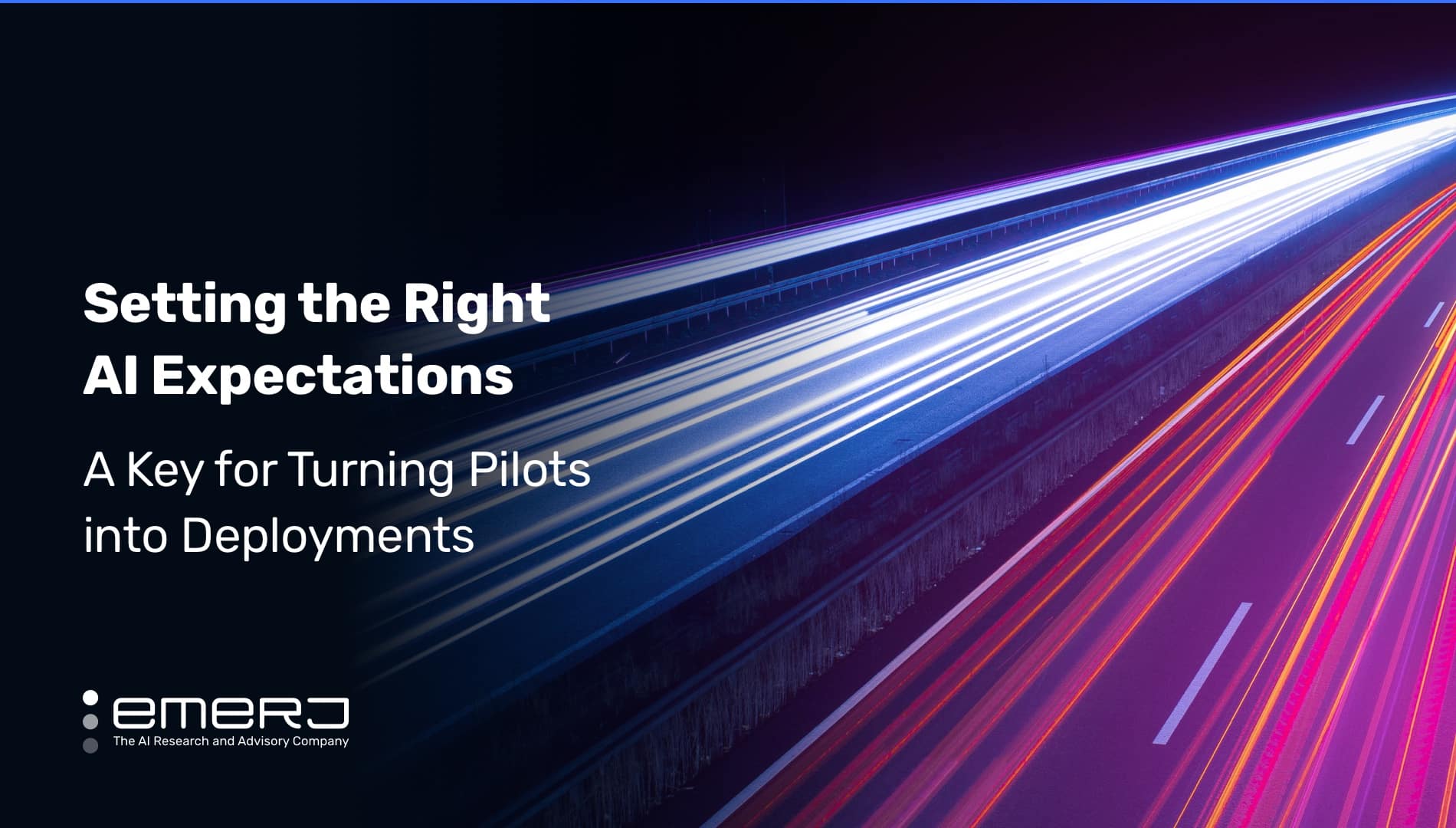
Setting expectations.
In our ongoing poll of new Emerj Plus members indicates that in addition to “accessing more AI use-cases” and “measuring AI ROI,” our members consistently tell us that they join in order to “set expectations for AI projects” – especially with leadership.
For professionals without direct experience managing AI projects, that objective may come as a surprise. For non-technical professionals with AI project experience, it will come as no surprise at all.
