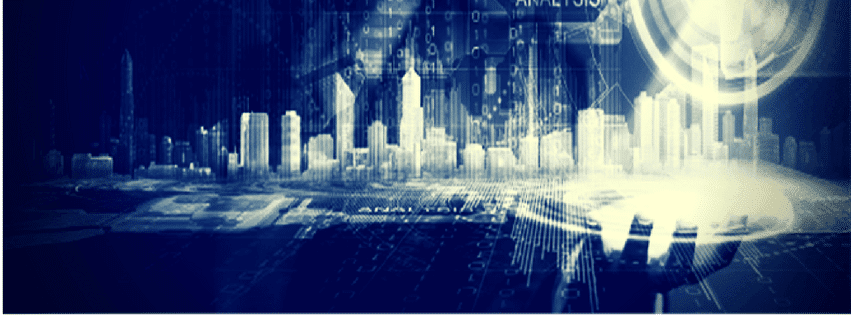
Unlike other components to an enterprises’ technology mix, determining the ROI of machine learning is a less-than-obvious process, particularly when solutions are new and little by way of case studies or benchmarks exist.
While we’re far from a world where SMBs (small- and mid-sized businesses) outside of Silicon Valley integrate AI into their regular operations, we will undoubtedly see an explosion of novel uses in industry and enterprise over the next 5 to 10 years, and executives are rightly concerned with how to make the most of those technology, time, and staffing decisions. If you’re a business who’s new to the machine learning scene (and that’s a vast majority), there are more burning questions than answers at present.
This is exactly why Emerj set out to ask the question:
“What are the criterion needed for a company to derive maximal value from the application of machine learning in a business problem?”
Tapping into our hundreds of interviews (on our podcast and otherwise), as well as reaching out to other experts in the field, allowed us to glean valuable insight from researchers and executives across the globe. We reached out to 31 experts and asked them to share their thoughts on this trending topic. Thirty of those participants responded to this particular consensus question.
You can see an abbreviated version of the answers to our “Deriving Value from Machine Learning in Business” question below in our full infographic.
Determining the ROI of Machine Learning – Expert Consensus:

Note: The data for this graphic is no longer available publicly and are only available for Emerj research members. This change was enacted in November 2018.
The three major categories of responses were almost even across the board, with “sufficient data” taking a slight lead, and there was significant overlap of more than one category applying to some responses.
Having enough existing data seems to be a leading concern for most ML practitioners. As Dr. Reza Zadeh of Stanford commented, “datasets are the differentiator” for businesses. Some tout the important of using unstructured data, and others clarify the importance of using noisy data in large amounts (or, if your data set is limited, make sure it’s clean). This idea is also paired with the (a) need to automate training of ML systems and develop ways to more seamlessly integrate data with business processes (Harvard Business Review published a solid overview of real-world use cases) and (b) having sufficient time to train and make sense of a data set.
Building an in-house team of data science talent also seems poised to disrupt the job market, particularly in larger companies, though this will likely become more important across mid-size businesses in the next 5 or 10 years as well (possibly edging out or giving machine learning consultancies a run for their money). A last, but definitely not minimal insight, in using ML for business: applying ML to cases where knowledge is not complete, in situations where discovering patterns or insights is too extensive and expensive a process in using just humans (as articulated by Dr. Claude Touzet of Aix-Marseille University in France).
In summary, businesses are most likely to be successful in implementing ML when they cover the triad of starting points: Choosing the right problem, having enough existing data to produce meaningful results, and having the right minds on board to help bring the process around full circle.
Related Emerj Interviews:
Image credit: Computer Business Review