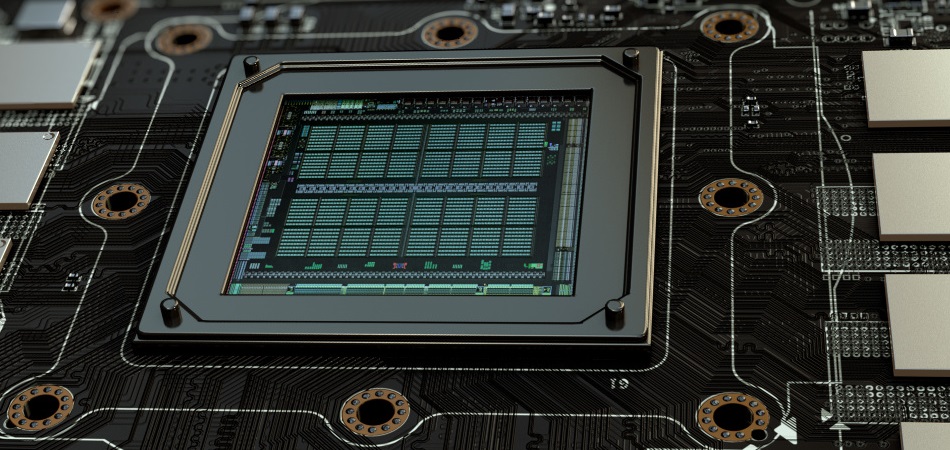
Large enterprises are eager to use artificial intelligence software, but many of them aren’t aware of the hardware required to execute many AI capabilities. To get a better idea of these hardware considerations, Emerj spoke with Victoria Rege, Director of Alliances & Strategic Partnerships and Graphcore, for Kisaco Research’s AI Hardware Summit in Europe, which takes place October 29 – 30 in Munich, Germany.
We discussed the current challenges enterprises are facing in adopting and using AI hardware. We then projected where AI hardware may find a home in business in the new decade.
Listen to the full interview from our AI in Industry below:
Subscribe to our AI in Industry Podcast with your favorite podcast service:
Guest: Victoria Rege, Director of Alliances & Strategic Partnerships – Graphcore
Expertise: Technology marketing and go-to-market strategies
Brief Recognition: Rege is also an Operating Partner at Air Street Capital, and she served for 9 years at NVIDIA as Head of Global Marketing.
Interview Highlights
(2:00) How is newer AI hardware making its way into enterprise today?
Victoria Rege: New AI hardware is making its way an enterprise today from various different routes. I think we have seen a lot of interest for people both using physical hardware in their data centers, as well as accessing hardware from the cloud. But we’re really just at the very beginning and I don’t even know if we’ve reached the tipping point yet of using hardware for machine learning and artificial intelligence.
(02:30) What do you consider to be the tipping point?
VR: So I would say most companies know they need to use it. Obviously, data scientist is one of the most popular job functions in this space. But really understanding how to use the hardware and how to get that to get better insights for inference and training is, really, we’re only just getting started.
GPUs definitely have traction. I think it’s that the explosion of data and the explosion of compute and cloud computing that’s happened over the last several years has just led to a hunger for even more computing power and different computing power that solves problems that not only people are challenged with today, but what machine learning researchers and experts in the AI space see as the challenges that are five years from now, 10 years from now.
So we built a processor called IPU, intelligence processing unit, and we built it from scratch from the ground up to support the future models.
Today, we train models with incredible amounts of that data and we infer from the model. But that’s really just the beginning because today’s models struggle with context and the ability to learn from experience.
Today’s hardware is kind of holding people back from achieving the next breakthrough. So training is taking far too long than people would like, training a dataset. Models can’t really be deployed in real-time, and that’s why you’re seeing so many different companies and why we have different conferences just focused on AI hardware and new types of hardware.
(05:30) it sounds like the challenge is people kind of have their existing workflows with GPUs. Is that safe to say?
VR: I think it really just depends. We’ve gotten a lot of feedback from leaders in the AI space on both what’s holding them back, what they would do if they had a different type of processor.
We also spent a lot of time building our poplar software roadmap at the same time as we built the chip to provide less of a friction point for people who are using GPUs or CPUs or FPGAs today.