The wrong expectations about AI projects lead to some of the most pervasive issues we see in enterprise AI projects, including:
- The wrong AI projects (either not valuable, or unrealistic, or not connected to strategic/long-term goals) being select in the first place
- Projects being canceled or nixed because leadership didn’t understand the hurdles involved in achieving the desired outcome (this might include cleaning data, necessary iteration with different algorithmic approaches, etc)
On the other hand, setting the right expectations can help propel project success in important ways:
- Leadership vastly more willing to endure the ups and downs of iterative AI project approaches
- Leadership is able to see the value of building AI maturity, not simply to benefit from one plug-and-play solution
- AI projects get the runway, support, and budget they need to turn into real deployment – building momentum for the next batch of AI initiatives
We argue that executive AI fluency (i.e. leadership understanding how AI works practically and strategically) will be the lynchpin to unlocking the value of AI in the enterprise – but what can enterprise AI project leaders do when executive leadership has very little AI fluency?
That’s exactly what we’ll cover in this article.
The Wrong Expectations Come as the Default with Most Enterprise Leadership
The crux of almost all “wrong” expectation-setting in enterprise AI has to do with expectations around value. Simply put:
- Leadership is interested in (usually unrealistic) near-term financial ROI.
- Leadership sees little to no value in AI maturity and the strategic value of AI – and is often unwilling to even hear about these kinds of long-term value until some proxies of near-term value has been delivered.
AI is iterative, AI requires access to data, participation from SMEs, and many other unique factors for success – almost all of which make immediate, push-button financial ROI (in revenue or cost-savings) unrealistic.
So what do AI project leaders do?
- Naive or inexperienced AI project leaders imagine push-button results with AI, and they pass the pitch right from the vendors to the executives – selling leadership on bloated claims in order to win budgets and start projects.
- Savvy or experienced AI project leaders understand that immediate AI ROI is hard, but they also know that leadership wants to hear about financial ROI, not strategic or long-term value – and definitely not about building AI maturity. So, they let leadership stay ignorant, and they sell leadership on near-term value only, hoping to find a “quick win” (something you can never count on with AI) in order to open up bigger, strategic conversations about AI, and more ambitious projects.
The best vendors and the best internal AI project leaders do something different. They understand the balance between perceived and delivered value, which is what we’ll explore next:
Balancing Perceived and Deliverable Value
In the graphic above we highlight three possible scenarios:
- Scenario 1 is the most common. Here, AI project leaders sell leadership on AI ROI in order to “get to yes” for their budget and approval, but end up unable to show deliverable results that are anywhere near the perceived value that they conveyed upfront. As a consequence, project leaders look foolish, leadership is upset, and sometimes AI momentum slows for the entire company or department in substantial ways.
- Scenario 2 is rare, but not desirable. In this scenario, AI project leaders deliver AI value, but not in a way that matches the expectations (perceived value) in the mind of the executives who signed off on the budget to get the project started. Usually, this means that the project team helped to build AI maturity (improved data infrastructure, better AI talent, better AI project team alignment/best-practices), or bring a company closer to some important strategic goal (i.e. improving customer loyalty, becoming more data-driven, etc) – but leadership didn’t want that value, they wanted the short-term, plug-and-play AI that they perceived when they signed off on the project. The result – as with scenario 1, is a failure.
- Scenario 3 is rare and desirable. In this scenario, the AI project team set expectations of AI value, and then delivered on that same value to leadership. Leadership understood what they were getting into, and was happy with what they got.
Even with every best practice in place, and even with an excellent project team and savvy executives, there is absolutely no way to guarantee that AI projects will be a success. What we want to come close to guaranteeing, however, is:
- That leadership won’t be wildly surprised (and so wildly disappointed) by the challenges and setbacks our AI projects face
- That leadership will be much more likely to support a project long-term, and focus on delivering transformation, not just unrealistic short-term ROI
We’ve already covered how challenging it is for AI to deliver on guaranteed better-than-break-even financial ROI. So how can AI project leaders make good on deliverable results if their financial/measurable results
To do this, we need to actively leverage other types of return on investment beyond financial.
