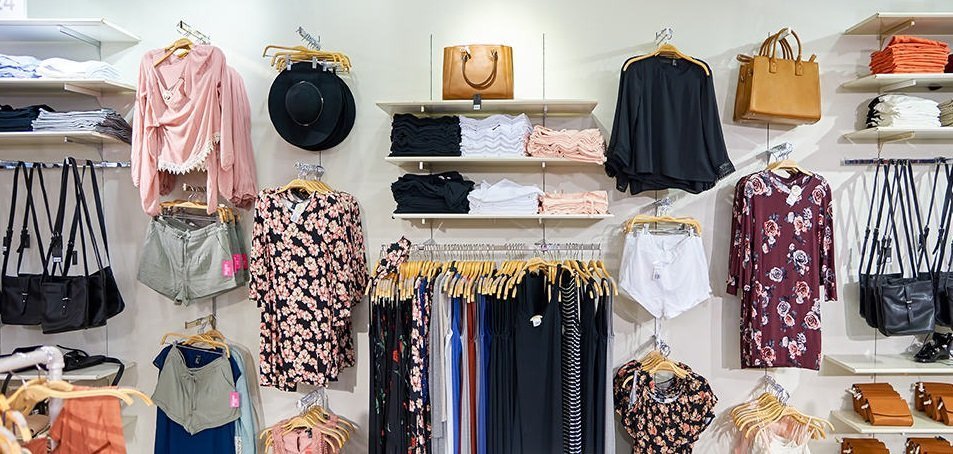
Episode Summary: This week we talk to Alejandro Giacometti, the data science lead at a company called EDITED, based in London. The company claims to help retailers with inventory optimization, and we speak with Giacometti about how artificial intelligence can be used to search the web for the product clusters and individual products of major retailers to help inform other retailers on which products might be popular.
There are two primary takeaways from this episode. The first is the broad capability of monitoring the competition with artificial intelligence, something that can be applied across industries, not just in retail. The second is that EDITED is generating information from what is freely available on the web, and so it would seem their software doesn’t require businesses to integrate it into inventory management systems in order to train the algorithm behind it.
I’m not necessarily lauding the company; I haven’t used their product nor read all of their case studies. That said, it’s worth noting simply because its approach is fundamentally different than most AI vendors.
Subscribe to our AI in Industry Podcast with your favorite podcast service:
Guest: Alejandro Giacometti, Data Science Lead – EDITED
Expertise: data science, machine learning, image analysis
Brief Recognition: Giacometti holds a PhD in Image Analysis from UCL. Previously, Giacometti was a senior data scientist at DigitasLBi.
Interview Highlights
(03:30) How did [inventory stocking] used to get done and sort of where is AI playing a role in chipping away and making that a bit easier?
AG: So most of our customers are buyers and merchandisers and daily fashion retailers, and the process that they have to do is they have analysts…walk into their competitors’ stores and with a notebook for looking at specific products. For example, looking at what is new in outerwear in this season. So they would keep a list of every jacket that is in the stock, what’s the price, what’s the material, what colors, what kind of graphics, and they would tally tell all this sort of information manually every week or every couple of weeks. And then they would go back to these large meetings where they would compare and contrast what sort of trends are happening in shops in malls, in shops in high streets.
Often they would also have a digital team that goes to competitors websites and gathers all this information in an extremely manual way, and so one of the main ways that we can help these people when they log into EDITED, immediately they have access to hundreds of millions of folks where they can search for market, for region, they can list them as their color or even patterns, and they can pinpoint exactly where their competitors are and have a broad view of exactly what’s happening in the market on a real-time basis.
(5:30) So it sounds like a very sophisticated kind of functionality for scraping that you can use to train algorithms on what kind of style is it, what kind of colors is it, what kind of designer is it.