It’s really straight forward, with IPUs able to plug into the existing machine learning frameworks that people are using today, like TensorFlow and PyTorch and Onyx. Those frameworks have only been around for a few years. OpenAI recently announced that they’re planning to use PyTorch going forward. When we first started Graphcore, none of those machine learning frameworks existed.
So building an architecture and a software stack that’s flexible and allows people to make big breakthroughs is what we’re focused on. Once we have a chance to share with AI researchers and practitioners why our architecture is different and how easy it is to use with our software stack, many people are jumping at the chance to use us. Back at the end of 2019, Microsoft announced that IPUs were available in Azure in preview.
So that’s fantastic. We have lots of different industries and different types of people who are really focused on solving challenges. If it solves their pain point or their issue or it allows them to do something faster with better efficiency, switching hardware is not a big deal. In fact, some people who are data scientists don’t even care what the underlying hardware is necessarily, as long as they’re getting the results.
(09:30) What are the differences between inference and training when it comes to AI?
VR: Training is essentially referring to the process of creating a machine learning algorithm. It involves the use of a framework, like PyTorch or TensorFlow, and a training dataset. Data scientists, engineers, can have a dataset and a model that they use to train for a variety of use cases.
Inference actually refers to the process of using a trained machine learning algorithm to make a prediction. Inference is when the computer is learning from the data that it trained on.
One of the good examples of that is in the autonomous driving space. Many of the autonomous driving companies have to train all of their datasets to come up with all the possible scenarios of what could happen if it’s raining, if it’s snowing, if it’s a hill, if there’s traffic, identifying whether there’s a leaf blowing across the street versus a child crossing the street and doing all of that in real-time.
I mentioned earlier, people are frustrated that training is taking so long and that models can’t be deployed yet in real-time. So that’s why we have different types of hardware. Some of the other simple use cases as well is, if you work with colleagues in different countries, they could talk in their language and you could talk in your language and in real-time, you can hear each other, but in the language that you understand.
So being able to deploy the data in real-time. But in order to do inference, you have to do the training part first. Today, most people use different types of hardware and then they have to transfer the data from one kind of hardware to another.
The IPU was developed to be able to support both. You can do inference or training, which makes it a lot easier in terms of saving you time, just even from transferring the learnings from the training over to the inference.
(14:00) What does it look like to move data from a training environment to an inference environment?
VR: It really depends on the specific use case, but I think if we take the example of autonomous vehicles, the training happens in a data center with racks and racks of hardware. What they want to do is be able to infer in the car.
Obviously, you can’t put a data center in a car necessarily or that wouldn’t be practical, but I think that’s one of the things that autonomous driving companies are focusing on. How can they achieve full autonomy if they’ve got the data in two different places?
I know there are a few different speakers at this upcoming conference who are focused on embedded. It’s just really the case of bringing the training results closer to the computer’s ability to infer.
(15:30) What does the future of AI hardware look like?
VR: Some of the areas where it’s being used today and has a lot of potential are in the financial sector, so hedge funds and quants and people who are trying to make better decisions about their financial forecasting or analyzing things over a series of time.
There’s something called, Markov chain Monte Carlo, and that’s a probabilistic model that’s really suited to time series analysis problems. We’ve seen customers be able to use that to train their models in four or five minutes instead of two hours.
The other space we’re seeing a big shift in is healthcare. How can doctors identify problems sooner, whether it’s a hemorrhage or cancer or something where if the models could infer based on images or data and give a faster diagnosis and also a more precise diagnosis. That benefits everyone. It benefits the patients.
At a medical conference at the end of last year, there was a Kaggle competition where folks were provided 600,000 image slices of brain hemorrhages and all the data was augmented, and they were able to run it on a model and find out that, using this new hardware, the IPU, they were able to run the model two times faster than existing hardware today and have four times more efficiency.
So if you think about just helping doctors identify problems sooner, or even if computers can infer what’s going to happen to a patient, so they can say, “You’re on track to have some type of health incident, but here are the things that you can change today to prevent that from happening,” I think that’s one of the areas I’m most excited about because that’s something that benefits everyone.
Subscribe to our AI in Industry Podcast with your favorite podcast service:
This article was sponsored by Kisaco Research and was written, edited and published in alignment with our transparent Emerj sponsored content guidelines. Learn more about reaching our AI-focused executive audience on our Emerj advertising page.
Header Image Credit: Carleton University