Leveraging Strategic and Capability ROI
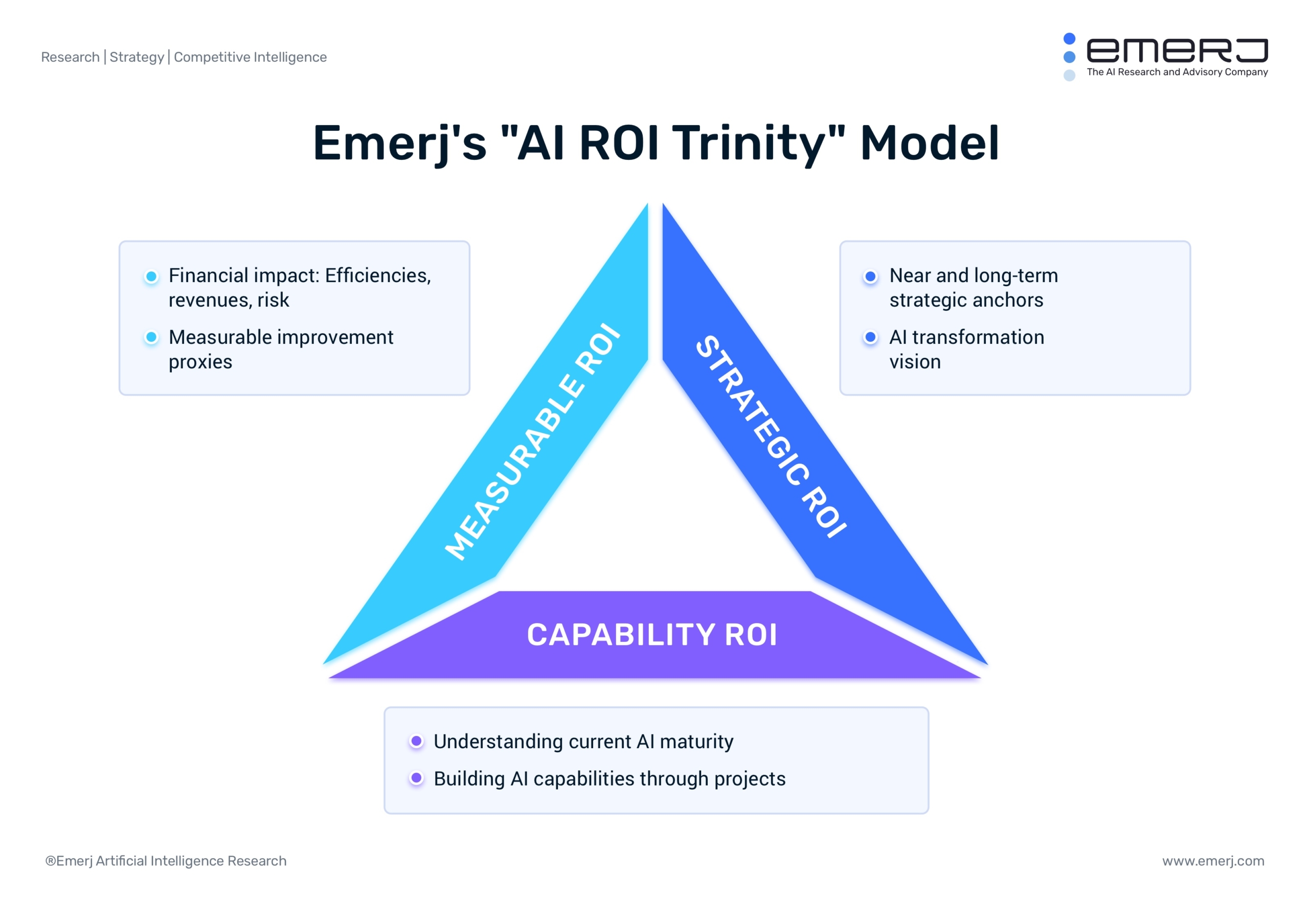
If AI project leaders use near-term (measurable, financial) ROI as the only type of value in their pitch (the perceived value for the executive buyer), they’re extremely unlikely to be able to show deliverable value that reaches those expectations.
The appropriate thing is not for project leaders to leave executive naive, while hoping for an unusual quick win from a first AI project. Rather, responsible AI project leaders present their business case with strategic and capability ROI as critical elements of what they’re asking leadership to invest in.
Strategic ROI is somewhat subjective. It implies getting closer to specific strategic goals or outcomes for the company. For example:
- An AI project that helps to unlock customer engagement and purchase data for an eCommerce company may also has strategic value if unlocking that data ties to the company’s strategic goals. If the firm has a long term strategic goal of improving loyalty and customer lifetime value for its niche, then an AI project that makes better use of that core customer data might be seen to have a strategic value.