AG: Yeah, that’s absolutely right. We have this pretty complex system of crawlers that go out to about more than a few 1000 retailers all over the world, where they gather every single product that’s available on their website every single day. And so that gets all of their information instead of providing in a way that retailers have decided to say. So some retailers have their own hierarchies, they might for example focus only in underwear and so they might have like a very detailed hierarchies kind of way which they put their products in. And so crawlers just go to the websites, go product by product, get an average of every SKU, every image, every product description, and then we gather those in sort of like a central location, and there’s where we have some pretty sophisticated artificial intelligence, which buttons throughout the whole market of data.
And we have our own hierarchy of classification that we’ve hired alongside experts in the domain in fashion where we have decided how to precisely sort every single product that comes into our system and not just categorize them like our traditional hierarchies. For example, you can say footwear, and then something that is a category of footwear would be a boot or a shoe or something. And so we have very sort of precise hierarchies.
But then we also have some pretty advanced conclusions in neural networks that look at the images of the specific boot, for example, and determine whether it has a heel or it doesn’t have a heel. So when a buyer is actually looking to compare what to find, what products their assortment is going to compete to, they have some very fine-grained choices that they can select a section of the market and then they can do interesting things like looking at the variation week on week or a month on month or looking at the same season, the last year or variations in colors or whether a particular pattern is coming out or is trending this year or something.
We find that products follow different patterns, and this is something that maybe wouldn’t have been apparent a few years ago without EDITED, and that there are some products that are really, really successful in that they can be in the market for years and they never really go out of stock in the sense that they come back season after season. Maybe a back to school strategy from a specific retailer. On the other hand, there are some products that are just trends or maybe something that they’re just trying out. For example, yellow dresses, maybe last summer you would’ve seen a few retailers trying out a couple of yellow dresses and you’d seen them for maybe a few days or a few weeks or sometime, but most likely they were in the market for just a short amount of time maybe with a stable pricing.
But then exactly one season later, you would see an explosion on the same sort of yellow dresses and what do you would’ve been able to pinpoint with EDITED is these sort of smaller sort of tests that retailers are making maybe one season prior to the one that in which a product that’s going to be extremely successful. But on the other hand, a yellow dress after it has had a wave for the next season and maybe it’s not going to be such a big thing. Right? So if you see that a massive volume of yellow dresses appeared less season, this is where the experts in the industry and where we’re not trying to replace them [inaudible 00:11:17] they’re always going to have that sort of know-how, whether they’re gonna make the decision on whether something is likely to come back or something that is now here to stay.
(12:30) The process of bucketing for you folks is sort of where AI can get that done at scale because obviously if you’re scraping thousands of retailers on a daily basis, it’s very computationally expensive to do it with machines, but it’s way easier than having to do it with humans. So it sounds like it’s really that bucketing that machines can kind of proxy based on, I would imagine a lot of labels they can proxy. Where does this item go? Where does this item go, where does this item them go?
AG: Yeah. And I mean, traditional analysts that had to do the same thing, would have had to go to a store and decided which one of the products that they’re seeing on the shop prior competing with the products that we are planning on putting in this next season. And in fact we’ve recently run a study where we compare the performance of our machines, of our algorithms against the expense in the industry and we found that our algorithms were doing a better job more consistently than experts.
(14:30) What does that future look like to you?
AG: I think in a sense the data that we’ve been collecting for the past 10, 20 years has recently empowered a host of algorithims and tools and ideas about where’s going to take us in sort of the retail space and I think the power of this information that we’ve gathered so far, it can only get better if we become better at using it basically. And I think the main space that I can see is there’s a lot of different kinds of ways in which the products do well in the market. There’s no sort of single measure for success. And so if basically machines can help us identify the different ways in which the products can be successful in the market that can help us make better decisions about what products to stock and where to sell them.
That is significantly different to a product that is trend-based or different than a product that you can have because maybe a trend-based product is high margin for a second, but you really need to figure out how many of them you need to stock, for example. Those are decisions that traditionally have been made by people with generally a feel for the industry and good taste, but the power of data is basically to give us some evidence to take those decisions without replacing the experts in the industry. I think the power of the intuition is never going to be replaced by machines, but it’s going to be enhanced.
Subscribe to our AI in Industry Podcast with your favorite podcast service:
Header Image Credit: Adobe Images