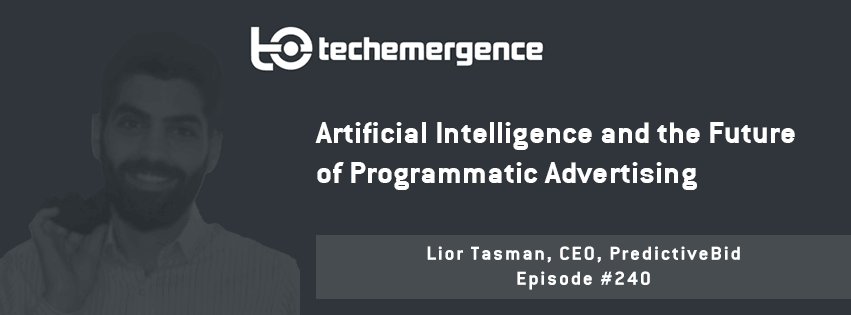
Episode summary: In this episode we talk to Lior Tasman who is the CEO of PredictiveBid, an Israeli-based predictive advertising optimization start-up. The team focuses on applying AI to some of the bigger issues in programmatic advertising to help draw out more ROI from ads. We discuss some of the challenges of programmatic advertising and what the future of programmatic advertising may look like from an advertiser’s perspective.
A huge percentage of digital advertising dollars today go to Google and Facebook, who dominate that sector – and are inevitably central for the future of programmatic advertising. There’s a lot of evidence to suggest that the growth in digital advertising in the last two to three years has gone almost entirely into their coffers. At least for the foreseeable future, Facebook and Google will retain the ability to dominate that space.
The ability to be able to bid for the attention of particular target audiences, whether they’re searching for a specific term, live in a specific place or they like a specific sports team, is something that doesn’t seem to be going away, and seems to be rather efficient, thanks in the large part to Artificial Intelligence.
Guest: Lior Tasman, CEO, PredictiveBid
Expertise: Data and advanced machine learning algorithms, growth hacking, complex tracking methods.
Brief recognition: Lior Tasman is the CEO of PredictiveBid Predictive Bidding Platform. Tasman has spent 10 years in innovative startups, working with some of the biggest companies in advertising. He has a B.Sc. in mathematics and philosophy from Tel Aviv University.
Big Idea:
Just as a huge volume of stock market transactions are handled by AI today, the farther future of programmatic advertising will likely involve competing AI systems, altering bids and ads in real time (overseen by humans, but acting autonomously to optimize for a goal).
Programmatic advertising already involves a good deal of AI, but that doesn’t mean there isn’t room for improvement from the perspective of the advertiser. While Google may be able to use AI, can advertisers use AI to optimize the results they are getting from Google in the first place? Moving forward to the future, the answer is increasingly likely to be yes. Advancements in marketing, particularly, getting more ROI from marketing effort is synonymous with getting more granular with targeting the right prospects at the right time, and that task requires more data and better use of that information.
(Readers who work in the marketing domain may be interested in our full “guide” article on the applications of artificial intelligence for marketing and advertising.)
Insight to Action: Our interview with Lior teased out a number of insights for overcoming the challenges of AI in advertising, and understanding it’s potential benefits.
Overcoming the Challenges of AI in Advertising
- Timing: It can be difficult to track cohorts and groups over time, particularly if the sales down not take place until 30, 60 or 90 days down the line. It’s important to have a longer-term customer lifetime value within a marketing campaign, one that allows you to gain a wider perspective on these groups
- Tracking Data: Marketers need to be able to use the end of the funnel data to optimize the experience of potential clients as they move along their journey to a sale. To do this you can use important data like, how many people left items in their shopping cart, which customer respond during which days of the week, or hours of the day?
- Data Entry: Each company has its own needs and sets of points that it wants to track. One of the problems with tying in all this information is that it comes from many different sources, including data from outside third party sources, such as calendar: days of the week, holiday, holy events and how they may affect the potential conversion rates. The more data a company has, theoretically the more power they have to target their advertising, but it’s incredibly difficult to crunch all this data.
The Promises of AI in Programmatic Advertising
In the near term, AI could help eCommerce companies predict and understand the value of each customer and each deal and also to segment complex data into groups such as high, middle and lower performing groups in order to make complex calculations based on this data. This would allow marketers to target advertising more specifically based on the time of day customers prefer to shop, calendar events, long-term conversions etc. For example, marketers could break visitor traffic down in to one 24-hour period to see where visitors were coming from, what time they made their purchase and how much they were spending.
Will this type of AI eventually replace marketers? The answer is no. Though the machines can certainly make programmatic advertising more efficient, faster and easier to implement and AI can help break down data to a much more granular level to optimize targeted advertising, there remain many factors which need human input and interaction.
For example, companies must be able to set their own rules such as maximum budget, which products to offer next season. Once the appropriate guidelines have been set, business owners can let the machines crunch the data and help with decision making so they can focus on the growth of their company.
Interview Highlights on the Future of Programmatic Advertising
The following is a condensed version of the full audio interview, which is available in the above links on Emerj’s SoundCloud and iTunes stations
(3.08): What are your ideas about some of the limitations and problems in the programmatic advertising world?
Lior Tasman: Looking at the main acquisition channels, I would day that the two main problems today are using all the available funnel data, and then crunching it with the right algorithms based on specific data needs then matching that data to the market’s needs. So, improving results on all available data is a hard challenge to tackle.
(4.15): What are some examples of that data that is hard to factor into a business case?
Lior Tasman: Anything that really happens in the customer journey after he is performing the first conversion event on the website, whether it’s a lead and someone putting in detail of in eCommerce and someone adding stuff to his cart. Measuring what happens later in the funnel, such as a closed deal with a sales team or even a sale that’s happening thirty days after the user arrives at the website, this is really important data that is really hard to tie back to your marketing efforts today. It’s also difficult to predict how the traffic that’s coming to the website will perform at the end of the funnel.
(7.11): What are the challenges of pulling all this data together?
Lior Tasman: The problem is even more complex because you really need to be able to make decision sin real time based on all the available historical data…You really need to make a prediction of how likely is someone who is coming to your website on Monday morning is likely to convert versus someone else coming in later that day…Tying in the data is really complex because it involves using a number of different data sources.
(17.48) Where does AI fit the bill in terms of softening some of these bigger problems?
Lior Tasman: AI is solving the problem of crunching big sets of data and then making a lot of relatively fast, complex calculations…it is also a system that improves itself over time based on its predictions. Building a rule-based algorithm is relatively easy, but when you want to have a machine that really reacts to specific events in the market, that’s better at predicting how likely the market is to behave, and you need to make that decision really fast, you really need a system that is more. AI comes in in making those calculations and then helping and automating manual or really hard to compute problems where the market is also changing over time.
(24.19): The set-up has got to be tough, but after that what is the interaction between man and machine?
Lior Tasman: Basically the AI is making the team more efficient. I don’t see AI replacing teams. There are so many factors that needed to be guided by humans. The machine is only there to make them ore efficient and to make the decision making process easier or faster.
(30.04) What are the major categories of data that you have to pull together?
Lior Tasman: Top of the line data with bottom of the funnel data…It sounds complex but you have to tie in your marketing data either with your data banks or with your CRM. You make the connection from whenever the conversion happens and from that point onwards, you’re really able to track things…Then third party data is usually the last part in the optimization, unless it’s a very specific situation or major event that’s going to affect the market.
(For insights on broader applications, see our full article on AI applications in marketing and advertising.)