Capability ROI is the value of the AI maturity developed in a given AI project. AI maturity is a composite of factors that allow for the fruitful use of AI – including skills, culture, and resources (including data and data infrastructure). AI projects that develop maturity allow a company to more easily leverage AI in the future. For example:
- A financial services firm with limited data science talent and almost no understanding of its fraud data might leverage AI to reduce payment fraud or detect money laundering. The project itself could be measured by a financial ROI, but the data scientists hired for the project and the increased understanding (and cleanliness) of fraud data would be what we call Capability ROI, and should be framed as such up front.
Setting AI expectations the right way implies being able to frame stategic and capability ROI upfront – even though executives are usually thinking purely in terms of financial ROI only. Having the tact and ability to frame strategic value and capability value upfront helps set those benefits as part of the perceived value that gets a decision-maker to say “yes.” This allows the AI project team to anchor on the strategic and capability benefits of the project even when some of the measurable aspects of ROI hit inevitable roadblocks. When perceived value matches deliverable value (what a leader sees at the end of the project), AI initiatives gain momentum and lead to real deployments.
Getting a decision-maker to “yes” with measurable ROI promises along is almost always a recipe for failure.
Sales Skills and Business Growth for AI Consultants and Service Providers
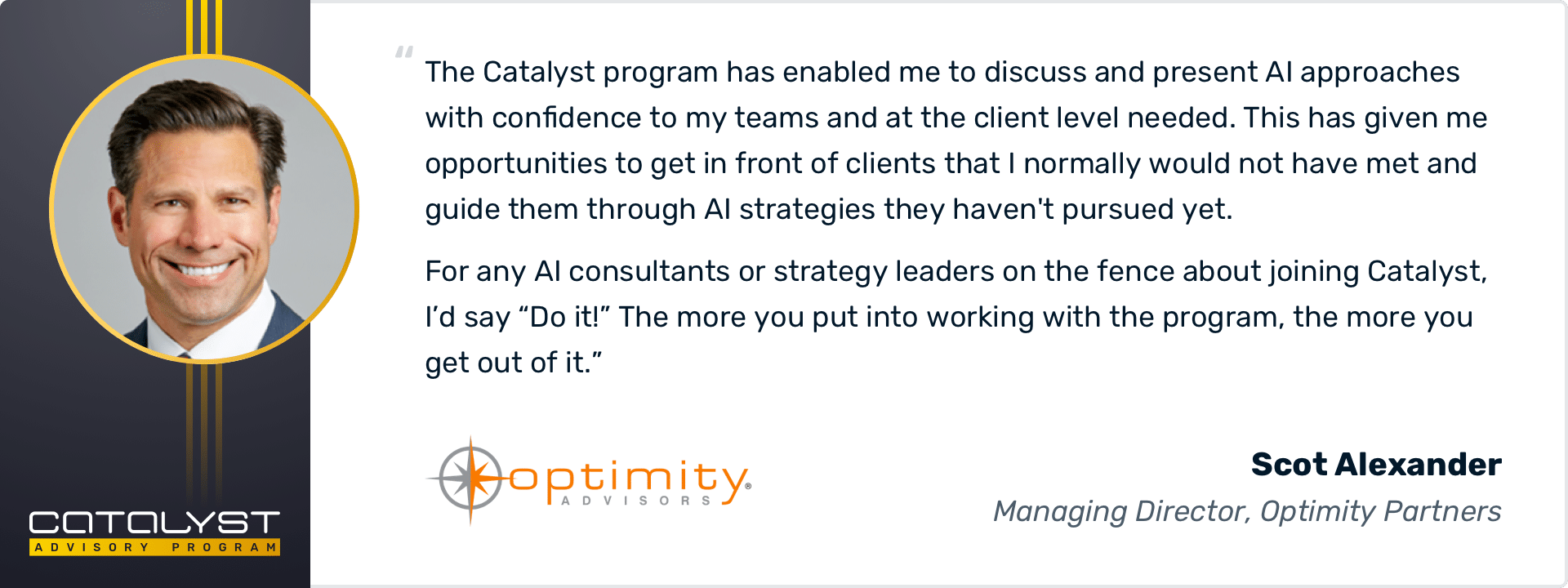
AI consultants, advisors, and vendors join our Catalyst Advisory Program to land more AI services business, and expand their client engagements to lucrative, long-term transformation relationships. Discover best-practices for finding and reaching potential customers and simple frameworks for framing a strong business case for your services, ultimately helping you win more deals and deliver more value to your clients. The Catalyst program involves 1-to-1 advisory, as well as peer-to-peer mentoring with other ambitious Catalyst members.
Take your AI services business to the next level – learn more on our Catalyst Advisory Program page.